BeetlePalooza! Advancing AI-Enabled Beetle Identification and Trait Measurement
October 23, 2024
BeetlePalooza 2024, hosted by the Imageomics Institute at The Ohio State University (OSU), brought together a diverse group of biologists, ecologists, and computer scientists to develop and refine AI tools for beetle identification and trait measurement. These AI tools could save substantial time for field ecologists and enable new discoveries, made possible only with very large image-based datasets.
What’s Imageomics?
Imageomics is an emerging field at the intersection of biology, data science, and artificial intelligence (AI). It leverages machine learning and computer vision to extract information from large sets of biological images. Machine learning (ML) is a form of AI that allows computers to find and learn from patterns in data to make predictions or decisions without being explicitly programmed. Computer vision allows machines to “see” and interpret images by recognizing objects, shapes, and image features. Together, these technologies enable scientists to analyze and understand biological images at a scale that would be impossible manually.
The Imageomics Institute was established and is led by Dr. Tanya Berger-Wolf, Director of the Translational Data Analytics Institute and a Professor of Computer Science Engineering, Electrical, and Computer Engineering, as well as Evolution, Ecology, and Organismal Biology at OSU. The Institute is supported by a U.S. National Science Foundation (NSF) grant as part of the Harnessing the Data Revolution (HDR) initiative. Dr. Paula Mabee, the Chief Scientist and Observatory Director for the National Ecological Observatory Network (NEON) program, brings her background in semantics and evolutionary biology to the research team and serves as the Director of HDR Ecosystem Coordination for the Imageomics Institute.
Moving Forward in AI… With Beetles
BeetlePalooza 2024 was held this August in Columbus, Ohio. The four-day event was funded by NSF Imageomics awards and hosted 30 participants representing a diverse mix of interests, backgrounds, and perspectives. Computing resources were provided by CyVerse. The organizing committee included Hilmar Lapp, a Research Scientist at Duke University in the Department of Biostatistics and Bioinformatics; Dr. Sydne Record, Associate Professor of Landscape Conservation at the University of Maine in the Department of Wildlife, Fisheries and Conservation Biology (also the lead member of the NEON Science, Technology & Education Advisory Committee); Michelle Ramirez, a graduate student and researcher with the Imageomics Institute; and Dr. Eric Sokol, a staff scientist in quantitative ecology at NEON.
Why beetles? NEON observes ground beetles because they are considered sentinel taxa for understanding ecosystem change. Additionally, Record explains, “Ground beetles are one of the most species-rich animal families on Earth, so it presents an interesting challenge for species identification. We also wanted to measure traits in different beetles to look at how traits might influence community assembly and patterns in biodiversity.” (For a prior look at the use of AI in beetle identification, see our 2021 blog “What’s That Beetle? Ask the Algorithm.”)
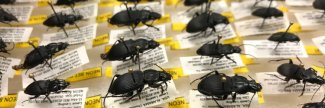
Beetle samples
There are more than 40,000 known species in the ground beetle family (Latin name Carabidae), with roughly 2000 found in North America. Making an exact species identification—or even narrowing it down to the tribe or sub-family—can be quite challenging for field ecologists. Many species are differentiated by minute trait differences that can be difficult to spot with the human eye. That’s where computer vision and ML come in.
BeetlePalooza provided an opportunity to bring people together to advance the science of imageomics as it relates to ground beetles and other insects. “This was very much a hands-on, collaborative workshop, where people worked together to solve problems,” says Lapp, the Director of Informatics and Infrastructure for the Imageomics Institute and a Co-principal Investigator on the NSF grant supporting the event.
After day one, workshop attendees split into six participant-led subgroups to work on problems defined by each group. Most of the groups worked with images of beetles collected at the NEON field sites and archived in the NEON Biorepository. Photographs were taken by Isadora Fluck, a graduate student at the University of Florida, who had previously collaborated with Record. One group (the “bucket of bugs” group) worked with a different image library of flying nocturnal insects in Panama.
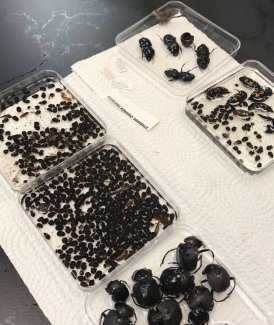
Ground beetles from one trap at Ordway-Swisher Biological Station (OSBS), FL.
Some of the groups worked with BioCLIP, an AI model designed to process and analyze biological images and classify them according to taxonomic labels from the “Tree of Life.” One subgroup focused on developing species distribution models to predict suitable habitats of beetle species under various climate scenarios. Another integrated other NEON environmental data and metadata into species images to improve the accuracy of beetle identification.
Trait measurement was another area of interest. “Traits are the currency of natural selection,” says Record. “Individual variation in traits influences which individuals in a species are able to survive and pass on their genes. AI creates a whole new opportunity within biology to automate measurement of different traits from images of biological specimens in museums and archives. That opens up new avenues of research that become possible when you can significantly scale up measurement of these traits. For example, we can start to look at which traits matter most for biodiversity patterns.”
The BeetlePalooza workshop is the latest of several events held by the Imageomics Institute in recent years to advance the use of AI tools in biology and ecology. Lapp says, “Part of our role at the Institute is to dramatically lower the barriers for scientists to use, reuse, and build upon these products, including models and software. This kind of workshop is a perfect venue to do that and to surface the opportunities and friction points in the field.”
Advancing Ecology with AI
AI is poised to revolutionize biology and ecology—and species identification and trait measurements may only be the beginning. By automating some of the most arduous and time-consuming tasks in field ecology, image-based AI can increase data availability by orders of magnitude and free up researchers to focus on analysis and interpretation.
Record says, “In the past, we’ve been limited by the people power required for these kinds of analyses. Now, a task that took two undergraduates and a graduate student all summer to complete could be reduced to just a couple of days using these automation tools.” While the NEON program does not currently take images of ground beetles or other organismal specimens as part of its regular protocol, Record believes that adding image-based AI tools for species identification and trait measurement has the potential to generate significant cost savings for the Observatory in the future.
Computer vision may also be able to see things that humans just can’t. For example, computers may highlight patterns in butterfly wings that humans have not previously paid attention to, but turn out to be important for bird predators. In addition to images, AI could be applied to other areas of data, such as audio recordings of bird songs or insect noises.
Record also notes that AI tools like ChatGPT have made programming faster and more accessible for scientists with limited computer science experience. Large language models can be leveraged to assist in coding for R packages and other programming environments by generating code snippets, explaining complex functions, debugging, and even optimizing existing code. “It’s literally changing how people are analyzing ecological data, which is helping us move the needle forward faster,” she says.
She is especially excited by the opportunities created by NEON’s open-access ecology data. The beetle specimens imaged for the workshop come with rich metadata and an extensive compilation of concurrently collected data products, including meteorological, soil, and vegetation data. This allows beetle images to be analyzed within the context of the environment in which the specimen was collected. “There are a lot of great opportunities with NEON because of the different types of data that are collected concurrently using standardized protocols,” Record says. “This is the bread and butter of the kind of data you need to run these multimodal AI models. AI is going to enable discoveries that have been hindered by limited people power and data availability.”