Case Study
Automating Biodiversity Surveys at NEON Field Sites
March 10, 2022
Most biodiversity data at the NEON field sites is collected the old-fashioned way: by humans traipsing through the fields and woods, gathering specimens or noting observations. But modern instrumentation and machine learning methods are increasingly used in the ecology community to supplement human effort. Could some of these methods be applied at the NEON field sites? A recent paper in Ecosphere - Expanding NEON biodiversity surveys with new instrumentation and machine learning approaches - explores the possibilities.
Who's There? Just Listen!
Dr. Justin Kitzes, an assistant professor of Biological Sciences at the University of Pittsburgh, initially proposed the idea as a topic for a working group at the October 2020 NEON Science Summit. Kitzes, who focuses on quantitative ecology, sits on two NEON Technical Working Groups (TWGs): Breeding Birds and Data Standards.
In his own research, he uses autonomous acoustic recorders and machine learning algorithms to identify and classify birds and anurans (frogs and toads) from their calls. He and his students and colleagues place more than 1000 recording devices each year in field sites across North and South America. In Pennsylvania, he is monitoring large-scale forest restoration projects supported by the National Fish and Wildlife Foundation.
"We're looking for focal species in these areas—golden wing warblers, cerulean warblers, wood thrushes and other species—to see if they are using the habitat as hoped," he explains. "The acoustic recorders allow us to listen in without disturbing the wildlife to see what species are actually there. It's one way to monitor the success of the restoration project."
Acoustic recording devices are just one example of automated sensors that can be used for biodiversity surveying. The working group also explored several others, including wildlife cameras for mammals, hydroacoustic sensors and remote sensing for aquatic species, expanded remote and ground-based sensor measurements for plant biodiversity, and laboratory analysis of the NEON physical specimen collections.
The Rise of Automation in Ecology
Automated instruments have shown tremendous promise for collection of biodiversity data. A fully automated system typically includes two parts:
- A sensor that collects visual, acoustic, or other data that provides evidence of the presence of a species in an area. These could include camera traps, acoustic sensors, or remote sensing technologies (e.g., imaging spectrometer). Some types of sensors can be automated so they only collect data when triggered (e.g., by motion or sound). This reduces collection of data that are not meaningful for ecologists. For example, a wildlife camera trap typically uses a motion sensor so that it only records when an animal is within range of the camera.
- A machine learning algorithm that is capable of processing the data and providing preliminary identification of a species. The program may use visual or acoustic signatures for species identification. These programs must be trained using large datasets so that they learn to distinguish, for example, the call of a cerulean warbler from that of a golden wing warbler, or the profile and markings of a bobcat vs. a common housecat. Once they are trained, they can quickly process vast amounts of recorded data and pull out the data of interest to ecologists, who can confirm the species identification.
Automated sensing and identification systems enable collection of much more data than is possible by human field collection alone. Sensors can be left in the field 24/7/365 to record events that may happen diurnally or seasonally. And unlike human observers, they do not disrupt the wildlife researchers are trying to record.
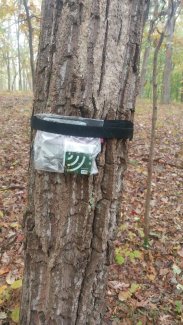
Pilot deployment at SCBI in Front Royal in 2019. An AudioMoth recorder is hung on a tree in a Ziploc bag. Photo credit: Justin Kitzes.
Kitzes says that automation is particularly helpful in detecting events that are very rare or hard to capture. It is also a better way to capture "firsts" in a season, such as the first birdsong that heralds the return of a species in the spring. "One of the things instruments are really good at is waiting around. They don't care if it's raining or snowing, and they don't have to sleep. So, they are particularly good at detecting events that are very rare, such as the call of a rare species, or recording the first time something happens in a year. When humans are in the field, they would have to be there at just the right time to capture these events. The instruments can just wait there until it happens."
Biodiversity, Automation, and the NEON Program
Researchers have used instrument data to explore biodiversity in a variety of ways. For example, the phenocams and remote sensing data (hyperspectral and lidar) from the NEON Airborne Observation Platform (AOP) have been used by researchers to study plant community composition, including tree species classification via machine learning using lidar and hyperspectral data. Researchers have also investigated the use of machine learning to classify beetles and other taxa from photos taken of pitfall trap catches. Additional and more sophisticated machine learning algorithms could be used to extract other kinds of biodiversity data from existing instrument systems or NEON Biorepository collections.
As suggested by Kitzes and coauthors, adding new instrumentation—such as acoustic recorders, camera traps, and sonar and remote imaging technologies for aquatic sites—would further expand the types of biodiversity data that researchers could derive from NEON data. Camera traps, for example, would enable study of medium-to-large mammal populations that are not currently included in the NEON program design. Acoustic recorders would provide valuable insights into the presence of many different species of birds, bats, insects, and anurans—taxa that are either currently not covered at all by the NEON program or are likely to be missed by human observation or specimen collection methods such as pitfall traps. And additional aquatic instrumentation could supplement labor-intensive human surveys of fish species presence and abundance and enable better monitoring of rare, migratory, or invasive species.
These instruments are beyond the scope of the original NEON instrument system design. When the NEON program was scoped many years ago, many of these instruments and methods were not yet in widespread use. Adding them now would require additional funding support from the NSF for instrumentation and significant work to write new data collection protocols. However, adding new instruments to NEON field sites can happen through the NEON Assignable Assets program. Under this program, a researcher can apply for permission to add instrumentation to existing NEON infrastructure in a cost-recoverable arrangement to one or more NEON field sites.
Other suggestions made by Kitzes and coauthors would require fewer resources to implement. The physical specimens archived by the NEON Biorepository represent a treasure trove of data that could be used to support biodiversity studies. Machine learning is already being applied for taxonomic classification of carabids collected in the pitfall traps. Imaging of other types of organisms—including aquatic and benthic invertebrates, terrestrial invertebrates now classified as "bycatch" in the carabid pitfall traps, and other physical plant and animal specimens—would open new possibilities for machine learning classification and species identification using the specimens that already exist in the archive.
Kitzes is enthusiastic about the possibilities of automated instruments and machine learning for biodiversity surveys. He says, "Our group is interested in studying biodiversity at large scales across both time and space. When you look at it that way, you start to realize how much of the planet has not been surveyed. Currently, we try to fill those holes statistically, but that's not the same as having actual observations—especially when you are looking for rare species and events. These technologies can be used to augment human efforts and vastly expand the scale of biodiversity data collection."