Case Study
How Permanent is Permafrost? AI Might Help Us Find the Answer
March 27, 2024
Permafrost degradation is an urgent concern for Arctic ecosystems and the global climate. However, more work is needed to understand the relationships and feedback loops between permafrost thaw, greenhouse gas emissions, and climate variables.
A recent paper in Environmental Research Letters, led by NASA JPL’s Dr. Bradley Gay, leverages artificial intelligence (AI) to tease out these complex interrelationships and develop better predictive models of permafrost degradation. The researchers utilized data from NEON’s terrestrial field sites in Alaska to train the model, along with data from the AmeriFlux network.
Permafrost Thaw, Emissions, and Climate Change
“Most people assume permafrost is, well, permanent, but the name is a bit of a misnomer,” says Gay. “It’s very complex, and it’s changing rapidly. We should all be worried about permafrost right now because it is thawing at an exponential rate. In the past, it has been a gradual freeze-thaw process in alignment with past growing and cold seasons. And now we're seeing these abrupt shifts, and not only the expansion of the growing season but the shrinking of the cold season.”
Dr. Bradley Gay is a Postdoctoral Fellow at Caltech and NASA Jet Propulsion Laboratory (JPL). His work with Dr. Charles E. Miller at JPL focuses on permafrost carbon modeling and understanding drivers of change in the Arctic in a warming global climate.
Permafrost is characterized by a layer of frozen soil below the Earth's surface that remains frozen year-round for at least two consecutive years. Found in high-latitude Arctic, sub-Arctic, and some alpine regions, it contains large amounts of organic material and trapped gases like carbon dioxide and methane. Permafrost distribution has profoundly shaped Tundra and Taiga ecosystems by creating unique ground conditions that affect the carbon and water cycles, vegetation cover, and animal community composition.
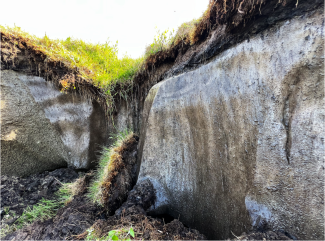
Thermokarst and exposed ice wedge east of the Katakturuk River in the Alaska National Wildlife Refuge, Alaska. Photo credit: Bradley Gay (July 2022)
Permafrost thaw has been escalating significantly in recent decades due to global warming. Thawing permafrost has potential cascading consequences for local ecosystems and the global climate system. As the underlying frozen layers thaw, this can lead to ground subsidence (sinking or settling of the ground surface), affecting natural habitats and human infrastructure. The permafrost layer also acts as a massive store of greenhouse gases. The rapid thawing of permafrost layers that have remained frozen for hundreds of thousands to millions of years is predicted to release large amounts of carbon and methane into the atmosphere, potentially accelerating climate change further. Thawing also increases moisture in the soil, which supports microbial activity as soil warms, creating an amplified feedback mechanism that further increases warming locally.
“These feedback loops escalate local warming through the greenhouse effect,” Gay explains, “When there is increased thaw, the permafrost releases a bunch of CO2 and methane into the local atmosphere. Those gases prevent the reflected solar radiation from returning to space, so energy gets trapped and reflected downward. And that further increases the surface temperature. As soil heats up, you will see more microbial decomposition activity, which produces even more CO2, and other biotic and abiotic processes release more carbon and methane into the atmosphere. This all contributes to a feedback mechanism that further accelerates permafrost thaw.”
However, there is much uncertainty about how these feedback mechanisms work and how fast this is all happening. By some estimates, most of Earth’s near-surface permafrost (the upper 10-13 feet of the soil layer) will be gone by 2100, which could have devastating effects on humans and the environment. In addition to accelerating global warming, melting permafrost will substantially alter the landscape and hydrology of Arctic and sub-Arctic habitats, increase the risk of landslides, floods, and coastal erosion, and impact plant, animal, and microbial diversity across the northern latitudes. Melting permafrost will also destabilize human infrastructure, with disproportionate effects on First Nations and Indigenous peoples who have inhabited these lands for thousands of years.
Leveraging AI for Earth-System Modeling
Gay and his co-authors believe that artificial intelligence (AI) can help us build better models of permafrost carbon feedback (PCF) mechanisms. They created an AI system called GeoCryoAI to model permafrost thawing and carbon and methane emissions from permafrost. GeoCryoAI is a deep learning algorithm specifically tailored to uncover patterns in data from permafrost-affected landscapes, such as thaw depth and carbon and methane exchange between the landscape and the atmosphere. By applying AI to the problem of permafrost feedback loops, they hope to uncover the drivers and mechanisms of permafrost degradation and quantify the uncertainty in permafrost models. Deep learning is a subset of machine learning (ML) that structures algorithms in layers to create an “artificial neural network” that can learn from vast amounts of data and make intelligent decisions or conclusions.
The study ultimately used field data from 790 sites in Alaska spanning more than five decades. But first, the system had to be trained. The researchers used field data from NEON and AmeriFlux to train and validate the system. Gay says that NEON data are valuable as a training set. “While NEON doesn’t have the spatial coverage represented by AmeriFlux and other flux networks in Alaska, the richness and consistency of the data is ideal for the teacher forcing process,” he explains. “For example, the sampling rate for some tower instruments ranges from 10- to 30-minute intervals with at least a kilometer of reach. This richness allows us to see what is happening at a very fine resolution with all these different features, including things happening above and below ground.”
The researchers trained the model using the first seven years of a myriad of data products from NEON’s Arctic terrestrial sites (BONA, DEJU, HEAL, TOOL, and BARR), along with comparable data from some AmeriFlux sites. Then, they validated the model by using it to make thaw depth and carbon flux predictions for the next three years, and compared these forecasts with actual field data. Gay says, “With the training data set, the model is learning about all these dynamics, like soil moisture, air temperature, and how patterns emerge as these variables interact. Then, it logs those patterns to memory. Once you have that kind of structure or understanding, you can modulate the temporal window to project forward two or three years and then compare to actual data to ensure that what its learning aligns with what happened for the past three years.”
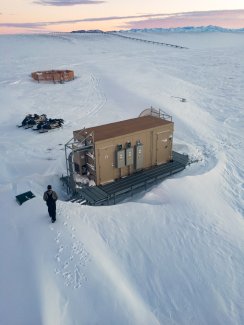
View from the TOOL tower (2017)
Overall, the model did better at predicting carbon dioxide and methane flux than thaw depth. Gay says this is not surprising, as they had much finer resolution data for gas fluxes vs. soil measurements in the training set. Gay and his co-authors already have a second paper with GeoCryoAI under review, which expands the model spatially with imaging spectroscopy and process-based modeling (PBM). His ongoing research continues to spatially expand and spectrally enrich the model with process-based models (PBM) and imaging spectroscopy while future efforts will focus on extending the model across the Circumarctic, using available field-based and remotely sensed data from Canada, Scandinavia, and Siberia.
Currently, Gay is including PBM outputs and airborne remote sensing data to find proxies to measure and model permafrost degradation over larger spatial areas. Data from UAVSAR (radar instruments mounted on aircraft) or the NEON Airborne Observation Platform (AOP), for example, can monitor landscapes for subsidence and deformation that may indicate underlying thawing of the permafrost layer and for changes in vegetation that are correlated with warming soil temperatures and deeper thaw depth. The NASA-ISRO Synthetic Aperture Radar (NISAR) satellite mission, planned for launch in the spring of 2024, will provide fine-grained measurements of land- and ice-covered surfaces with an average sampling frequency of twice over 12 days. Gay says, “We've been using UAVSAR for some time to measure subsidence up in Alaska and even in the contiguous U.S. to see how terradeformation changes over time. This stage aims to see if we can use remote sensing data to make the same kind of models and forecasts for permafrost thaw and carbon fluxes.”
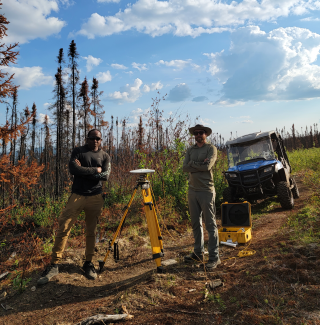
Airborne Sensor Operators Mike Mussow and Cam Chapman set up ground-truthing points using a GPS at D19 (Taiga) site BONA. Photo credit: Cam Chapman.
Gay emphasizes the importance of validating remote sensing data with on-the-ground measurements from field sampling and flux towers. The NEON field sites were helpful for validation and he hopes more funding and resources become available to expand in situ databases, enable data accessibility, and improve network representation across Alaska and the Circumarctic. He says, “There is no substitute to being on the ground and actually seeing and even smelling what's going on.”
The Future of AI and Ecology
Moving forward, the role of AI in ecology and the cryosphere (the regions on Earth where water freezes) is likely to grow. Gay says, “From a research standpoint, I think it will be transformative; in fact, it already is. It's significantly cutting down time for pre-processing data, for example. That means researchers have more time to not only synthesize the data, write papers, and get them out but also to ask new questions and have the time to explore them. Ultimately, it will allow us to look at things in new ways.”
Researchers will need to be careful about taking AI findings at face value. As AI algorithms become more advanced and sophisticated, these tools also become more opaque to human observers. Deep learning models, in particular, can be highly complex and act as “black boxes,” making it challenging to interpret how conclusions are reached. AI models can also inherit biases in the training data, leading to skewed or inaccurate predictions. Moreover, models trained on data from specific regions or conditions might generalize poorly to other contexts. In some cases, AI tools like large language models and deep learning algorithms have even been known to fabricate data. Gay cautions that researchers should watch out for findings that “raise their eyebrows” and do not align well with the overall body of evidence.
Still, he is highly optimistic about using deep learning and other AI tools in science. Gay is especially excited about the potential for process-guided data-driven models like GeoCryoAI to help ecologists explore mechanisms and drivers of ecological processes. More broadly, he believes the intersection of AI and “big ecology data” will be transformative for the discipline as a whole. He says, “Physics is universal and critical, but having data also provides insight. If we have these two tools, they can work synergistically to better understand our environment. I think we should certainly use them.”