Series
Introduction to Hyperspectral Remote Sensing Data in Python
In this series, we go over the basics of working with the NEON Level 3 NEON Spectrometer orthorectified surface directional reflectance - mosaic data (https://data.neonscience.org/data-products/DP3.30006.001) in Python.
This series covers how to:
- Open hyperspectral reflectance data stored in HDF5 format in Python
- Read in and explore the data and metadata contents
- Use functions in a Python module to efficiently visualize and interact with hyperspectral data cubes
- Extract and plot a single reflectance band and RGB and false-color band combinations
- Read in and plot the spectral signature of a single pixel
- Interactively plot the spectral signature of various pixels within a reflectance tile
Series Objectives
After completing the series you will:
-
Know how to extract data and metadata from HDF5 files in Python
-
Understand how to extract and plot spectra from an HDF5 file
-
Know how to work with groups and datasets within an HDF5 file in Python
-
Create RGB images from different band combinations in a hyperspectral data cube
-
Plot spectral signatures and understand how to remove the water vapor absorption "bad band" windows
-
Create basic vegetation indices, like NDVI, using raster-based calculations
Things You’ll Need To Complete This Series
Setup Python
To complete some of the tutorials in this series, you will need a recent version of Python
(3.9+) and, preferably, Jupyter Notebooks installed on your computer. You will also need to install the Python packages requests, gdal, and h5py.
Data
Data are downloaded programmatically in the lessons, but can also be downloaded from the NEON Data Portal. Code for downloading the data are provided in each lesson, but the same dataset is used in multiple lessons, so if you are following in sequence, you do not need to download the data each time.
NEON AOP Hyperspectral Data in HDF5 format with Python
Authors: Bridget Hass
Last Updated: Apr 17, 2024
In this introductory tutorial, we demonstrate how to read NEON AOP hyperspectral reflectance (Level 3, tiled) data in Python. This starts with the fundamental steps of reading in and exploring the HDF5 (h5) format that the reflectance data is delivered in. Then you will develop and practice skills to explore and visualize the spectral data.
Learning Objectives
After completing this tutorial, you will be able to:
- Use the package
h5py
and thevisititems
method to read a reflectance HDF5 file and view data attributes. - Read the data ignore value and scaling factor and apply these values to produce a "cleaned" reflectance array.
- Plot a histogram of reflectance values to visualize the range and distribution of values.
- Extract and plot a single band of reflectance data.
- Apply a histogram stretch and adaptive equalization to improve the contrast of an image.
Install Python Packages
If you don't already have these packages installed, you will need to do so, using pip
or conda
install:
- requests
- gdal
- h5py
Standard packages used in this tutorial include:
- numpy
- pandas
- matplotlib
Download Data
To complete this tutorial, you will download and read in surface directional reflectance data collected at the NEON Smithsonian Environmental Research Center (SERC) site in Maryland. This data can be downloaded by clicking the link below.
Download the SERC Directional Reflectance H5 Tile: NEON_D02_SERC_DP3_368000_4306000_reflectance.h5
Additional Resources
More details about the surface directional reflectance data product can be found on the data product page, linked below.
In addition, NEON'S Airborne Observation Platform provides Algorithm Theoretical Basis Documents (ATBDs) for all of their data products. Please refer to the ATBDs below for a more in-depth understanding ofthe reflectance datad.
Hyperspectral remote sensing data is a useful tool for measuring changes to our environment at the Earth’s surface. In this tutorial we explore how to extract information from a tile (1000m x 1000m x 426 bands) of NEON AOP orthorectified surface reflectance data, stored in hdf5 format. For more information on this data product, refer to the NEON Data Product Catalog.
Mapping the Invisible: Introduction to Spectral Remote Sensing
For more information on spectral remote sensing watch this video.
Set up
First let's import the required packages:
import os
import requests
import numpy as np
import h5py
from osgeo import gdal
import matplotlib.pyplot as plt
Read in the datasets
To start, we download the NEON surface directional reflectance data (DP3.30006.001), which is provided in hdf5 (.h5) format. You can download the file by clicking on the download link at the top of this lesson. Place the file inside a "data" folder in your working directory, and double check the file is located in the correct location, as follows:
# display the contents in the ./data folder to confirm the download completed
os.listdir('./data')
['NEON_D02_SERC_DP3_368000_4306000_reflectance.h5']
Read in hdf5 file
f = h5py.File('file.h5','r')
reads in an h5 file to the variable f.
Using the help
We will be using a number of built-in and user-defined functions and methods throughout the tutorial. If you are uncertain what a certain function does, or how to call it, you can type help()
or type a
?
at the end of the function or method and run the cell (either select Cell > Run Cells or Shift Enter with your cursor in the cell you want to run). The ?
will pop up a window at the bottom of the notebook displaying the function's docstrings
, which includes information about the function and usage. We encourage you to use help
and ?
throughout the tutorial as you come across functions you are unfamiliar with. Try out these commands:
help(h5py)
or
h5py.File?
Now that we have an idea of how to use h5py
to read in an h5 file, let's use this to explore the hyperspectral reflectance data. Note that if the h5 file is stored in a different directory than where you are running your notebook, you need to include the path (either relative or absolute) to the directory where that data file is stored. Use os.path.join
to create the full path of the file.
# Note that you may need to update this filepath for your local machine
f = h5py.File('./data/NEON_D02_SERC_DP3_368000_4306000_reflectance.h5','r')
Explore NEON AOP HDF5 Reflectance Files
We can look inside the HDF5 dataset with the h5py visititems
function. The list_dataset
function defined below displays all datasets stored in the hdf5 file and their locations within the hdf5 file:
#list_dataset lists the names of datasets in an hdf5 file
def list_dataset(name,node):
if isinstance(node, h5py.Dataset):
print(name)
f.visititems(list_dataset)
SERC/Reflectance/Metadata/Ancillary_Imagery/Aerosol_Optical_Depth
SERC/Reflectance/Metadata/Ancillary_Imagery/Aspect
SERC/Reflectance/Metadata/Ancillary_Imagery/Cast_Shadow
SERC/Reflectance/Metadata/Ancillary_Imagery/Dark_Dense_Vegetation_Classification
SERC/Reflectance/Metadata/Ancillary_Imagery/Data_Selection_Index
SERC/Reflectance/Metadata/Ancillary_Imagery/Haze_Cloud_Water_Map
SERC/Reflectance/Metadata/Ancillary_Imagery/Illumination_Factor
SERC/Reflectance/Metadata/Ancillary_Imagery/Path_Length
SERC/Reflectance/Metadata/Ancillary_Imagery/Sky_View_Factor
SERC/Reflectance/Metadata/Ancillary_Imagery/Slope
SERC/Reflectance/Metadata/Ancillary_Imagery/Smooth_Surface_Elevation
SERC/Reflectance/Metadata/Ancillary_Imagery/Visibility_Index_Map
SERC/Reflectance/Metadata/Ancillary_Imagery/Water_Vapor_Column
SERC/Reflectance/Metadata/Ancillary_Imagery/Weather_Quality_Indicator
SERC/Reflectance/Metadata/Coordinate_System/Coordinate_System_String
SERC/Reflectance/Metadata/Coordinate_System/EPSG Code
SERC/Reflectance/Metadata/Coordinate_System/Map_Info
SERC/Reflectance/Metadata/Coordinate_System/Proj4
SERC/Reflectance/Metadata/Logs/150649/ATCOR_Input_file
SERC/Reflectance/Metadata/Logs/150649/ATCOR_Processing_Log
SERC/Reflectance/Metadata/Logs/150649/Shadow_Processing_Log
SERC/Reflectance/Metadata/Logs/150649/Skyview_Processing_Log
SERC/Reflectance/Metadata/Logs/150649/Solar_Azimuth_Angle
SERC/Reflectance/Metadata/Logs/150649/Solar_Zenith_Angle
SERC/Reflectance/Metadata/Logs/151125/ATCOR_Input_file
SERC/Reflectance/Metadata/Logs/151125/ATCOR_Processing_Log
SERC/Reflectance/Metadata/Logs/151125/Shadow_Processing_Log
SERC/Reflectance/Metadata/Logs/151125/Skyview_Processing_Log
SERC/Reflectance/Metadata/Logs/151125/Solar_Azimuth_Angle
SERC/Reflectance/Metadata/Logs/151125/Solar_Zenith_Angle
SERC/Reflectance/Metadata/Logs/151614/ATCOR_Input_file
SERC/Reflectance/Metadata/Logs/151614/ATCOR_Processing_Log
SERC/Reflectance/Metadata/Logs/151614/Shadow_Processing_Log
SERC/Reflectance/Metadata/Logs/151614/Skyview_Processing_Log
SERC/Reflectance/Metadata/Logs/151614/Solar_Azimuth_Angle
SERC/Reflectance/Metadata/Logs/151614/Solar_Zenith_Angle
SERC/Reflectance/Metadata/Spectral_Data/FWHM
SERC/Reflectance/Metadata/Spectral_Data/Wavelength
SERC/Reflectance/Metadata/to-sensor_azimuth_angle
SERC/Reflectance/Metadata/to-sensor_zenith_angle
SERC/Reflectance/Reflectance_Data
You can see that there is a lot of information stored inside this reflectance hdf5 file. Most of this information is metadata (data about the reflectance data), for example, this file stores input parameters used in the atmospheric correction. For this introductory lesson, we will only work with two of these datasets, the reflectance data (hyperspectral cube), and the corresponding geospatial information, stored in Metadata/Coordinate_System:
-
SERC/Reflectance/Reflectance_Data
-
SERC/Reflectance/Metadata/Coordinate_System/
We can also display the name, shape, and type of each of these datasets using the ls_dataset
function defined below, which is also called with the visititems
method:
#ls_dataset displays the name, shape, and type of datasets in hdf5 file
def ls_dataset(name,node):
if isinstance(node, h5py.Dataset):
print(node)
Data Tip: To see what the visititems method (or any method) does, type ? at the end, eg.
f.visititems?
f.visititems(ls_dataset)
<HDF5 dataset "Aerosol_Optical_Depth": shape (1000, 1000), type "<i2">
<HDF5 dataset "Aspect": shape (1000, 1000), type "<f4">
<HDF5 dataset "Cast_Shadow": shape (1000, 1000), type "|u1">
<HDF5 dataset "Dark_Dense_Vegetation_Classification": shape (1000, 1000), type "|u1">
<HDF5 dataset "Data_Selection_Index": shape (1000, 1000), type "<i4">
<HDF5 dataset "Haze_Cloud_Water_Map": shape (1000, 1000), type "|u1">
<HDF5 dataset "Illumination_Factor": shape (1000, 1000), type "|u1">
<HDF5 dataset "Path_Length": shape (1000, 1000), type "<f4">
<HDF5 dataset "Sky_View_Factor": shape (1000, 1000), type "|u1">
<HDF5 dataset "Slope": shape (1000, 1000), type "<f4">
<HDF5 dataset "Smooth_Surface_Elevation": shape (1000, 1000), type "<f4">
<HDF5 dataset "Visibility_Index_Map": shape (1000, 1000), type "|u1">
<HDF5 dataset "Water_Vapor_Column": shape (1000, 1000), type "<f4">
<HDF5 dataset "Weather_Quality_Indicator": shape (1000, 1000, 3), type "|u1">
<HDF5 dataset "Coordinate_System_String": shape (), type "|O">
<HDF5 dataset "EPSG Code": shape (), type "|O">
<HDF5 dataset "Map_Info": shape (), type "|O">
<HDF5 dataset "Proj4": shape (), type "|O">
<HDF5 dataset "ATCOR_Input_file": shape (), type "|O">
<HDF5 dataset "ATCOR_Processing_Log": shape (), type "|O">
<HDF5 dataset "Shadow_Processing_Log": shape (), type "|O">
<HDF5 dataset "Skyview_Processing_Log": shape (), type "|O">
<HDF5 dataset "Solar_Azimuth_Angle": shape (), type "<f4">
<HDF5 dataset "Solar_Zenith_Angle": shape (), type "<f4">
<HDF5 dataset "ATCOR_Input_file": shape (), type "|O">
<HDF5 dataset "ATCOR_Processing_Log": shape (), type "|O">
<HDF5 dataset "Shadow_Processing_Log": shape (), type "|O">
<HDF5 dataset "Skyview_Processing_Log": shape (), type "|O">
<HDF5 dataset "Solar_Azimuth_Angle": shape (), type "<f4">
<HDF5 dataset "Solar_Zenith_Angle": shape (), type "<f4">
<HDF5 dataset "ATCOR_Input_file": shape (), type "|O">
<HDF5 dataset "ATCOR_Processing_Log": shape (), type "|O">
<HDF5 dataset "Shadow_Processing_Log": shape (), type "|O">
<HDF5 dataset "Skyview_Processing_Log": shape (), type "|O">
<HDF5 dataset "Solar_Azimuth_Angle": shape (), type "<f4">
<HDF5 dataset "Solar_Zenith_Angle": shape (), type "<f4">
<HDF5 dataset "FWHM": shape (426,), type "<f4">
<HDF5 dataset "Wavelength": shape (426,), type "<f4">
<HDF5 dataset "to-sensor_azimuth_angle": shape (1000, 1000), type "<f4">
<HDF5 dataset "to-sensor_zenith_angle": shape (1000, 1000), type "<f4">
<HDF5 dataset "Reflectance_Data": shape (1000, 1000, 426), type "<i2">
Now that we can see the structure of the hdf5 file, let's take a look at some of the information that is stored inside. Let's start by extracting the reflectance data, which is nested under SERC/Reflectance/Reflectance_Data
:
serc_refl = f['SERC']['Reflectance']
print(serc_refl)
<HDF5 group "/SERC/Reflectance" (2 members)>
The two members of the HDF5 group /SERC/Reflectance
are Metadata
and Reflectance_Data
. Let's save the reflectance data as the variable serc_reflArray:
serc_reflArray = serc_refl['Reflectance_Data']
print(serc_reflArray)
<HDF5 dataset "Reflectance_Data": shape (1000, 1000, 426), type "<i2">
We can extract the size of this reflectance array that we extracted using the shape
method:
refl_shape = serc_reflArray.shape
print('SERC Reflectance Data Dimensions:',refl_shape)
SERC Reflectance Data Dimensions: (1000, 1000, 426)
This 3-D shape (1000,1000,426) corresponds to (y,x,bands), where (x,y) are the dimensions of the reflectance array in pixels. Hyperspectral data sets are often called "cubes" to reflect this 3-dimensional shape.
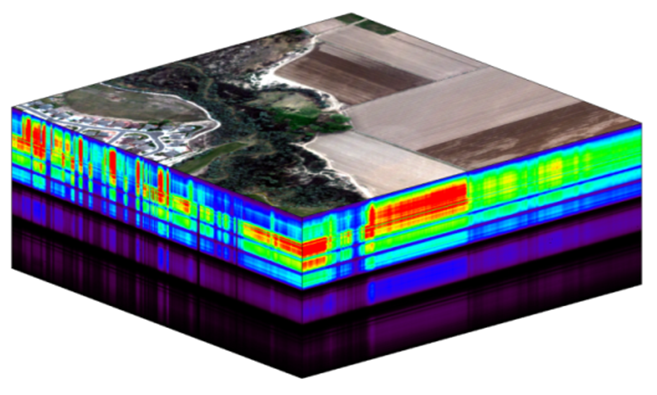
NEON hyperspectral data contain around 426 spectral bands, and when working with tiled data, the spatial dimensions are 1000 x 1000, where each pixel represents 1 meter. Now let's take a look at the wavelength values. First, we will extract wavelength information from the serc_refl
variable that we created:
#define the wavelengths variable
wavelengths = serc_refl['Metadata']['Spectral_Data']['Wavelength']
#View wavelength information and values
print('wavelengths:',wavelengths)
wavelengths: <HDF5 dataset "Wavelength": shape (426,), type "<f4">
We can then use numpy
(imported as np
) to see the minimum and maximum wavelength values:
# Display min & max wavelengths
print('min wavelength:', np.amin(wavelengths),'nm')
print('max wavelength:', np.amax(wavelengths),'nm')
min wavelength: 383.884 nm
max wavelength: 2512.1804 nm
Finally, we can determine the band widths (distance between center bands of two adjacent bands). Let's try this for the first two bands and the last two bands. Remember that Python uses 0-based indexing ([0]
represents the first value in an array), and note that you can also use negative numbers to splice values from the end of an array ([-1]
represents the last value in an array).
#show the band widths between the first 2 bands and last 2 bands
print('band width between first 2 bands =',(wavelengths[1]-wavelengths[0]),'nm')
print('band width between last 2 bands =',(wavelengths[-1]-wavelengths[-2]),'nm')
band width between first 2 bands = 5.0076904 nm
band width between last 2 bands = 5.0078125 nm
The center wavelengths recorded in this hyperspectral cube range from 383.88 - 2512.18 nm, and each band covers a range of ~5 nm. Now let's extract spatial information, which is stored under SERC/Reflectance/Metadata/Coordinate_System/Map_Info
:
serc_mapInfo = serc_refl['Metadata']['Coordinate_System']['Map_Info']
print('SERC Map Info:',serc_mapInfo)
SERC Map Info: <HDF5 dataset "Map_Info": shape (), type "|O">
serc_mapInfo[()]
b'UTM, 1.000, 1.000, 368000.00, 4307000.0, 1.0000000, 1.0000000, 18, North, WGS-84, units=Meters, 0'
Understanding the output:
Here we can spatial information about the reflectance data. Below is a break down of what each of these values means:
-
UTM
- coordinate system (Universal Transverse Mercator) -
1.000, 1.000
- -
368000.000, 4307000.0
- UTM coordinates (meters) of the map origin, which refers to the upper-left corner of the image (xMin, yMax). -
1.0000000, 1.0000000
- pixel resolution (meters) -
18
- UTM zone -
N
- UTM hemisphere (North for all NEON sites) -
WGS-84
- reference ellipoid
Note that the letter b
that appears before UTM signifies that the variable-length string data is stored in binary format when it is written to the hdf5 file. Don't worry about it for now, as we will convert the numerical data we need into floating point numbers. For more information on hdf5 strings read the h5py documentation.
You can display this in as a string as follows:
serc_mapInfo[()].decode("utf-8")
Let's extract relevant information from the Map_Info
metadata to define the spatial extent of this dataset. To do this, we can use the split
method to break up this string into separate values:
#First convert mapInfo to a string
mapInfo_string = serc_mapInfo[()].decode("utf-8") # read in as string
#split the strings using the separator ","
mapInfo_split = mapInfo_string.split(",")
print(mapInfo_split)
['UTM', ' 1.000', ' 1.000', ' 368000.00', ' 4307000.0', ' 1.0000000', ' 1.0000000', ' 18', ' North', ' WGS-84', ' units=Meters', ' 0']
Now we can extract the spatial information we need from the map info values, convert them to the appropriate data type (float) and store it in a way that will enable us to access and apply it later when we want to plot the data:
#Extract the resolution & convert to floating decimal number
res = float(mapInfo_split[5]),float(mapInfo_split[6])
print('Resolution:',res)
Resolution: (1.0, 1.0)
#Extract the upper left-hand corner coordinates from mapInfo
xMin = float(mapInfo_split[3])
yMax = float(mapInfo_split[4])
#Calculate the xMax and yMin values from the dimensions
xMax = xMin + (refl_shape[1]*res[0]) #xMax = left edge + (# of columns * x pixel resolution)
yMin = yMax - (refl_shape[0]*res[1]) #yMin = top edge - (# of rows * y pixel resolution)
Now we can define the spatial extent as the tuple (xMin, xMax, yMin, yMax)
. This is the format required for applying the spatial extent when plotting with matplotlib.pyplot
.
#Define extent as a tuple:
serc_ext = (xMin, xMax, yMin, yMax)
print('serc_ext:',serc_ext)
print('serc_ext type:',type(serc_ext))
serc_ext: (368000.0, 369000.0, 4306000.0, 4307000.0)
serc_ext type: <class 'tuple'>
Extract a Single Band from Array
While it is useful to have all the data contained in a hyperspectral cube, it is difficult to visualize all this information at once. We can extract a single band (representing a ~5nm band, approximating a single wavelength) from the cube by using splicing as follows. Note that we have to cast the reflectance data into the type float
. Recall that since Python indexing starts at 0 instead of 1, in order to extract band 56, we need to use the index 55.
b56 = serc_reflArray[:,:,55].astype(float)
print('b56 type:',type(b56))
print('b56 shape:',b56.shape)
print('Band 56 Reflectance:\n',b56)
b56 type: <class 'numpy.ndarray'>
b56 shape: (1000, 1000)
Band 56 Reflectance:
[[1045. 954. 926. ... 399. 386. 461.]
[ 877. 877. 993. ... 341. 379. 428.]
[ 768. 1849. 1932. ... 369. 380. 384.]
...
[ 337. 254. 252. ... 421. 971. 1191.]
[ 340. 341. 329. ... 708. 1102. 1449.]
[ 334. 345. 341. ... 685. 862. 1382.]]
Here we can see that we extracted a 2-D array (1000 x 1000) of the scaled reflectance data corresponding to the wavelength band 56. Before we can use the data, we need to clean it up a little. We'll show how to do this below.
Scale factor and No Data Value
This array represents the scaled reflectance for band 56. Recall from exploring the HDF5 data in HDFViewer that NEON AOP reflectance data uses a Data_Ignore_Value
of -9999
to represent missing data (often called NaN
), and a reflectance Scale_Factor
of 10000.0
in order to save disk space (can use lower precision this way).
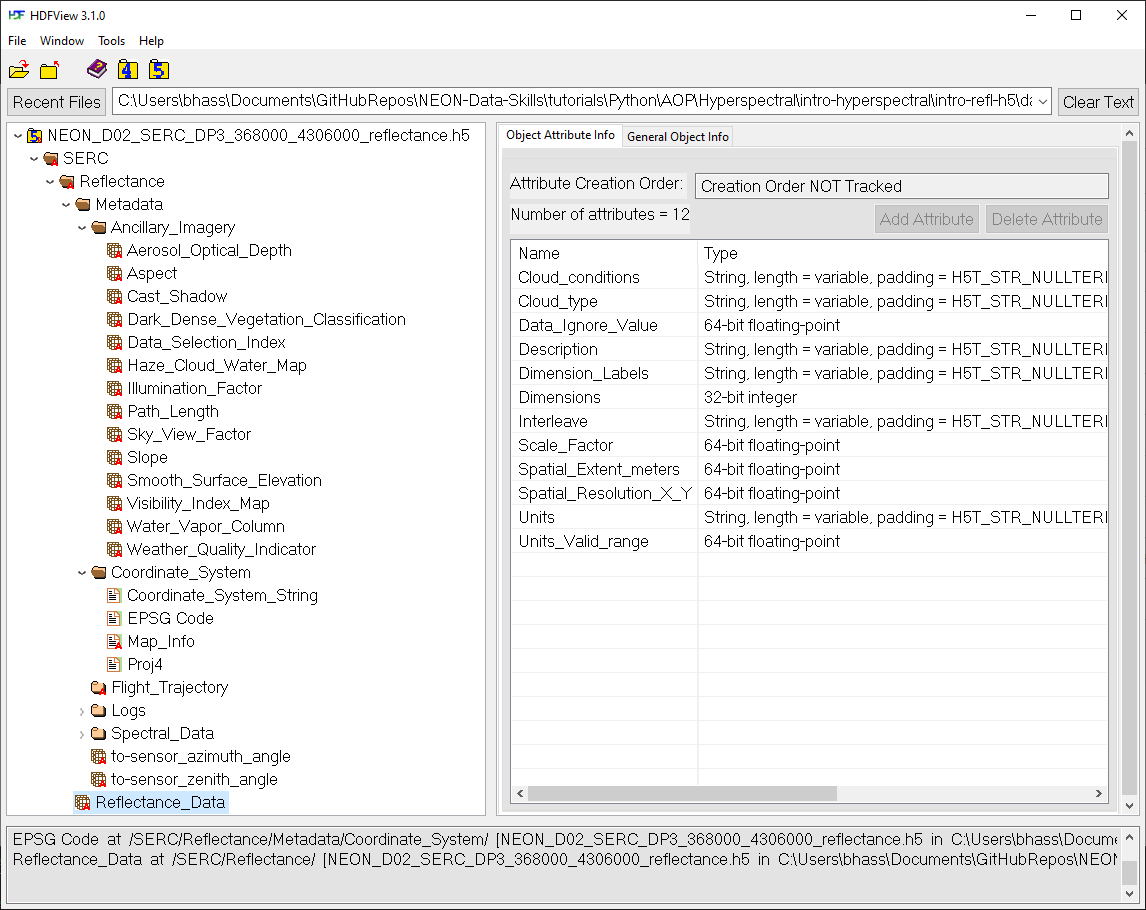
We can extract and apply the Data_Ignore_Value
and Scale_Factor
as follows:
#View and apply scale factor and data ignore value
scaleFactor = serc_reflArray.attrs['Scale_Factor']
noDataValue = serc_reflArray.attrs['Data_Ignore_Value']
print('Scale Factor:',scaleFactor)
print('Data Ignore Value:',noDataValue)
b56[b56==int(noDataValue)]=np.nan
b56 = b56/scaleFactor
print('Cleaned Band 56 Reflectance:\n',b56)
Scale Factor: 10000.0
Data Ignore Value: -9999.0
Cleaned Band 56 Reflectance:
[[0.1045 0.0954 0.0926 ... 0.0399 0.0386 0.0461]
[0.0877 0.0877 0.0993 ... 0.0341 0.0379 0.0428]
[0.0768 0.1849 0.1932 ... 0.0369 0.038 0.0384]
...
[0.0337 0.0254 0.0252 ... 0.0421 0.0971 0.1191]
[0.034 0.0341 0.0329 ... 0.0708 0.1102 0.1449]
[0.0334 0.0345 0.0341 ... 0.0685 0.0862 0.1382]]
Plot single reflectance band
Now we can plot this band using the Python package matplotlib.pyplot
, which we imported at the beginning of the lesson as plt
. Note that the default colormap is jet unless otherwise specified. You can explore using different colormaps on your own; see the mapplotlib colormaps for for other options.
serc_plot = plt.imshow(b56,extent=serc_ext,cmap='Greys')
We can see that this image looks pretty washed out. To see why this is, it helps to look at the range and distribution of reflectance values that we are plotting. We can do this by making a histogram.
Plot histogram
We can plot a histogram using the matplotlib.pyplot.hist
function. Note that this function won't work if there are any NaN values, so we can ensure we are only plotting the real data values using the call below. You can also specify the # of bins you want to divide the data into.
plt.hist(b56[~np.isnan(b56)],50); #50 signifies the # of bins
We can see that most of the reflectance values are < 0.4. In order to show more contrast in the image, we can adjust the colorlimit (clim
) to 0-0.4:
serc_plot = plt.imshow(b56,extent=serc_ext,cmap='Greys',clim=(0,0.4))
plt.title('SERC Band 56 Reflectance');
Here you can see that adjusting the colorlimit displays features (eg. roads, buildings) much better than when we set the colormap limits to the entire range of reflectance values.
Optional Exercise: Image Processing -- Contrast Stretch & Histogram Equalization
We can also try out some basic image processing to better visualize the reflectance data using the scikit-image
package.
Histogram equalization is a method in image processing of contrast adjustment using the image's histogram. Stretching the histogram can improve the contrast of a displayed image, as we will show how to do below.
The following code is adapted from scikit-image's tutorial Histogram Equalization.
Below we demonstrate a widget to interactively explore different linear contrast stretches:
Explore the contrast stretch feature interactively using IPython widgets:
from skimage import exposure
from IPython.html.widgets import *
def linearStretch(percent):
pLow, pHigh = np.percentile(b56[~np.isnan(b56)], (percent,100-percent))
img_rescale = exposure.rescale_intensity(b56, in_range=(pLow,pHigh))
plt.imshow(img_rescale,extent=serc_ext,cmap='gist_earth')
#cbar = plt.colorbar(); cbar.set_label('Reflectance')
plt.title('SERC Band 56 \n Linear ' + str(percent) + '% Contrast Stretch');
ax = plt.gca()
ax.ticklabel_format(useOffset=False, style='plain') #do not use scientific notation #
rotatexlabels = plt.setp(ax.get_xticklabels(),rotation=90) #rotate x tick labels 90 degree
interact(linearStretch,percent=(0,50,1));
interactive(children=(IntSlider(value=25, description='percent', max=50), Output()), _dom_classes=('widget-int…
Get Lesson Code
Read in and visualize hyperspectral data in Python
Authors: Bridget Hass
Last Updated: Apr 15, 2024
In this tutorial, you will learn how to efficiently read in hyperspectral surface directional reflectance hdf5 data and metadata, plot a single band and Red-Green-Blue (RGB) band combinations of a reflectance data tile using Python functions created for working with and visualizing NEON AOP hyperspectral data.
This tutorial works with the Level 3 Spectrometer orthorectified surface directional reflectance - mosaic data product.
Learning Objectives
After completing this tutorial, you will be able to:
- Work with Python modules and functions
- Read in tiled NEON AOP reflectance hdf5 data and associated metadata
- Plot a single band of reflectance data
- Stack and plot 3-band combinations to visualize true color and false color images
Install Python Packages
- h5py
- gdal
- requests
Data
Data and additional scripts required for this lesson are downloaded programmatically as part of the tutorial.
The data used in this tutorial were collected over NEON's Disney Wilderness Preserve (DSNY) field site and processed at NEON headquarters.
The dataset can also be downloaded from the NEON Data Portal.
We can combine any three bands from the NEON reflectance data to make an RGB image that will depict different information about the Earth's surface. A natural color image, made with bands from the red, green, and blue wavelengths looks close to what we would see with the naked eye. We can also choose band combinations from other wavelenghts, and map them to the red, blue, and green colors to highlight different features. A false color image is made with one or more bands from a non-visible portion of the electromagnetic spectrum that are mapped to red, green, and blue colors. These images can display other information about the landscape that is not easily seen with a natural color image.
The NASA Goddard Media Studio video "Peeling Back Landsat's Layers of Data" gives a good quick overview of natural and false color band combinations. Note that the Landsat multispectral sensor collects information from 11 bands, while NEON AOP hyperspectral data captures information spanning 426 bands!
Peeling Back Landsat's Layers of Data Video
Further Reading
- Check out the NASA Earth Observatory article How to Interpret a False-Color Satellite Image.
- Read the supporting article for the video above, Landsat 8 Onion Skin.
Load Function Module
First we can import the required packages and the neon_aop_hyperspectral
module, which includes a number of functions which we will use to read in the hyperspectral hdf5 data as well as visualize the data.
import os
import sys
import time
import h5py
import requests
import numpy as np
import matplotlib.pyplot as plt
This next function is a handy way to download the Python module and data that we will be using for this lesson. This uses the requests
package.
# function to download data stored on the internet in a public url to a local file
def download_url(url,download_dir):
if not os.path.isdir(download_dir):
os.makedirs(download_dir)
filename = url.split('/')[-1]
r = requests.get(url, allow_redirects=True)
file_object = open(os.path.join(download_dir,filename),'wb')
file_object.write(r.content)
Download the module from its location on GitHub, add the python_modules to the path and import the neon_aop_hyperspectral.py module.
module_url = "https://raw.githubusercontent.com/NEONScience/NEON-Data-Skills/main/tutorials/Python/AOP/aop_python_modules/neon_aop_hyperspectral.py"
download_url(module_url,'../python_modules')
# os.listdir('../python_modules') #optionally show the contents of this directory to confirm the file downloaded
sys.path.insert(0, '../python_modules')
# import the neon_aop_hyperspectral module, the semicolon supresses an empty plot from displaying
import neon_aop_hyperspectral as neon_hs;
The first function we will use is aop_h5refl2array
. We encourage you to look through the code to understand what it is doing behind the scenes. This function automates the steps required to read AOP hdf5 reflectance files into a Python numpy array. This function also cleans the data: it sets any no data values within the reflectance tile to nan
(not a number) and applies the reflectance scale factor so the final array that is returned represents unitless scaled reflectance, with values ranging between 0 and 1 (0-100%).
If you forget what this function does, or don't want to scroll up to read the docstrings, remember you can use help
or ?
to display the associated docstrings.
help(neon_hs.aop_h5refl2array)
# neon_hs.aop_h5refl2array? #uncomment for an alternate way to show the help
Help on function aop_h5refl2array in module neon_aop_hyperspectral:
aop_h5refl2array(h5_filename, raster_type_: Literal['Cast_Shadow', 'Data_Selection_Index', 'GLT_Data', 'Haze_Cloud_Water_Map', 'IGM_Data', 'Illumination_Factor', 'OBS_Data', 'Radiance', 'Reflectance', 'Sky_View_Factor', 'to-sensor_Azimuth_Angle', 'to-sensor_Zenith_Angle', 'Visibility_Index_Map', 'Weather_Quality_Indicator'], only_metadata=False)
read in NEON AOP reflectance hdf5 file and return the un-scaled
reflectance array, associated metadata, and wavelengths
Parameters
----------
h5_filename : string
reflectance hdf5 file name, including full or relative path
raster : string
name of raster value to read in; this will typically be the reflectance data,
but other data stored in the h5 file can be accessed as well
valid options:
Cast_Shadow (ATCOR input)
Data_Selection_Index
GLT_Data
Haze_Cloud_Water_Map (ATCOR output)
IGM_Data
Illumination_Factor (ATCOR input)
OBS_Data
Reflectance
Radiance
Sky_View_Factor (ATCOR input)
to-sensor_Azimuth_Angle
to-sensor_Zenith_Angle
Visibility_Index_Map: sea level values of visibility index / total optical thickeness
Weather_Quality_Indicator: estimated percentage of overhead cloud cover during acquisition
Returns
--------
raster_array : ndarray
array of reflectance values
metadata: dictionary
associated metadata containing
bad_band_window1 (tuple)
bad_band_window2 (tuple)
bands: # of bands (float)
data ignore value: value corresponding to no data (float)
epsg: coordinate system code (float)
map info: coordinate system, datum & ellipsoid, pixel dimensions, and origin coordinates (string)
reflectance scale factor: factor by which reflectance is scaled (float)
wavelengths: array
wavelength values, in nm
--------
Example Execution:
--------
refl, refl_metadata = aop_h5refl2array('NEON_D02_SERC_DP3_368000_4306000_reflectance.h5','Reflectance')
Now that we have an idea of how this function works, let's try it out. First, let's download a file. For this tutorial, we will use requests to download from the public link where the data is stored on the cloud (Google Cloud Storage). This downloads to a data folder in the working directory, but you can download it to a different location if you prefer.
# define the data_url to point to the cloud storage location of the the hyperspectral hdf5 data file
data_url = "https://storage.googleapis.com/neon-aop-products/2021/FullSite/D03/2021_DSNY_6/L3/Spectrometer/Reflectance/NEON_D03_DSNY_DP3_454000_3113000_reflectance.h5"
# download the h5 data and display how much time it took to download (uncomment 1st and 3rd lines)
# start_time = time.time()
download_url(data_url,'.\data')
# print("--- It took %s seconds to download the data ---" % round((time.time() - start_time),1))
# display the contents in the ./data folder to confirm the download completed
os.listdir('./data')
['NEON_D03_DSNY_DP3_454000_3113000_reflectance.h5']
# read the h5 reflectance file (including the full path) to the variable h5_file_name
h5_file_name = data_url.split('/')[-1]
h5_tile = os.path.join(".\data",h5_file_name)
print(f'h5_tile: {h5_tile}')
h5_tile: .\data\NEON_D03_DSNY_DP3_454000_3113000_reflectance.h5
Now that we've specified our reflectance tile, we can call aop_h5refl2array
to read in the reflectance tile as a python array called refl
, the metadata into a dictionary called refl_metadata
, and the wavelengths into an array.
# read in the reflectance data using the aop_h5refl2array function, this may also take a bit of time
start_time = time.time()
refl, refl_metadata, wavelengths = neon_hs.aop_h5refl2array(h5_tile,'Reflectance')
print("--- It took %s seconds to read in the data ---" % round((time.time() - start_time),0))
Reading in .\data\NEON_D03_DSNY_DP3_454000_3113000_reflectance.h5
--- It took 7.0 seconds to read in the data ---
# display the reflectance metadata dictionary contents
refl_metadata
{'shape': (1000, 1000, 426),
'no_data_value': -9999.0,
'scale_factor': 10000.0,
'bad_band_window1': array([1340, 1445]),
'bad_band_window2': array([1790, 1955]),
'projection': b'+proj=UTM +zone=17 +ellps=WGS84 +datum=WGS84 +units=m +no_defs',
'EPSG': 32617,
'res': {'pixelWidth': 1.0, 'pixelHeight': 1.0},
'extent': (454000.0, 455000.0, 3113000.0, 3114000.0),
'ext_dict': {'xMin': 454000.0,
'xMax': 455000.0,
'yMin': 3113000.0,
'yMax': 3114000.0},
'source': '.\\data\\NEON_D03_DSNY_DP3_454000_3113000_reflectance.h5'}
# display the first 5 values of the wavelengths
wavelengths[:5]
array([383.884 , 388.8917, 393.8995, 398.9072, 403.915 ], dtype=float32)
We can use the shape
method to see the dimensions of the array we read in. Use this method to confirm that the size of the reflectance array makes sense given the hyperspectral data cube, which is 1000 meters x 1000 meters x 426 bands.
refl.shape
(1000, 1000, 426)
plot_aop_refl
: plot a single band of the reflectance data
Next we'll use the function plot_aop_refl
to plot a single band of reflectance data. You can use help
to understand the required inputs and data types for each of these; only the band and spatial extent are required inputs, the rest are optional inputs. If specified, these optional inputs allow you to set the range color values, specify the axis, add a title, colorbar, colorbar title, and change the colormap (default is to plot in greyscale).
band56 = refl[:,:,55]
neon_hs.plot_aop_refl(band56/refl_metadata['scale_factor'],
refl_metadata['extent'],
colorlimit=(0,0.3),
title='DSNY Tile Band 56',
cmap_title='Reflectance',
colormap='gist_earth')
RGB Plots - Band Stacking
It is often useful to look at several bands together. We can extract and stack three reflectance bands in the red, green, and blue (RGB) spectrums to produce a color image that looks like what we see with our eyes; this is your typical camera image. In the next part of this tutorial, we will learn to stack multiple bands and make a geotif raster from the compilation of these bands. We can see that different combinations of bands allow for different visualizations of the remotely-sensed objects and also conveys useful information about the chemical makeup of the Earth's surface.
We will select bands that fall within the visible range of the electromagnetic spectrum (400-700 nm) and at specific points that correspond to what we see as red, green, and blue.

For this exercise, we'll first use the function stack_rgb
to extract the bands we want to stack. This function uses splicing to extract the nth band from the reflectance array, and then uses the numpy function stack
to create a new 3D array (1000 x 1000 x 3) consisting of only the three bands we want.
# pull out the true-color band combinations
rgb_bands = (58,34,19) # set the red, green, and blue bands
# stack the 3-band combinations (rgb and cir) using stack_rgb function
rgb_unscaled = neon_hs.stack_rgb(refl,rgb_bands)
# apply the reflectance scale factor
rgb = rgb_unscaled/refl_metadata['scale_factor']
We can display the red, green, and blue band center wavelengths, whose indices were defined above. To confirm that these band indices correspond to wavelengths in the expected portion of the spectrum, we can print out the wavelength values in nanometers.
print('Center wavelengths:')
print('Band 58: %.1f' %(wavelengths[57]),'nm')
print('Band 33: %.1f' %(wavelengths[33]),'nm')
print('Band 19: %.1f' %(wavelengths[18]),'nm')
Center wavelengths:
Band 58: 669.3 nm
Band 33: 549.1 nm
Band 19: 474.0 nm
plot_aop_rgb: plot an RGB band combination
Next, we can use the function plot_aop_rgb
to plot the band stack as follows:
# plot the true color image (rgb)
neon_hs.plot_aop_rgb(rgb,
refl_metadata['extent'],
plot_title='DSNY Reflectance RGB Image')
False Color Image - Color Infrared (CIR)
We can also create an image from bands outside of the visible spectrum. An image containing one or more bands outside of the visible range is called a false-color image. Here we'll use the green and blue bands as before, but we replace the red band with a near-infrared (NIR) band.
For more information about non-visible wavelengths, false color images, and some frequently used false-color band combinations, refer to NASA's Earth Observatory page.
cir_bands = (90,34,19)
print('Band 90 Center Wavelength = %.1f' %(wavelengths[89]),'nm')
print('Band 34 Center Wavelength = %.1f' %(wavelengths[33]),'nm')
print('Band 19 Center Wavelength = %.1f' %(wavelengths[18]),'nm')
cir = neon_hs.stack_rgb(refl,cir_bands)
neon_hs.plot_aop_rgb(cir,
refl_metadata['extent'],
ls_pct=20,
plot_title='DSNY Color Infrared Image')
Clipping input data to the valid range for imshow with RGB data ([0..1] for floats or [0..255] for integers).
Band 90 Center Wavelength = 829.6 nm
Band 34 Center Wavelength = 549.1 nm
Band 19 Center Wavelength = 474.0 nm
References
Kekesi, Alex et al. "NASA | Peeling Back Landsat's Layers of Data". https://svs.gsfc.nasa.gov/vis/a010000/a011400/a011491/. Published on Feb 24, 2014.
Riebeek, Holli. "Why is that Forest Red and that Cloud Blue? How to Interpret a False-Color Satellite Image" https://earthobservatory.nasa.gov/Features/FalseColor/
Get Lesson Code
Plot a Spectral Signature from Reflectance Data in Python
Authors: Bridget Hass
Last Updated: Apr 15, 2024
In this tutorial, we will learn how to extract and plot a spectral profile from a single pixel of a reflectance band in a NEON hyperspectral HDF5 file.
This tutorial works with NEON's Level 3 Spectrometer orthorectified surface directional reflectance - mosaic data product.
Objectives
After completing this tutorial, you will be able to:
- Plot the spectral signature of a single pixel
- Remove bad band windows from a spectra
- Use a IPython
widget
to interactively view spectra of various pixels
Install Python Packages
- gdal
- h5py
- requests
- IPython
Data
Data and additional scripts required for this lesson are downloaded programmatically as part of the tutorial.
The hyperspectral imagery file used in this lesson was collected over NEON's Smithsonian Environmental Research Center field site in 2021 and processed at NEON headquarters.
The entire dataset can be accessed on the NEON Data Portal.
In this exercise, we will learn how to extract and plot a spectral profile from a single pixel of a reflectance band in a NEON hyperspectral hdf5 file. To do this, we will use the aop_h5refl2array
function to read in and clean our h5 reflectance data, and the Python package pandas
to create a dataframe for the reflectance and associated wavelength data.
Spectral Signatures
A spectral signature is a plot of the amount of light energy reflected by an object throughout the range of wavelengths in the electromagnetic spectrum. The spectral signature of an object conveys useful information about its structural and chemical composition. We can use these signatures to identify and classify different objects from a spectral image.
For example, vegetation has a distinct spectral signature.
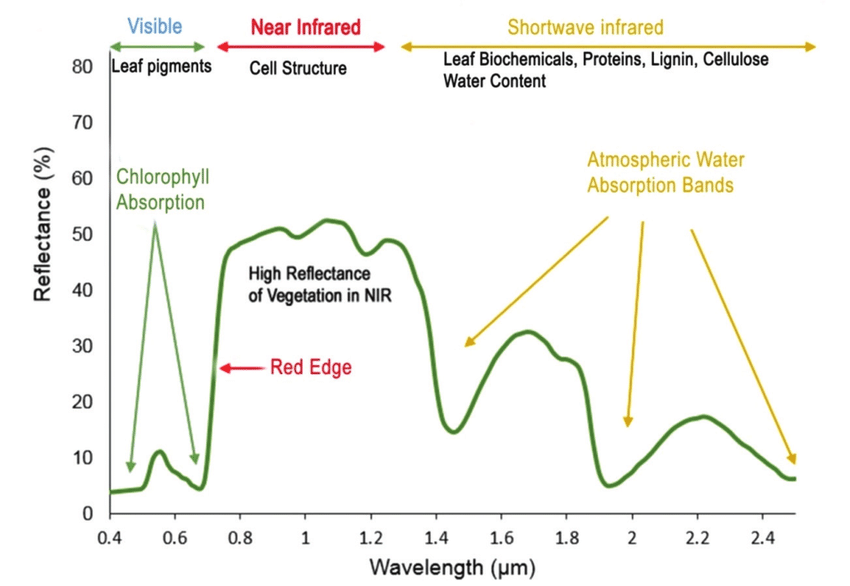
Vegetation has a unique spectral signature characterized by high reflectance in the near infrared wavelengths, and much lower reflectance in the green portion of the visible spectrum. For more details, please see Vegetation Analysis: Using Vegetation Indices in ENVI . We can extract reflectance values in the NIR and visible spectrums from hyperspectral data in order to map vegetation on the earth's surface. You can also use spectral curves as a proxy for vegetation health. We will explore this concept more in the next lesson, where we will caluclate vegetation indices.

import os, sys
import requests
import numpy as np
import pandas as pd
import matplotlib.pyplot as plt
This next function is a handy way to download the Python module and data that we will be using for this lesson. This uses the requests
package.
# function to download data stored on the internet in a public url to a local file
def download_url(url,download_dir):
if not os.path.isdir(download_dir):
os.makedirs(download_dir)
filename = url.split('/')[-1]
r = requests.get(url, allow_redirects=True)
file_object = open(os.path.join(download_dir,filename),'wb')
file_object.write(r.content)
Download the module from its location on GitHub, add the python_modules to the path and import the neon_aop_hyperspectral.py module.
module_url = "https://raw.githubusercontent.com/NEONScience/NEON-Data-Skills/main/tutorials/Python/AOP/aop_python_modules/neon_aop_hyperspectral.py"
download_url(module_url,'../python_modules')
# os.listdir('../python_modules') #optionally show the contents of this directory to confirm the file downloaded
sys.path.insert(0, '../python_modules')
# import the neon_aop_hyperspectral module, the semicolon supresses an empty plot from displaying
import neon_aop_hyperspectral as neon_hs;
# define the data_url to point to the cloud storage location of the the hyperspectral hdf5 data file
data_url = "https://storage.googleapis.com/neon-aop-products/2021/FullSite/D02/2021_SERC_5/L3/Spectrometer/Reflectance/NEON_D02_SERC_DP3_368000_4306000_reflectance.h5"
# download the h5 data
download_url(data_url,'.\data')
# read the h5 reflectance file (including the full path) to the variable h5_file_name
h5_file_name = data_url.split('/')[-1]
h5_tile = os.path.join(".\data",h5_file_name)
# read in the data using the neon_hs module
serc_refl, serc_refl_md, wavelengths = neon_hs.aop_h5refl2array(h5_tile,'Reflectance')
Reading in .\data\NEON_D02_SERC_DP3_368000_4306000_reflectance.h5
Optionally, you can view the data stored in the metadata dictionary, and print the minimum, maximum, and mean reflectance values in the tile. In order to ignore NaN values, use numpy.nanmin/nanmax/nanmean
.
for item in sorted(serc_refl_md):
print(item + ':',serc_refl_md[item])
print('\nSERC Tile Reflectance Stats:')
print('min:',np.nanmin(serc_refl))
print('max:',round(np.nanmax(serc_refl),2))
print('mean:',round(np.nanmean(serc_refl),2))
EPSG: 32618
bad_band_window1: [1340 1445]
bad_band_window2: [1790 1955]
ext_dict: {'xMin': 368000.0, 'xMax': 369000.0, 'yMin': 4306000.0, 'yMax': 4307000.0}
extent: (368000.0, 369000.0, 4306000.0, 4307000.0)
no_data_value: -9999.0
projection: b'+proj=UTM +zone=18 +ellps=WGS84 +datum=WGS84 +units=m +no_defs'
res: {'pixelWidth': 1.0, 'pixelHeight': 1.0}
scale_factor: 10000.0
shape: (1000, 1000, 426)
source: .\data\NEON_D02_SERC_DP3_368000_4306000_reflectance.h5
SERC Tile Reflectance Stats:
min: -100
max: 15459
mean: 1324.72
For reference, plot the red band of the tile, using splicing, and the plot_aop_refl
function:
sercb56 = serc_refl[:,:,55]/serc_refl_md['scale_factor']
neon_hs.plot_aop_refl(sercb56,
serc_refl_md['extent'],
colorlimit=(0,0.3),
title='SERC Tile Band 56',
cmap_title='Reflectance',
colormap='gist_earth')
We can use pandas
to create a dataframe containing the wavelength and reflectance values for a single pixel - in this example, we'll look at the center pixel of the tile (500,500). To extract all reflectance values from a single pixel, use splicing as we did before to select a single band, but now we need to specify (y,x) and select all bands (using :
).
serc_pixel_df = pd.DataFrame()
serc_pixel_df['reflectance'] = serc_refl[500,500,:]/serc_refl_md['scale_factor']
serc_pixel_df['wavelengths'] = wavelengths
We can preview the first and last five values of the dataframe using head
and tail
:
print(serc_pixel_df.head(5))
print(serc_pixel_df.tail(5))
reflectance wavelengths
0 0.0641 383.884003
1 0.0544 388.891693
2 0.0426 393.899506
3 0.0384 398.907196
4 0.0341 403.915009
reflectance wavelengths
421 1.4949 2492.149414
422 1.4948 2497.157227
423 0.6192 2502.165039
424 1.4922 2507.172607
425 1.4922 2512.180420
We can now plot the spectra, stored in this dataframe structure. pandas
has a built in plotting routine, which can be called by typing .plot
at the end of the dataframe.
serc_pixel_df.plot(x='wavelengths',y='reflectance',kind='scatter',edgecolor='none')
plt.title('Spectral Signature for SERC Pixel (500,500)')
ax = plt.gca()
ax.set_xlim([np.min(serc_pixel_df['wavelengths']),np.max(serc_pixel_df['wavelengths'])])
ax.set_ylim(0,0.6)
ax.set_xlabel("Wavelength, nm")
ax.set_ylabel("Reflectance")
ax.grid('on')
Water Vapor Band Windows
We can see from the spectral profile above that there are spikes in reflectance around ~1400nm and ~1800nm. These result from water vapor which absorbs light between wavelengths 1340-1445 nm and 1790-1955 nm. The atmospheric correction that converts radiance to reflectance subsequently results in a spike at these two bands. The wavelengths of these water vapor bands is stored in the reflectance attributes, which is saved in the reflectance metadata dictionary created with h5refl2array
:
bbw1 = serc_refl_md['bad_band_window1'];
bbw2 = serc_refl_md['bad_band_window2'];
print('Bad Band Window 1:',bbw1)
print('Bad Band Window 2:',bbw2)
Bad Band Window 1: [1340 1445]
Bad Band Window 2: [1790 1955]
Below we repeat the plot we made above, but this time draw in the edges of the water vapor band windows that we need to remove.
serc_pixel_df.plot(x='wavelengths',y='reflectance',kind='scatter',edgecolor='none');
plt.title('Spectral Signature for SERC Pixel (500,500)')
ax1 = plt.gca(); ax1.grid('on')
ax1.set_xlim([np.min(serc_pixel_df['wavelengths']),np.max(serc_pixel_df['wavelengths'])]);
ax1.set_ylim(0,0.5)
ax1.set_xlabel("Wavelength, nm"); ax1.set_ylabel("Reflectance")
#Add in red dotted lines to show boundaries of bad band windows:
ax1.plot((1340,1340),(0,1.5), 'r--');
ax1.plot((1445,1445),(0,1.5), 'r--');
ax1.plot((1790,1790),(0,1.5), 'r--');
ax1.plot((1955,1955),(0,1.5), 'r--');
We can now set these bad band windows to nan
, along with the last 10 bands, which are also often noisy (as seen in the spectral profile plotted above). First make a copy of the wavelengths so that the original metadata doesn't change.
w = wavelengths.copy() #make a copy to deal with the mutable data type
w[((w >= 1340) & (w <= 1445)) | ((w >= 1790) & (w <= 1955))]=np.nan #can also use bbw1[0] or bbw1[1] to avoid hard-coding in
w[-10:]=np.nan; # the last 10 bands sometimes have noise - best to eliminate
#print(w) #optionally print wavelength values to show that -9999 values are replaced with nan
Interactive Spectra Visualization
Finally, we can create a widget
to interactively view the spectra of different pixels along the reflectance tile. Run the cell below, and select different pixel_x and pixel_y values to gain a sense of what the spectra look like for different materials on the ground.
#define refl_band, refl, and metadata, as copies of the original serc_refl data
refl_band = sercb56
refl = serc_refl.copy()
metadata = serc_refl_md.copy()
from IPython.html.widgets import *
def interactive_spectra_plot(pixel_x,pixel_y):
reflectance = refl[pixel_y,pixel_x,:]
pixel_df = pd.DataFrame()
pixel_df['reflectance'] = reflectance
pixel_df['wavelengths'] = w
fig = plt.figure(figsize=(15,5))
ax1 = fig.add_subplot(1,2,1)
# fig, axes = plt.subplots(nrows=1, ncols=2)
pixel_df.plot(ax=ax1,x='wavelengths',y='reflectance',kind='scatter',edgecolor='none');
ax1.set_title('Spectra of Pixel (' + str(pixel_x) + ',' + str(pixel_y) + ')')
ax1.set_xlim([np.min(wavelengths),np.max(wavelengths)]);
ax1.set_ylim([np.min(pixel_df['reflectance']),np.max(pixel_df['reflectance']*1.1)])
ax1.set_xlabel("Wavelength, nm"); ax1.set_ylabel("Reflectance")
ax1.grid('on')
ax2 = fig.add_subplot(1,2,2)
plot = plt.imshow(refl_band,extent=metadata['extent'],clim=(0,0.1));
plt.title('Pixel Location');
cbar = plt.colorbar(plot,aspect=20); plt.set_cmap('gist_earth');
cbar.set_label('Reflectance',rotation=90,labelpad=20);
ax2.ticklabel_format(useOffset=False, style='plain') #do not use scientific notation
rotatexlabels = plt.setp(ax2.get_xticklabels(),rotation=90) #rotate x tick labels 90 degrees
ax2.plot(metadata['extent'][0]+pixel_x,metadata['extent'][3]-pixel_y,'s',markersize=5,color='red')
ax2.set_xlim(metadata['extent'][0],metadata['extent'][1])
ax2.set_ylim(metadata['extent'][2],metadata['extent'][3])
interact(interactive_spectra_plot, pixel_x = (0,refl.shape[1]-1,1),pixel_y=(0,refl.shape[0]-1,1));
Get Lesson Code
Calculate NDVI & Extract Spectra Using Masks in Python
Authors: Bridget Hass
Last Updated: Apr 15, 2024
In this tutorial, we will calculate the Normalized Difference Vegetation Index (NDVI) from hyperspectral reflectance data using Python functions.
This tutorial uses the Level 3 Spectrometer orthorectified surface directional reflectance - mosaic.
Objectives
After completing this tutorial, you will be able to:
- Calculate NDVI from hyperspectral data in Python.
Calculate the mean spectr of all pixels whose NDVI is greater than or less than a specified value.I
Install Python Packages
- requests
- pandas
- gdal
- h5py
Data
Data and additional scripts required for this lesson are downloaded programmatically as part of the tutorial.
The hyperspectral imagery file used in this lesson was collected over the National Ecological Observatory Network's Smithsonian Environmental Research Center field site in 2021 and processed at NEON headquarters.
The entire dataset can be accessed on the NEON Data Portal.
Calculate NDVI & Extract Spectra with Masks
Background:
The Normalized Difference Vegetation Index (NDVI) is a standard band-ratio calculation frequently used to analyze ecological remote sensing data. NDVI indicates whether the remotely-sensed target contains live green vegetation. When sunlight strikes objects, certain wavelengths of the electromagnetic spectrum are absorbed and other wavelengths are reflected. The pigment chlorophyll in plant leaves strongly absorbs visible light (with wavelengths in the range of 400-700 nm) for use in photosynthesis. The cell structure of the leaves, however, strongly reflects near-infrared light (wavelengths ranging from 700 - 1100 nm). Plants reflect up to 60% more light in the near infrared portion of the spectrum than they do in the green portion of the spectrum. By calculating the ratio of Near Infrared (NIR) to Visible (VIS) bands in hyperspectral data, we can obtain a metric of vegetation density and health.
The formula for NDVI is: $$NDVI = \frac{(NIR - VIS)}{(NIR+ VIS)}$$
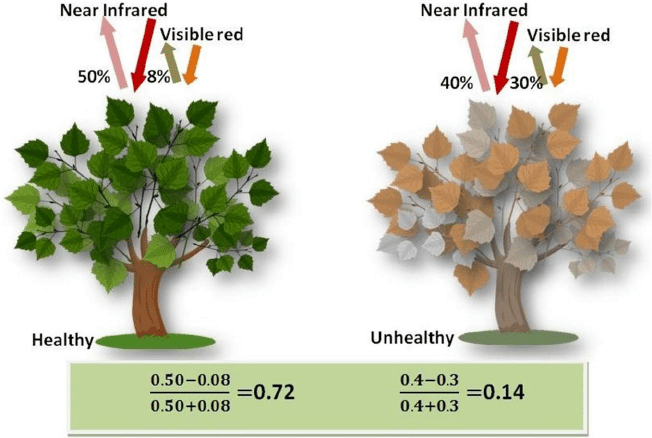
Start by setting plot preferences and loading the neon_aop_hyperspectral.py module:
import os, sys
from copy import copy
import requests
import numpy as np
import pandas as pd
import matplotlib.pyplot as plt
This next function is a handy way to download the Python module and data that we will be using for this lesson. This uses the requests
package.
# function to download data stored on the internet in a public url to a local file
def download_url(url,download_dir):
if not os.path.isdir(download_dir):
os.makedirs(download_dir)
filename = url.split('/')[-1]
r = requests.get(url, allow_redirects=True)
file_object = open(os.path.join(download_dir,filename),'wb')
file_object.write(r.content)
Download the module from its location on GitHub, add the python_modules to the path and import the neon_aop_hyperspectral.py module as neon_hs
.
# download the neon_aop_hyperspectral.py module from GitHub
module_url = "https://raw.githubusercontent.com/NEONScience/NEON-Data-Skills/main/tutorials/Python/AOP/aop_python_modules/neon_aop_hyperspectral.py"
download_url(module_url,'../python_modules')
# add the python_modules to the path and import the python neon download and hyperspectral functions
sys.path.insert(0, '../python_modules')
# import the neon_aop_hyperspectral module, the semicolon supresses an empty plot from displaying
import neon_aop_hyperspectral as neon_hs;
# define the data_url to point to the cloud storage location of the the hyperspectral hdf5 data file
data_url = "https://storage.googleapis.com/neon-aop-products/2021/FullSite/D02/2021_SERC_5/L3/Spectrometer/Reflectance/NEON_D02_SERC_DP3_368000_4306000_reflectance.h5"
# download the h5 data and display how much time it took to download (uncomment 1st and 3rd lines)
# start_time = time.time()
download_url(data_url,'.\data')
# print("--- It took %s seconds to download the data ---" % round((time.time() - start_time),1))
Read in SERC Reflectance Tile
# read the h5 reflectance file (including the full path) to the variable h5_file_name
h5_file_name = data_url.split('/')[-1]
h5_tile = os.path.join(".\data",h5_file_name)
print(f'h5_tile: {h5_tile}')
h5_tile: .\data\NEON_D02_SERC_DP3_368000_4306000_reflectance.h5
# Note you will need to update this filepath for your local machine
serc_refl, serc_refl_md, wavelengths = neon_hs.aop_h5refl2array(h5_tile,'Reflectance')
Reading in .\data\NEON_D02_SERC_DP3_368000_4306000_reflectance.h5
Extract NIR and VIS bands
Now that we have uploaded all the required functions, we can calculate NDVI and plot it. Below we print the center wavelengths that these bands correspond to:
print('band 58 center wavelength (nm): ', wavelengths[57])
print('band 90 center wavelength (nm) : ', wavelengths[89])
band 58 center wavelength (nm): 669.3261
band 90 center wavelength (nm) : 829.5743
Calculate & Plot NDVI
Here we see that band 58 represents red visible light, while band 90 is in the NIR portion of the spectrum. Let's extract these two bands from the reflectance array and calculate the ratio using the numpy.true_divide
which divides arrays element-wise. This also handles a case where the denominator = 0, which would otherwise throw a warning or error.
vis = serc_refl[:,:,57]
nir = serc_refl[:,:,89]
# handle a divide by zero by setting the numpy errstate as follows
with np.errstate(divide='ignore', invalid='ignore'):
ndvi = np.true_divide((nir-vis),(nir+vis))
ndvi[ndvi == np.inf] = 0
ndvi = np.nan_to_num(ndvi)
Let's take a look at the min, mean, and max values of NDVI that we calculated:
print(f'NDVI Min: {ndvi.min()}')
print(f'NDVI Mean: {round(ndvi.mean(),2)}')
print(f'NDVI Max: {ndvi.max()}')
NDVI Min: -1.0
NDVI Mean: 0.63
NDVI Max: 1.0
We can use the function plot_aop_refl
to plot this, and choose the seismic
color pallette to highlight the difference between positive and negative NDVI values. Since this is a normalized index, the values should range from -1 to +1.
neon_hs.plot_aop_refl(ndvi,serc_refl_md['extent'],
colorlimit = (np.min(ndvi),np.max(ndvi)),
title='SERC Subset NDVI \n (VIS = Band 58, NIR = Band 90)',
cmap_title='NDVI',
colormap='seismic')
Extract Spectra Using Masks
In the second part of this tutorial, we will learn how to extract the average spectra of pixels whose NDVI exceeds a specified threshold value. There are several ways to do this using numpy
, including the mask functions numpy.ma
, as well as numpy.where
and finally using boolean
indexing.
To start, lets copy the NDVI calculated above and use booleans to create an array only containing NDVI > 0.6.
# make a copy of ndvi
ndvi_gtpt6 = ndvi.copy()
#set all pixels with NDVI < 0.6 to nan, keeping only values > 0.6
ndvi_gtpt6[ndvi<0.6] = np.nan
print('Mean NDVI > 0.6:',round(np.nanmean(ndvi_gtpt6),2))
Mean NDVI > 0.6: 0.87
Now let's plot the values of NDVI after masking out values < 0.6.
neon_hs.plot_aop_refl(ndvi_gtpt6,
serc_refl_md['extent'],
colorlimit=(0.6,1),
title='SERC Subset NDVI > 0.6 \n (VIS = Band 58, NIR = Band 90)',
cmap_title='NDVI',
colormap='RdYlGn')
Calculate the mean spectra, thresholded by NDVI
Below we will demonstrate how to calculate statistics on arrays where you have applied a mask numpy.ma
. In this example, the function calculates the mean spectra for values that remain after masking out values by a specified threshold.
import numpy.ma as ma
def calculate_mean_masked_spectra(refl_array,ndvi,ndvi_threshold,ineq='>'):
mean_masked_refl = np.zeros(refl_array.shape[2])
for i in np.arange(refl_array.shape[2]):
refl_band = refl_array[:,:,i]
if ineq == '>':
ndvi_mask = ma.masked_where((ndvi<=ndvi_threshold) | (np.isnan(ndvi)),ndvi)
elif ineq == '<':
ndvi_mask = ma.masked_where((ndvi>=ndvi_threshold) | (np.isnan(ndvi)),ndvi)
else:
print('ERROR: Invalid inequality. Enter < or >')
masked_refl = ma.MaskedArray(refl_band,mask=ndvi_mask.mask)
mean_masked_refl[i] = ma.mean(masked_refl)
return mean_masked_refl
We can test out this function for various NDVI thresholds. We'll test two together, and you can try out different values on your own. Let's look at the average spectra for healthy vegetation (NDVI > 0.6), and for a lower threshold (NDVI < 0.3).
serc_ndvi_gtpt6 = calculate_mean_masked_spectra(serc_refl,ndvi,0.6)
serc_ndvi_ltpt3 = calculate_mean_masked_spectra(serc_refl,ndvi,0.3,ineq='<')
Finally, we can create a pandas
dataframe to plot the mean spectra.
#Remove water vapor bad band windows & last 10 bands
w = wavelengths.copy()
w[((w >= 1340) & (w <= 1445)) | ((w >= 1790) & (w <= 1955))]=np.nan
w[-10:]=np.nan;
nan_ind = np.argwhere(np.isnan(w))
serc_ndvi_gtpt6[nan_ind] = np.nan
serc_ndvi_ltpt3[nan_ind] = np.nan
#Create dataframe with masked NDVI mean spectra, scale by the reflectance scale factor
serc_ndvi_df = pd.DataFrame()
serc_ndvi_df['wavelength'] = w
serc_ndvi_df['mean_refl_ndvi_gtpt6'] = serc_ndvi_gtpt6/serc_refl_md['scale_factor']
serc_ndvi_df['mean_refl_ndvi_ltpt3'] = serc_ndvi_ltpt3/serc_refl_md['scale_factor']
Let's take a look at the first 5 values of this new dataframe:
serc_ndvi_df.head()
wavelength | mean_refl_ndvi_gtpt6 | mean_refl_ndvi_ltpt3 | |
---|---|---|---|
0 | 383.884003 | 0.055741 | 0.119835 |
1 | 388.891693 | 0.036432 | 0.090972 |
2 | 393.899506 | 0.027002 | 0.076867 |
3 | 398.907196 | 0.022841 | 0.072207 |
4 | 403.915009 | 0.018748 | 0.065984 |
Plot the masked NDVI dataframe to display the mean spectra for NDVI values that exceed 0.6 and that are less than 0.3:
ax = plt.gca();
serc_ndvi_df.plot(ax=ax,x='wavelength',y='mean_refl_ndvi_gtpt6',color='green',
edgecolor='none',kind='scatter',label='Mean Spectra where NDVI > 0.6',legend=True);
serc_ndvi_df.plot(ax=ax,x='wavelength',y='mean_refl_ndvi_ltpt3',color='red',
edgecolor='none',kind='scatter',label='Mean Spectra where NDVI < 0.3',legend=True);
ax.set_title('Mean Spectra of Reflectance Masked by NDVI')
ax.set_xlim([np.nanmin(w),np.nanmax(w)]);
ax.set_xlabel("Wavelength, nm"); ax.set_ylabel("Reflectance")
ax.grid('on');