Workshop
A Hands-On Primer for Working with Big Data in R – Introduction to Hierarchical Data Formats, Lidar Data & Efficient Data Visualization | ESA 2016
Ecological Society of America Annual Meeting
Ecologists working across scales and integrating disparate datasets face new data management and analysis challenges that demand tools beyond the spreadsheet. This workshop will overview three key data formats: ASCII, HDF5 and las and several key data types including temperature data from a tower, vegetation structure data, hyperspectral imagery and lidar data, that are often encountered when working with ‘Big Data’. It will provide an introduction to available tools in R for working with these formats and types.
Things to Do Before the Workshop
To participant in this workshop, you will need a laptop with the most current version of R and, preferably, RStudio loaded on your computer. For details on setting up R & RStudio in Mac, PC, or Linux operating systems please see Additional Set up Resources below.
Install R Libraries
Please install or update each package prior to the start of the workshop.
-
rgeos:
install.packages("rgeos")
-
maptools:
install.packages("maptools")
-
raster:
install.packages("raster")
-
rgdal:
install.packages("rgdal")
-
ggplot2:
install.packages("ggplot2")
-
rhdf5:
source("http://bioconductor.org/biocLite.R") biocLite("rhdf5")
-
ggplot2:
install.packages("ggplot2")
-
dpylr:
install.packages("dplyr")
: data manipulation at its finest!
Data to Download
[[nid:6332]] [[nid:6330]] [[nid:6420]]
After downloading the data, please uncompress and set your R working directory to the parent directory containing these files. For additional instructions see the bottom of the page. We will be setting up an RStudio project within this working directory. Read more about RStudio projects here.
Background Reading
This workshop will be most useful to those with some basic understanding of spatial & hierarchical data. Please read the following materials if you would like additional background on these topics.
- Read more about the HDF5 viewer
- A brief introduction to Lidar Data
- About the basic Lidar Derived Data Products - CHM, DEM, DSM
- A brief intro to the Hierarchical Data Format (HDF5) format.
- A brief intro to Hyperspectral Remote Sensing data format.
- A 5-minute video on imagery/optical remote sensing.
-
Documentation for the
raster
package in R.
Workshop Instructors
- Leah Wasser @leahawasser, Supervising Scientist, NEON, Inc
- Natalie Robinson, Staff Scientist, NEON, Inc
Workshop Fearless Instruction Assistants
- Claire Lunch @dr_lunch, Staff Scientist
- Kate Thibault @fluby, Senior Staff Scientist
- Christine Laney @cmlaney, Staff Scientist, NEON, Inc
- Mike Smorul @msmorul, Associate Director of Cyberinfrastructure, SESYNC
- Philippe Marchand, Scientific Support Specialist, SESYNC
Social Media
Please tweet using the hashtag #WorkWithData during this workshop! Also you can tweet at @NEON_Sci !
Schedule
Location: ESA Annual Meeting, Baltimore, Maryland
The schedule below is subject to change.
Time | Topic | Instructor |
---|---|---|
8:00 | Welcome, Introductions, & Logistics | |
8:05 | Getting Started with Rasters in R | Natalie |
9:30 | Raster Resolution, Extent & CRS in R | Natalie |
10:15 | ------- BREAK ------- | |
10:30 | LiDAR Data Derived Rasters in R | Leah |
11:45 - 1:00 PM | Lunch on Your Own | |
1:00 | Introduction to HDF5 in R | Leah |
2:30 | ------- BREAK ------- | |
2:45 | Hyperspectral Imagery in R | Leah |
Create Raster Stacks & NDVI in R (if time allows) | Leah | |
3:45 | ------- BREAK ------- | |
4:00 | Application of Skills with Capstone Project |
Additional Set Up Instructions
R & RStudio
Prior to the workshop you should have R and, preferably, RStudio installed on your computer.
[[nid:6408]] [[nid:6512]]
Install HDFView
The free HDFView application allows you to explore the contents of an HDF5 file.
To install HDFView:
-
Click to go to the download page.
-
From the section titled HDF-Java 2.1x Pre-Built Binary Distributions select the HDFView download option that matches the operating system and computer setup (32 bit vs 64 bit) that you have. The download will start automatically.
-
Open the downloaded file.
- Mac - You may want to add the HDFView application to your Applications directory.
- Windows - Unzip the file, open the folder, run the .exe file, and follow directions to complete installation.
- Open HDFView to ensure that the program installed correctly.
QGIS (Optional)
QGIS is a cross-platform Open Source Geographic Information system.
Online LiDAR Data/las Viewer (Optional)
Plas.io is a open source LiDAR data viewer developed by Martin Isenberg of Las Tools and several of his colleagues.
Time | Topic | Instructor |
---|---|---|
8:00 | Welcome, Introductions & Logistics | |
8:05 | Getting Started with Rasters in R | Natalie |
9:30 | Raster Resolution, Extent & CRS in R | Natalie |
10:15 | ------- BREAK ------- | |
10:30 | LiDAR Derived Rasters in R | Leah |
11:45 - 1:00 PM | Lunch on Your Own | |
1:00 | Introduction to HDF5 in R | Leah |
2:30 | ------- BREAK ------- | |
2:45 | Hyperspectral Imagery in R | Leah |
Create Raster Stacks & NDVI in R (If time permits) | Leah | |
3:45 | ------- BREAK ------- | |
4:00 | Application of Skills: Capstone Activity |
Workshop Notes
Visit the Workshop Etherpad to review collaborative notes from the workshop.
Raster Data in R - The Basics
This activity will walk you through the fundamental principles of working with raster data in R.
Learning Objectives
After completing this activity, you will be able to:
- Describe what a raster dataset is and its fundamental attributes.
- Import rasters into R using the raster library.
- Perform raster calculations in R.
Things You’ll Need To Complete This Tutorial
You will need the most current version of R and, preferably, RStudio
loaded
on your computer to complete this tutorial.
Install R Packages
-
raster:
install.packages("raster")
-
rgdal:
install.packages("rgdal")
Data to Download
NEON Teaching Data Subset: Field Site Spatial Data
These remote sensing data files provide information on the vegetation at the National Ecological Observatory Network's San Joaquin Experimental Range and Soaproot Saddle field sites. The entire dataset can be accessed by request from the NEON Data Portal.
Download DatasetThe LiDAR and imagery data used to create the rasters in this dataset were collected over the San Joaquin field site located in California (NEON Domain 17) and processed at NEON headquarters. The entire dataset can be accessed by request from the NEON website.
This data download contains several files used in related tutorials. The path to the files we will be using in this tutorial is: NEON-DS-Field-Site-Spatial-Data/SJER/. You should set your working directory to the parent directory of the downloaded data to follow the code exactly.
Recommended Reading
What is Raster Data?
Raster or "gridded" data are data that are saved in pixels. In the spatial world, each pixel represents an area on the Earth's surface. For example in the raster below, each pixel represents a particular land cover class that would be found in that location in the real world.
More on rasters in the The Relationship Between Raster Resolution, Spatial extent & Number of Pixels tutorial.
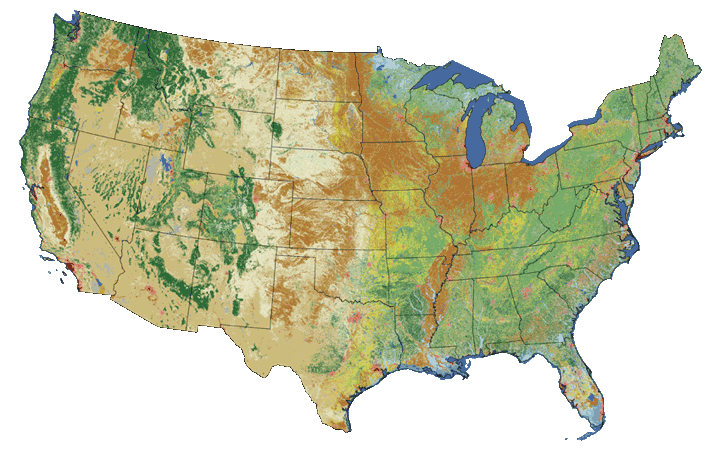
To work with rasters in R, we need two key packages, sp
and raster
.
To install the raster package you can use install.packages('raster')
.
When you install the raster package, sp
should also install. Also install the
rgdal
package install.packages('rgdal')
. Among other things, rgdal
will
allow us to export rasters to GeoTIFF format.
Once installed we can load the packages and start working with raster data.
# load the raster, sp, and rgdal packages
library(raster)
library(sp)
library(rgdal)
# set working directory to data folder
#setwd("pathToDirHere")
wd <- ("~/Git/data/")
setwd(wd)
Next, let's load a raster containing elevation data into our environment. And look at the attributes.
# load raster in an R object called 'DEM'
DEM <- raster(paste0(wd, "NEON-DS-Field-Site-Spatial-Data/SJER/DigitalTerrainModel/SJER2013_DTM.tif"))
# look at the raster attributes.
DEM
## class : RasterLayer
## dimensions : 5060, 4299, 21752940 (nrow, ncol, ncell)
## resolution : 1, 1 (x, y)
## extent : 254570, 258869, 4107302, 4112362 (xmin, xmax, ymin, ymax)
## crs : +proj=utm +zone=11 +datum=WGS84 +units=m +no_defs
## source : /Users/olearyd/Git/data/NEON-DS-Field-Site-Spatial-Data/SJER/DigitalTerrainModel/SJER2013_DTM.tif
## names : SJER2013_DTM
Notice a few things about this raster.
-
dimensions
: the "size" of the file in pixels
-
nrow
,ncol
: the number of rows and columns in the data (imagine a spreadsheet or a matrix). -
ncells
: the total number of pixels or cells that make up the raster.
-
resolution
: the size of each pixel (in meters in this case). 1 meter pixels means that each pixel represents a 1m x 1m area on the earth's surface. -
extent
: the spatial extent of the raster. This value will be in the same coordinate units as the coordinate reference system of the raster. -
coord ref
: the coordinate reference system string for the raster. This raster is in UTM (Universal Trans Mercator) zone 11 with a datum of WGS 84. More on UTM here.
Work with Rasters in R
Now that we have the raster loaded into R, let's grab some key raster attributes.
Define Min/Max Values
By default this raster doesn't have the min or max values associated with it's attributes
Let's change that by using the setMinMax()
function.
# calculate and save the min and max values of the raster to the raster object
DEM <- setMinMax(DEM)
# view raster attributes
DEM
## class : RasterLayer
## dimensions : 5060, 4299, 21752940 (nrow, ncol, ncell)
## resolution : 1, 1 (x, y)
## extent : 254570, 258869, 4107302, 4112362 (xmin, xmax, ymin, ymax)
## crs : +proj=utm +zone=11 +datum=WGS84 +units=m +no_defs
## source : /Users/olearyd/Git/data/NEON-DS-Field-Site-Spatial-Data/SJER/DigitalTerrainModel/SJER2013_DTM.tif
## names : SJER2013_DTM
## values : 228.1, 518.66 (min, max)
Notice the values
is now part of the attributes and shows the min and max values
for the pixels in the raster. What these min and max values represent depends on
what is represented by each pixel in the raster.
You can also view the rasters min and max values and the range of values contained within the pixels.
#Get min and max cell values from raster
#NOTE: this code may fail if the raster is too large
cellStats(DEM, min)
## [1] 228.1
cellStats(DEM, max)
## [1] 518.66
cellStats(DEM, range)
## [1] 228.10 518.66
View CRS
First, let's consider the Coordinate Reference System (CRS).
#view coordinate reference system
DEM@crs
## CRS arguments:
## +proj=utm +zone=11 +datum=WGS84 +units=m +no_defs
This raster is located in UTM Zone 11.
View Extent
If you want to know the exact boundaries of your raster that is in the extent
slot.
# view raster extent
DEM@extent
## class : Extent
## xmin : 254570
## xmax : 258869
## ymin : 4107302
## ymax : 4112362
Raster Pixel Values
We can also create a histogram to view the distribution of values in our raster.
Note that the max number of pixels that R will plot by default is 100,000. We
can tell it to plot more using the maxpixels
attribute. Be careful with this,
if your raster is large this can take a long time or crash your program.
Since our raster is a digital elevation model, we know that each pixel contains elevation data about our area of interest. In this case the units are meters.
This is an easy and quick data checking tool. Are there any totally weird values?
# the distribution of values in the raster
hist(DEM, main="Distribution of elevation values",
col= "purple",
maxpixels=22000000)
It looks like we have a lot of land around 325m and 425m.
Plot Raster Data
Let's take a look at our raster now that we know a bit more about it. We can do
a simple plot with the plot()
function.
# plot the raster
# note that this raster represents a small region of the NEON SJER field site
plot(DEM,
main="Digital Elevation Model, SJER") # add title with main
R has an image()
function that allows you to control the way a raster is
rendered on the screen. The plot()
function in R has a base setting for the number
of pixels that it will plot (100,000 pixels). The image command thus might be
better for rendering larger rasters.
# create a plot of our raster
image(DEM)
# specify the range of values that you want to plot in the DEM
# just plot pixels between 250 and 300 m in elevation
image(DEM, zlim=c(250,300))
# we can specify the colors too
col <- terrain.colors(5)
image(DEM, zlim=c(250,375), main="Digital Elevation Model (DEM)", col=col)
Plotting with Colors
In the above example. terrain.colors()
tells R to create a palette of colors
within the terrain.colors
color ramp. There are other palettes that you can
use as well include rainbow
and heat.colors
.
- More on color palettes in R here.
- Another good post on colors.
- RColorBrewer is another powerful tool to create sets of colors.
Explore colors more:
- What happens if you change the number of colors in the
terrain.colors()
function? - What happens if you change the
zlim
values in theimage()
function? - What are the other attributes that you can specify when using the
image()
function?
Breaks and Colorbars in R
A digital elevation model (DEM) is an example of a continuous raster. It contains elevation values for a range. For example, elevations values in a DEM might include any set of values between 200 m and 500 m. Given this range, you can plot DEM pixels using a gradient of colors.
By default, R will assign the gradient of colors uniformly across the full range of values in the data. In our case, our DEM has values between 250 and 500. However, we can adjust the "breaks" which represent the numeric locations where the colors change if we want too.
# add a color map with 5 colors
col=terrain.colors(5)
# add breaks to the colormap (6 breaks = 5 segments)
brk <- c(250, 300, 350, 400, 450, 500)
plot(DEM, col=col, breaks=brk, main="DEM with more breaks")
We can also customize the legend appearance.
# First, expand right side of clipping rectangle to make room for the legend
# turn xpd off
par(xpd = FALSE, mar=c(5.1, 4.1, 4.1, 4.5))
# Second, plot w/ no legend
plot(DEM, col=col, breaks=brk, main="DEM with a Custom (but flipped) Legend", legend = FALSE)
# Third, turn xpd back on to force the legend to fit next to the plot.
par(xpd = TRUE)
# Fourth, add a legend - & make it appear outside of the plot
legend(par()$usr[2], 4110600,
legend = c("lowest", "a bit higher", "middle ground", "higher yet", "highest"),
fill = col)
Notice that the legend is in reverse order in the previous plot. Let’s fix that.
We need to both reverse the order we have the legend laid out and reverse the
the color fill with the rev()
colors.
# Expand right side of clipping rect to make room for the legend
par(xpd = FALSE,mar=c(5.1, 4.1, 4.1, 4.5))
#DEM with a custom legend
plot(DEM, col=col, breaks=brk, main="DEM with a Custom Legend",legend = FALSE)
#turn xpd back on to force the legend to fit next to the plot.
par(xpd = TRUE)
#add a legend - but make it appear outside of the plot
legend( par()$usr[2], 4110600,
legend = c("Highest", "Higher yet", "Middle","A bit higher", "Lowest"),
fill = rev(col))
Try the code again but only make one of the changes -- reverse order or reverse colors-- what happens?
The raster plot now inverts the elevations! This is a good reason to understand your data so that a simple visualization error doesn't have you reversing the slope or some other error.
We can add a custom color map with fewer breaks as well.
#add a color map with 4 colors
col=terrain.colors(4)
#add breaks to the colormap (6 breaks = 5 segments)
brk <- c(200, 300, 350, 400,500)
plot(DEM, col=col, breaks=brk, main="DEM with fewer breaks")
A discrete dataset has a set of unique categories or classes. One example could be land use classes. The example below shows elevation zones generated using the same DEM.
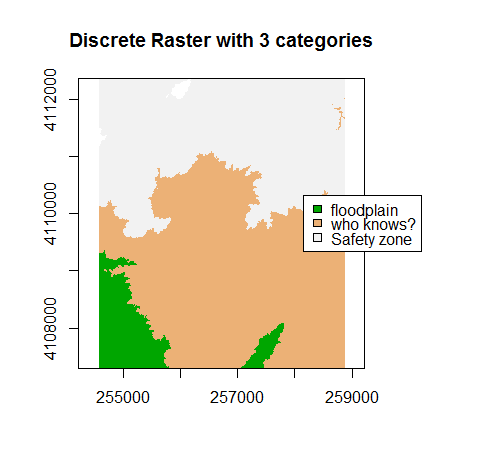
Basic Raster Math
We can also perform calculations on our raster. For instance, we could multiply all values within the raster by 2.
#multiple each pixel in the raster by 2
DEM2 <- DEM * 2
DEM2
## class : RasterLayer
## dimensions : 5060, 4299, 21752940 (nrow, ncol, ncell)
## resolution : 1, 1 (x, y)
## extent : 254570, 258869, 4107302, 4112362 (xmin, xmax, ymin, ymax)
## crs : +proj=utm +zone=11 +datum=WGS84 +units=m +no_defs
## source : memory
## names : SJER2013_DTM
## values : 456.2, 1037.32 (min, max)
#plot the new DEM
plot(DEM2, main="DEM with all values doubled")
Cropping Rasters in R
You can crop rasters in R using different methods. You can crop the raster directly drawing a box in the plot area. To do this, first plot the raster. Then define the crop extent by clicking twice:
- Click in the UPPER LEFT hand corner where you want the crop box to begin.
- Click again in the LOWER RIGHT hand corner to define where the box ends.
You'll see a red box on the plot. NOTE that this is a manual process that can be used to quickly define a crop extent.
#plot the DEM
plot(DEM)
#Define the extent of the crop by clicking on the plot
cropbox1 <- drawExtent()
#crop the raster, then plot the new cropped raster
DEMcrop1 <- crop(DEM, cropbox1)
#plot the cropped extent
plot(DEMcrop1)
You can also manually assign the extent coordinates to be used to crop a raster.
We'll need the extent defined as (xmin
, xmax
, ymin
, ymax
) to do this.
This is how we'd crop using a GIS shapefile (with a rectangular shape)
#define the crop extent
cropbox2 <-c(255077.3,257158.6,4109614,4110934)
#crop the raster
DEMcrop2 <- crop(DEM, cropbox2)
#plot cropped DEM
plot(DEMcrop2)
Use what you've learned to open and plot a Digital Surface Model.
- Create an R object called
DSM
from the raster:DigitalSurfaceModel/SJER2013_DSM.tif
. - Convert the raster data from m to feet. What is that conversion again? Oh, right 1m = ~3.3ft.
- Plot the
DSM
in feet using a custom color map. - Create numeric breaks that make sense given the distribution of the data.
Hint, your breaks might represent
high elevation
,medium elevation
,low elevation
.
Image (RGB) Data in R
Go to our tutorial Image Raster Data in R - An Intro to learn more about working with image formatted rasters in R.
The Relationship Between Raster Resolution, Spatial Extent & Number of Pixels
Learning Objectives:
After completing this activity, you will be able to:
- Explain the key attributes required to work with raster data including: spatial extent, coordinate reference system and spatial resolution.
- Describe what a spatial extent is and how it relates to resolution.
- Explain the basics of coordinate reference systems.
Things You’ll Need To Complete This Tutorial
You will need the most current version of R and, preferably, RStudio
loaded
on your computer to complete this tutorial.
Install R Packages
-
raster:
install.packages("raster")
-
rgdal:
install.packages("rgdal")
Data to Download
NEON Teaching Data Subset: Field Site Spatial Data
These remote sensing data files provide information on the vegetation at the National Ecological Observatory Network's San Joaquin Experimental Range and Soaproot Saddle field sites. The entire dataset can be accessed by request from the NEON Data Portal.
Download DatasetThe LiDAR and imagery data used to create the rasters in this dataset were collected over the San Joaquin field site located in California (NEON Domain 17) and processed at NEON headquarters. The entire dataset can be accessed by request from the NEON website.
This data download contains several files used in related tutorials. The path to
the files we will be using in this tutorial is:
NEON-DS-Field-Site-Spatial-Data/SJER/.
You should set your working directory to the parent directory of the downloaded
data to follow the code exactly.
This tutorial will overview the key attributes of a raster object, including
spatial extent, resolution and coordinate reference system. When working within
a GIS system often these attributes are accounted for. However, it is important
to be more familiar with them when working in non-GUI environments such as
R or even Python
.
In order to correctly spatially reference a raster that is not already georeferenced, you will also need to identify:
- The lower left hand corner coordinates of the raster.
- The number of columns and rows that the raster dataset contains.
Spatial Resolution
A raster consists of a series of pixels, each with the same dimensions and shape. In the case of rasters derived from airborne sensors, each pixel represents an area of space on the Earth's surface. The size of the area on the surface that each pixel covers is known as the spatial resolution of the image. For instance, an image that has a 1 m spatial resolution means that each pixel in the image represents a 1 m x 1 m area.
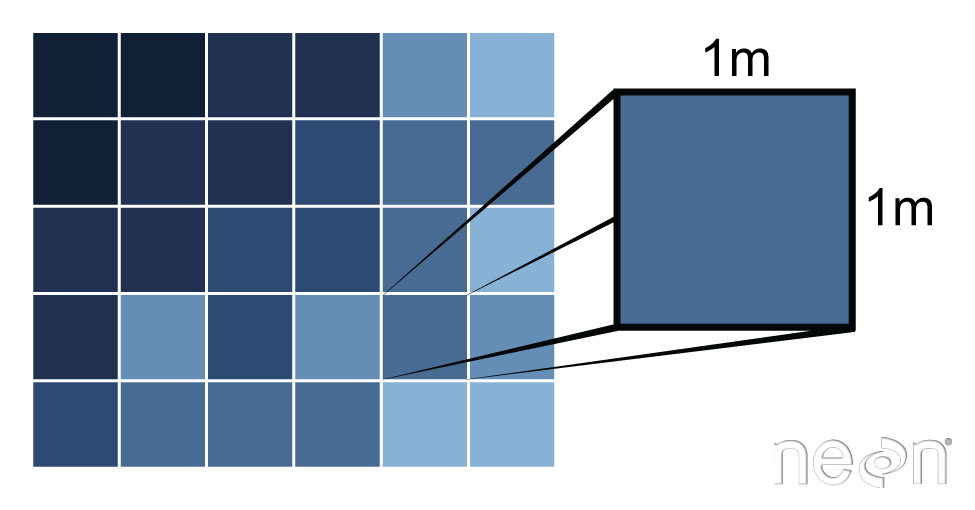
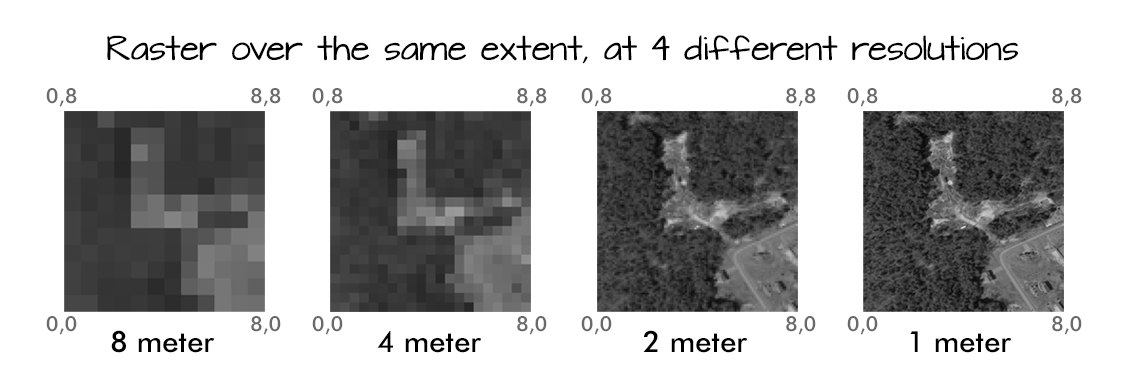
Load the Data
Let's open up a raster in R to see how the attributes are stored. We are going to work with a Digital Terrain Model from the San Joaquin Experimental Range in California.
# load packages
library(raster)
library(rgdal)
# set working directory to data folder
#setwd("pathToDirHere")
wd <- ("~/Git/data/")
setwd(wd)
# Load raster in an R object called 'DEM'
DEM <- raster(paste0(wd, "NEON-DS-Field-Site-Spatial-Data/SJER/DigitalTerrainModel/SJER2013_DTM.tif"))
# View raster attributes
DEM
## class : RasterLayer
## dimensions : 5060, 4299, 21752940 (nrow, ncol, ncell)
## resolution : 1, 1 (x, y)
## extent : 254570, 258869, 4107302, 4112362 (xmin, xmax, ymin, ymax)
## crs : +proj=utm +zone=11 +datum=WGS84 +units=m +no_defs
## source : /Users/olearyd/Git/data/NEON-DS-Field-Site-Spatial-Data/SJER/DigitalTerrainModel/SJER2013_DTM.tif
## names : SJER2013_DTM
Note that this raster (in GeoTIFF format) already has an extent, resolution, and CRS defined. The resolution in both x and y directions is 1. The CRS tells us that the x,y units of the data are meters (m).
Spatial Extent
The spatial extent of a raster, represents the "X, Y" coordinates of the corners
of the raster in geographic space. This information, in addition to the cell
size or spatial resolution, tells the program how to place or render each pixel
in 2 dimensional space. Tools like R, using supporting packages such as rgdal
and associated raster tools have functions that allow you to view and define the
extent of a new raster.
# View the extent of the raster
DEM@extent
## class : Extent
## xmin : 254570
## xmax : 258869
## ymin : 4107302
## ymax : 4112362
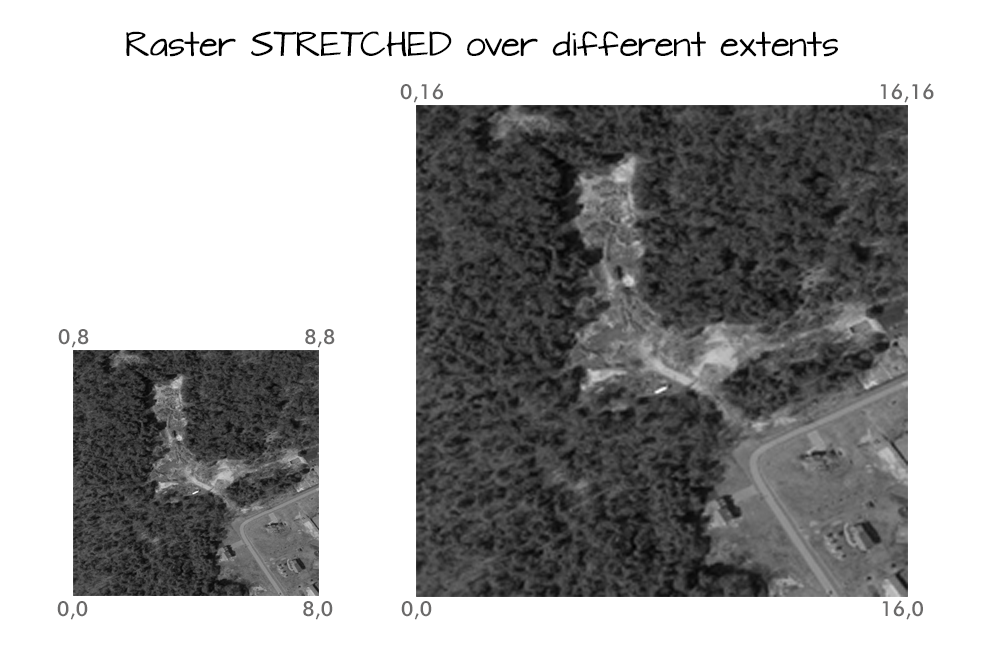
Calculating Raster Extent
Extent and spatial resolution are closely connected. To calculate the extent of a raster, we first need the bottom left hand (X,Y) coordinate of the raster. In the case of the UTM coordinate system which is in meters, to calculate the raster's extent, we can add the number of columns and rows to the X,Y corner coordinate location of the raster, multiplied by the resolution (the pixel size) of the raster.
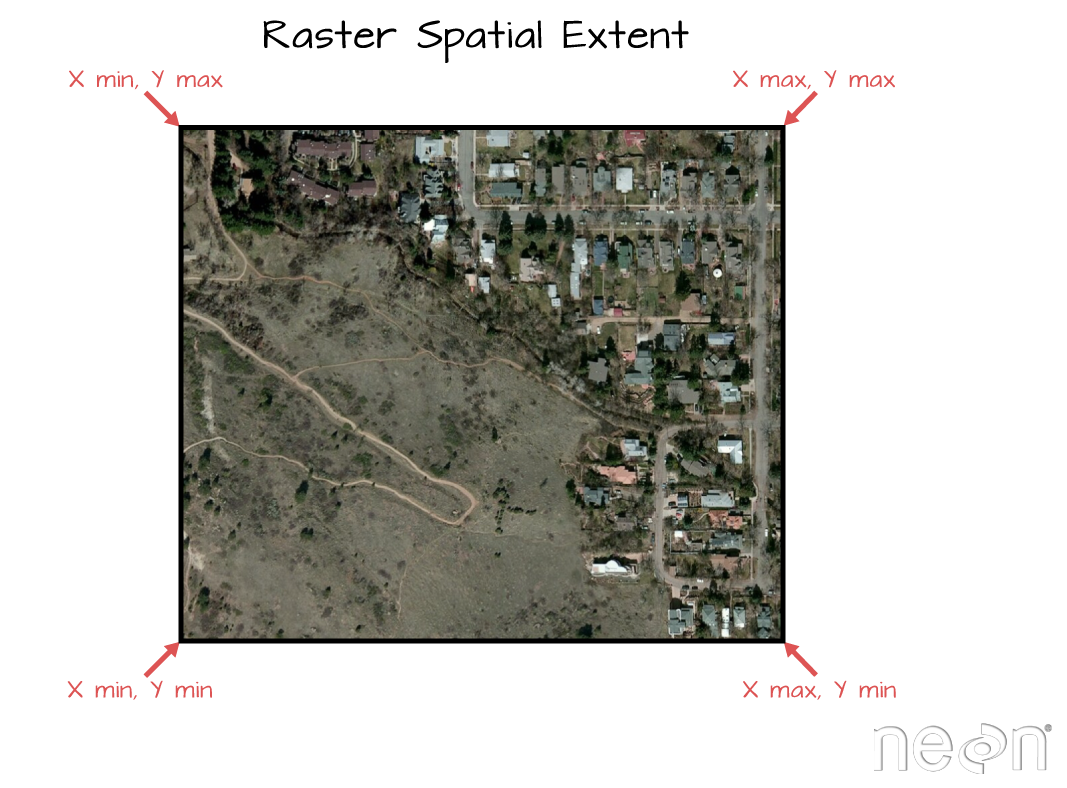
<figcaption>To be located geographically, a raster's location needs to be
defined in geographic space (i.e., on a spatial grid). The spatial extent
defines the four corners of a raster within a given coordinate reference
system. Source: National Ecological Observatory Network. </figcaption>
Let's explore that next, using a blank raster that we create.
# create a raster from the matrix - a "blank" raster of 4x4
myRaster1 <- raster(nrow=4, ncol=4)
# assign "data" to raster: 1 to n based on the number of cells in the raster
myRaster1[]<- 1:ncell(myRaster1)
# view attributes of the raster
myRaster1
## class : RasterLayer
## dimensions : 4, 4, 16 (nrow, ncol, ncell)
## resolution : 90, 45 (x, y)
## extent : -180, 180, -90, 90 (xmin, xmax, ymin, ymax)
## crs : +proj=longlat +datum=WGS84 +no_defs
## source : memory
## names : layer
## values : 1, 16 (min, max)
# is the CRS defined?
myRaster1@crs
## CRS arguments: +proj=longlat +datum=WGS84 +no_defs
Wait, why is the CRS defined on this new raster? This is the default values
for something created with the raster()
function if nothing is defined.
Let's get back to looking at more attributes.
# what is the raster extent?
myRaster1@extent
## class : Extent
## xmin : -180
## xmax : 180
## ymin : -90
## ymax : 90
# plot raster
plot(myRaster1, main="Raster with 16 pixels")
Here we see our raster with the value of 1 to 16 in each pixel.
We can resample the raster as well to adjust the resolution. If we want a higher resolution raster, we will apply a grid with more pixels within the same extent. If we want a lower resolution raster, we will apply a grid with fewer pixels within the same extent.
One way to do this is to create a raster of the resolution you want and then
resample()
your original raster. The resampling will be done for either
nearest neighbor assignments (for categorical data) or bilinear interpolation (for
numerical data).
## HIGHER RESOLUTION
# Create 32 pixel raster
myRaster2 <- raster(nrow=8, ncol=8)
# resample 16 pix raster with 32 pix raster
# use bilinear interpolation with our numeric data
myRaster2 <- resample(myRaster1, myRaster2, method='bilinear')
# notice new dimensions, resolution, & min/max
myRaster2
## class : RasterLayer
## dimensions : 8, 8, 64 (nrow, ncol, ncell)
## resolution : 45, 22.5 (x, y)
## extent : -180, 180, -90, 90 (xmin, xmax, ymin, ymax)
## crs : +proj=longlat +datum=WGS84 +no_defs
## source : memory
## names : layer
## values : -0.25, 17.25 (min, max)
# plot
plot(myRaster2, main="Raster with 32 pixels")
## LOWER RESOLUTION
myRaster3 <- raster(nrow=2, ncol=2)
myRaster3 <- resample(myRaster1, myRaster3, method='bilinear')
myRaster3
## class : RasterLayer
## dimensions : 2, 2, 4 (nrow, ncol, ncell)
## resolution : 180, 90 (x, y)
## extent : -180, 180, -90, 90 (xmin, xmax, ymin, ymax)
## crs : +proj=longlat +datum=WGS84 +no_defs
## source : memory
## names : layer
## values : 3.5, 13.5 (min, max)
plot(myRaster3, main="Raster with 4 pixels")
## SINGLE PIXEL RASTER
myRaster4 <- raster(nrow=1, ncol=1)
myRaster4 <- resample(myRaster1, myRaster4, method='bilinear')
myRaster4
## class : RasterLayer
## dimensions : 1, 1, 1 (nrow, ncol, ncell)
## resolution : 360, 180 (x, y)
## extent : -180, 180, -90, 90 (xmin, xmax, ymin, ymax)
## crs : +proj=longlat +datum=WGS84 +no_defs
## source : memory
## names : layer
## values : 7.666667, 7.666667 (min, max)
plot(myRaster4, main="Raster with 1 pixel")
To more easily compare them, let's create a graphic layout with 4 rasters in it. Notice that each raster has the same extent but each a different resolution because it has a different number of pixels spread out over the same extent.
# change graphical parameter to 2x2 grid
par(mfrow=c(2,2))
# arrange plots in order you wish to see them
plot(myRaster2, main="Raster with 32 pixels")
plot(myRaster1, main="Raster with 16 pixels")
plot(myRaster3, main="Raster with 4 pixels")
plot(myRaster4, main="Raster with 2 pixels")
# change graphical parameter back to 1x1
par(mfrow=c(1,1))
Extent & Coordinate Reference Systems
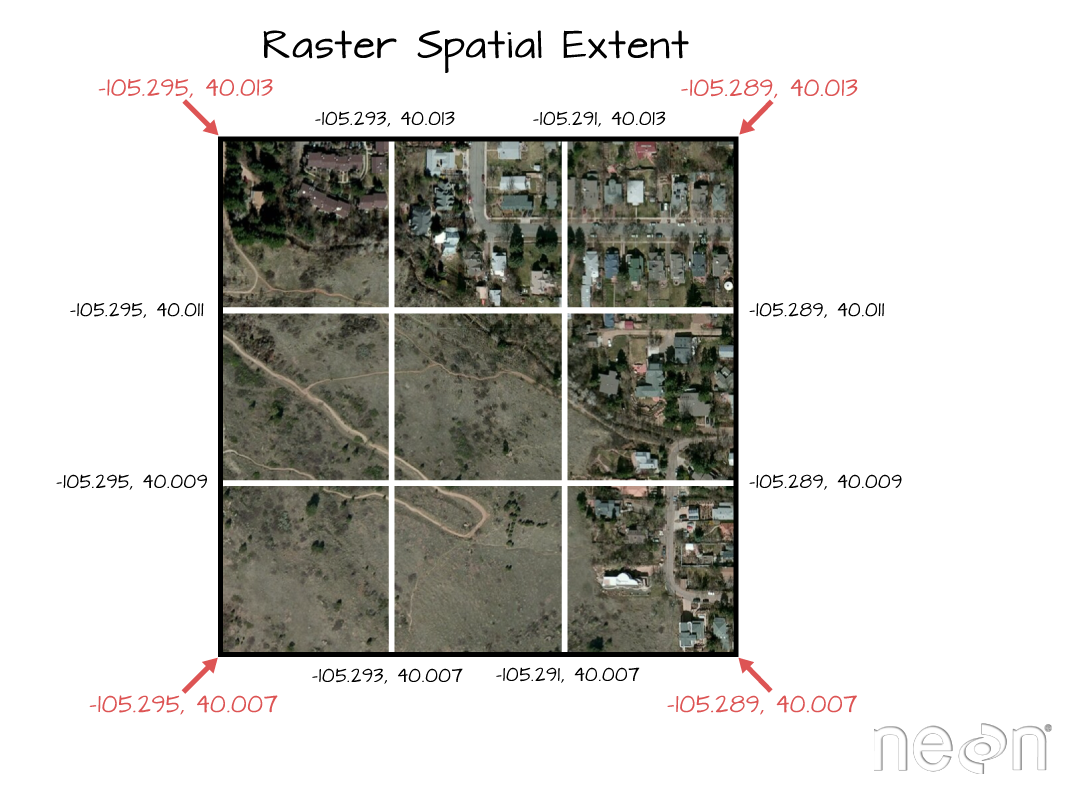
Coordinate Reference System & Projection Information
A spatial reference system (SRS) or coordinate reference system (CRS) is a coordinate-based local, regional or global system used to locate geographical entities. -- Wikipedia
The earth is round. This is not an new concept by any means, however we need to remember this when we talk about coordinate reference systems associated with spatial data. When we make maps on paper or on a computer screen, we are moving from a 3 dimensional space (the globe) to 2 dimensions (our computer screens or a piece of paper). To keep this short, the projection of a dataset relates to how the data are "flattened" in geographic space so our human eyes and brains can make sense of the information in 2 dimensions.
The projection refers to the mathematical calculations performed to "flatten the data" in into 2D space. The coordinate system references to the x and y coordinate space that is associated with the projection used to flatten the data. If you have the same dataset saved in two different projections, these two files won't line up correctly when rendered together.
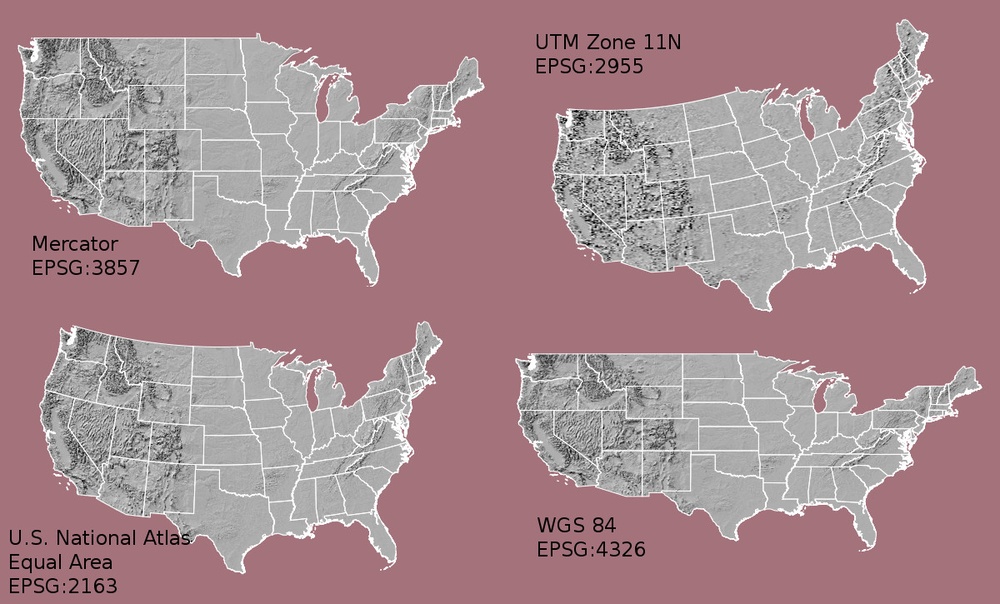
How Map Projections Can Fool the Eye
Check out this short video, by Buzzfeed, highlighting how map projections can make continents seems proportionally larger or smaller than they actually are!
What Makes Spatial Data Line Up On A Map?
There are lots of great resources that describe coordinate reference systems and
projections in greater detail. However, for the purposes of this activity, what
is important to understand is that data from the same location but saved in
different projections will not line up in any GIS or other program. Thus
it's important when working with spatial data in a program like R or Python
to identify the coordinate reference system applied to the data, and to grab
that information and retain it when you process / analyze the data.
For a library of CRS information: A great online library of CRS information.
CRS proj4 Strings
The rgdal package has all the common ESPG codes with proj4string built in. We
can see them by creating an object of the function make_ESPG()
.
# make sure you loaded rgdal package at the top of your script
# create an object with all ESPG codes
epsg = make_EPSG()
# use View(espg) to see the full table - doesn't render on website well
#View(epsg)
# View top 5 entries
head(epsg, 5)
## code note prj4
## 1 3819 HD1909 +proj=longlat +ellps=bessel +no_defs +type=crs
## 2 3821 TWD67 +proj=longlat +ellps=aust_SA +no_defs +type=crs
## 3 3822 TWD97 +proj=geocent +ellps=GRS80 +units=m +no_defs +type=crs
## 4 3823 TWD97 +proj=longlat +ellps=GRS80 +no_defs +type=crs
## 5 3824 TWD97 +proj=longlat +ellps=GRS80 +no_defs +type=crs
## prj_method
## 1 (null)
## 2 (null)
## 3 (null)
## 4 (null)
## 5 (null)
Define the extent
In the above raster example, we created several simple raster objects in R. R defaulted to a global lat/long extent. We can define the exact extent that we need to use too.
Let's create a new raster with the same projection as our original DEM. We know that our data are in UTM zone 11N. For the sake of this exercise, let say we want to create a raster with the left hand corner coordinate at:
- xmin = 254570
- ymin = 4107302
The resolution of this new raster will be 1 meter
and we will be working
in UTM (meters). First, let's set up the raster.
# create 10x20 matrix with values 1-8.
newMatrix <- (matrix(1:8, nrow = 10, ncol = 20))
# convert to raster
rasterNoProj <- raster(newMatrix)
rasterNoProj
## class : RasterLayer
## dimensions : 10, 20, 200 (nrow, ncol, ncell)
## resolution : 0.05, 0.1 (x, y)
## extent : 0, 1, 0, 1 (xmin, xmax, ymin, ymax)
## crs : NA
## source : memory
## names : layer
## values : 1, 8 (min, max)
Now we can define the new raster's extent by defining the lower left corner of the raster.
## Define the xmin and ymin (the lower left hand corner of the raster)
# 1. define xMin & yMin objects.
xMin = 254570
yMin = 4107302
# 2. grab the cols and rows for the raster using @ncols and @nrows
rasterNoProj@ncols
## [1] 20
rasterNoProj@nrows
## [1] 10
# 3. raster resolution
res <- 1.0
# 4. add the numbers of cols and rows to the x,y corner location,
# result = we get the bounds of our raster extent.
xMax <- xMin + (rasterNoProj@ncols * res)
yMax <- yMin + (rasterNoProj@nrows * res)
# 5.create a raster extent class
rasExt <- extent(xMin,xMax,yMin,yMax)
rasExt
## class : Extent
## xmin : 254570
## xmax : 254590
## ymin : 4107302
## ymax : 4107312
# 6. apply the extent to our raster
rasterNoProj@extent <- rasExt
# Did it work?
rasterNoProj
## class : RasterLayer
## dimensions : 10, 20, 200 (nrow, ncol, ncell)
## resolution : 1, 1 (x, y)
## extent : 254570, 254590, 4107302, 4107312 (xmin, xmax, ymin, ymax)
## crs : NA
## source : memory
## names : layer
## values : 1, 8 (min, max)
# or view extent only
rasterNoProj@extent
## class : Extent
## xmin : 254570
## xmax : 254590
## ymin : 4107302
## ymax : 4107312
Now we have an extent associated with our raster which places it in space!
# plot new raster
plot(rasterNoProj, main="Raster in UTM coordinates, 1 m resolution")
Notice that the coordinates show up on our plot now.
Now apply your skills in a new way!
- Resample
rasterNoProj
from 1 meter to 10 meter resolution. Plot it next to the 1 m resolution raster. Use:par(mfrow=c(1,2))
to create side by side plots. - What happens to the extent if you change the resolution to 1.5 when calculating the raster's extent properties??
Define Projection of a Raster
We can define the projection of a raster that has a known CRS already. Sometimes we download data that have projection information associated with them but the CRS is not defined either in the GeoTIFF tags or in the raster itself. If this is the case, we can simply assign the raster the correct projection.
Be careful doing this - it is not the same thing as reprojecting your data.
Let's define the projection for our newest raster using the DEM raster that already has defined CRS. NOTE: in this case we have to know that our raster is in this projection already so we don't run the risk of assigning the wrong projection to the data.
# view CRS from raster of interest
rasterNoProj@crs
## CRS arguments: NA
# view the CRS of our DEM object.
DEM@crs
## CRS arguments:
## +proj=utm +zone=11 +datum=WGS84 +units=m +no_defs
# define the CRS using a CRS of another raster
rasterNoProj@crs <- DEM@crs
# look at the attributes
rasterNoProj
## class : RasterLayer
## dimensions : 10, 20, 200 (nrow, ncol, ncell)
## resolution : 1, 1 (x, y)
## extent : 254570, 254590, 4107302, 4107312 (xmin, xmax, ymin, ymax)
## crs : +proj=utm +zone=11 +datum=WGS84 +units=m +no_defs
## source : memory
## names : layer
## values : 1, 8 (min, max)
# view just the crs
rasterNoProj@crs
## CRS arguments:
## +proj=utm +zone=11 +datum=WGS84 +units=m +no_defs
IMPORTANT: the above code does not reproject the raster. It simply defines the
Coordinate Reference System based upon the CRS of another raster. If you want to
actually change the CRS of a raster, you need to use the projectRaster
function.
You can set the CRS and extent of a raster using the syntax
rasterWithoutReference@crs <- rasterWithReference@crs
and
rasterWithoutReference@extent <- rasterWithReference@extent
. Using this information:
- open
band90.tif
in therasterLayers_tif
folder and plot it. (You could consider looking at it in QGIS first to compare it to the other rasters.) - Does it line up with our DEM? Look closely at the extent and pixel size. Does anything look off?
- Fix what is missing.
- (Advanced step) Export a new GeoTIFF Do things line up in QGIS?
The code below creates a raster and seeds it with some data. Experiment with the code.
- What happens to the resulting raster's resolution when you change the range of lat and long values to 5 instead of 10? Try 20, 50 and 100?
- What is the relationship between the extent and the raster resolution?
## Challenge Example Code
# set latLong
latLong <- data.frame(longitude=seq( 0,10,1), latitude=seq( 0,10,1))
# make spatial points dataframe, which will have a spatial extent
sp <- SpatialPoints( latLong[ c("longitude" , "latitude") ], proj4string = CRS("+proj=longlat +datum=WGS84") )
# make raster based on the extent of your data
r <- raster(nrow=5, ncol=5, extent( sp ) )
r[] <- 1
r[] <- sample(0:50,25)
r
## class : RasterLayer
## dimensions : 5, 5, 25 (nrow, ncol, ncell)
## resolution : 2, 2 (x, y)
## extent : 0, 10, 0, 10 (xmin, xmax, ymin, ymax)
## crs : NA
## source : memory
## names : layer
## values : 3, 50 (min, max)
Reprojecting Data
If you run into multiple spatial datasets with varying projections, you can always reproject the data so that they are all in the same projection. Python and R both have reprojection tools that perform this task.
# reproject raster data from UTM to CRS of Lat/Long WGS84
reprojectedData1 <- projectRaster(rasterNoProj,
crs="+proj=longlat +ellps=WGS84 +datum=WGS84 +no_defs ")
# note that the extent has been adjusted to account for the NEW crs
reprojectedData1@crs
## CRS arguments: +proj=longlat +datum=WGS84 +no_defs
reprojectedData1@extent
## class : Extent
## xmin : -119.761
## xmax : -119.7607
## ymin : 37.07988
## ymax : 37.08
# note the range of values in the output data
reprojectedData1
## class : RasterLayer
## dimensions : 13, 22, 286 (nrow, ncol, ncell)
## resolution : 1.12e-05, 9e-06 (x, y)
## extent : -119.761, -119.7607, 37.07988, 37.08 (xmin, xmax, ymin, ymax)
## crs : +proj=longlat +datum=WGS84 +no_defs
## source : memory
## names : layer
## values : 0.64765, 8.641957 (min, max)
# use nearest neighbor interpolation method to ensure that the values stay the same
reprojectedData2 <- projectRaster(rasterNoProj,
crs="+proj=longlat +ellps=WGS84 +datum=WGS84 +no_defs ",
method = "ngb")
# note that the min and max values have now been forced to stay within the same range.
reprojectedData2
## class : RasterLayer
## dimensions : 13, 22, 286 (nrow, ncol, ncell)
## resolution : 1.12e-05, 9e-06 (x, y)
## extent : -119.761, -119.7607, 37.07988, 37.08 (xmin, xmax, ymin, ymax)
## crs : +proj=longlat +datum=WGS84 +no_defs
## source : memory
## names : layer
## values : 1, 8 (min, max)
Create a Canopy Height Model from Lidar-derived rasters in R
A common analysis using lidar data are to derive top of the canopy height values from the lidar data. These values are often used to track changes in forest structure over time, to calculate biomass, and even leaf area index (LAI). Let's dive into the basics of working with raster formatted lidar data in R!
Learning Objectives
After completing this tutorial, you will be able to:
- Work with digital terrain model (DTM) & digital surface model (DSM) raster files.
- Create a canopy height model (CHM) raster from DTM & DSM rasters.
Things You’ll Need To Complete This Tutorial
You will need the most current version of R and, preferably, RStudio
loaded
on your computer to complete this tutorial.
Install R Packages
-
terra:
install.packages("terra")
-
neonUtilities:
install.packages("neonUtilities")
More on Packages in R - Adapted from Software Carpentry.
Download Data
Lidar elevation raster data are downloaded using the R neonUtilities::byTileAOP
function in the script.
These remote sensing data files provide information on the vegetation at the National Ecological Observatory Network's San Joaquin Experimental Range and Soaproot Saddle field sites. The entire datasets can be accessed from the NEON Data Portal.
This tutorial is designed for you to set your working directory to the directory created by unzipping this file.
Set Working Directory: This lesson will walk you through setting the working directory before downloading the datasets from neonUtilities.
An overview of setting the working directory in R can be found here.
R Script & Challenge Code: NEON data lessons often contain challenges to reinforce skills. If available, the code for challenge solutions is found in the downloadable R script of the entire lesson, available in the footer of each lesson page.
Recommended Reading
What is a CHM, DSM and DTM? About Gridded, Raster LiDAR DataCreate a lidar-derived Canopy Height Model (CHM)
The National Ecological Observatory Network (NEON) will provide lidar-derived data products as one of its many free ecological data products. These products will come in the GeoTIFF format, which is a .tif raster format that is spatially located on the earth.
In this tutorial, we create a Canopy Height Model. The Canopy Height Model (CHM), represents the heights of the trees on the ground. We can derive the CHM by subtracting the ground elevation from the elevation of the top of the surface (or the tops of the trees).
We will use the terra
R package to work with the the lidar-derived Digital
Surface Model (DSM) and the Digital Terrain Model (DTM).
# Load needed packages
library(terra)
library(neonUtilities)
Set the working directory so you know where to download data.
wd="~/data/" #This will depend on your local environment
setwd(wd)
We can use the neonUtilities
function byTileAOP
to download a single DTM and DSM tile at SJER. Both the DTM and DSM are delivered under the Elevation - LiDAR (DP3.30024.001) data product.
You can run help(byTileAOP)
to see more details on what the various inputs are. For this exercise, we'll specify the UTM Easting and Northing to be (257500, 4112500), which will download the tile with the lower left corner (257000,4112000). By default, the function will check the size total size of the download and ask you whether you wish to proceed (y/n). You can set check.size=FALSE
if you want to download without a prompt. This example will not be very large (~8MB), since it is only downloading two single-band rasters (plus some associated metadata).
byTileAOP(dpID='DP3.30024.001',
site='SJER',
year='2021',
easting=257500,
northing=4112500,
check.size=TRUE, # set to FALSE if you don't want to enter y/n
savepath = wd)
This file will be downloaded into a nested subdirectory under the ~/data
folder, inside a folder named DP3.30024.001
(the Data Product ID). The files should show up in these locations: ~/data/DP3.30024.001/neon-aop-products/2021/FullSite/D17/2021_SJER_5/L3/DiscreteLidar/DSMGtif/NEON_D17_SJER_DP3_257000_4112000_DSM.tif
and ~/data/DP3.30024.001/neon-aop-products/2021/FullSite/D17/2021_SJER_5/L3/DiscreteLidar/DTMGtif/NEON_D17_SJER_DP3_257000_4112000_DTM.tif
.
Now we can read in the files. You can move the files to a different location (eg. shorten the path), but make sure to change the path that points to the file accordingly.
# Define the DSM and DTM file names, including the full path
dsm_file <- paste0(wd,"DP3.30024.001/neon-aop-products/2021/FullSite/D17/2021_SJER_5/L3/DiscreteLidar/DSMGtif/NEON_D17_SJER_DP3_257000_4112000_DSM.tif")
dtm_file <- paste0(wd,"DP3.30024.001/neon-aop-products/2021/FullSite/D17/2021_SJER_5/L3/DiscreteLidar/DTMGtif/NEON_D17_SJER_DP3_257000_4112000_DTM.tif")
First, we will read in the Digital Surface Model (DSM). The DSM represents the elevation of the top of the objects on the ground (trees, buildings, etc).
# assign raster to object
dsm <- rast(dsm_file)
# view info about the raster.
dsm
## class : SpatRaster
## dimensions : 1000, 1000, 1 (nrow, ncol, nlyr)
## resolution : 1, 1 (x, y)
## extent : 257000, 258000, 4112000, 4113000 (xmin, xmax, ymin, ymax)
## coord. ref. : WGS 84 / UTM zone 11N (EPSG:32611)
## source : NEON_D17_SJER_DP3_257000_4112000_DSM.tif
## name : NEON_D17_SJER_DP3_257000_4112000_DSM
# plot the DSM
plot(dsm, main="Lidar Digital Surface Model \n SJER, California")
Note the resolution, extent, and coordinate reference system (CRS) of the raster. To do later steps, our DTM will need to be the same.
Next, we will import the Digital Terrain Model (DTM) for the same area. The DTM represents the ground (terrain) elevation.
# import the digital terrain model
dtm <- rast(dtm_file)
plot(dtm, main="Lidar Digital Terrain Model \n SJER, California")
With both of these rasters now loaded, we can create the Canopy Height Model (CHM). The CHM represents the difference between the DSM and the DTM or the height of all objects on the surface of the earth.
To do this we perform some basic raster math to calculate the CHM. You can perform the same raster math in a GIS program like QGIS.
When you do the math, make sure to subtract the DTM from the DSM or you'll get trees with negative heights!
# use raster math to create CHM
chm <- dsm - dtm
# view CHM attributes
chm
## class : SpatRaster
## dimensions : 1000, 1000, 1 (nrow, ncol, nlyr)
## resolution : 1, 1 (x, y)
## extent : 257000, 258000, 4112000, 4113000 (xmin, xmax, ymin, ymax)
## coord. ref. : WGS 84 / UTM zone 11N (EPSG:32611)
## source(s) : memory
## varname : NEON_D17_SJER_DP3_257000_4112000_DSM
## name : NEON_D17_SJER_DP3_257000_4112000_DSM
## min value : 0.00
## max value : 24.13
plot(chm, main="Lidar CHM - SJER, California")
We've now created a CHM from our DSM and DTM. What do you notice about the canopy cover at this location in the San Joaquin Experimental Range?
Challenge: Basic Raster Math
Convert the CHM from meters to feet and plot it.
We can write out the CHM as a GeoTiff using the writeRaster()
function.
# write out the CHM in tiff format.
writeRaster(chm,paste0(wd,"CHM_SJER.tif"),"GTiff")
We've now successfully created a canopy height model using basic raster math -- in
R! We can bring the CHM_SJER.tif
file into QGIS (or any GIS program) and look
at it.
Consider checking out the tutorial Compare tree height measured from the ground to a Lidar-based Canopy Height Model to compare a LiDAR-derived CHM with ground-based observations!
Introduction to HDF5 Files in R
Learning Objectives
After completing this tutorial, you will be able to:
- Understand how HDF5 files can be created and structured in R using the rhdf5 libraries.
- Understand the three key HDF5 elements: the HDF5 file itself, groups, and datasets.
- Understand how to add and read attributes from an HDF5 file.
Things You’ll Need To Complete This Tutorial
To complete this tutorial you will need the most current version of R and, preferably, RStudio loaded on your computer.
R Libraries to Install:
- rhdf5: The rhdf5 package is hosted on Bioconductor not CRAN. Directions for installation are in the first code chunk.
More on Packages in R – Adapted from Software Carpentry.
Data to Download
We will use the file below in the optional challenge activity at the end of this tutorial.
NEON Teaching Data Subset: Field Site Spatial Data
These remote sensing data files provide information on the vegetation at the National Ecological Observatory Network's San Joaquin Experimental Range and Soaproot Saddle field sites. The entire dataset can be accessed by request from the NEON Data Portal.
Download DatasetSet Working Directory: This lesson assumes that you have set your working directory to the location of the downloaded and unzipped data subsets.
An overview of setting the working directory in R can be found here.
R Script & Challenge Code: NEON data lessons often contain challenges that reinforce learned skills. If available, the code for challenge solutions is found in the downloadable R script of the entire lesson, available in the footer of each lesson page.
Additional Resources
Consider reviewing the documentation for the RHDF5 package.
About HDF5
The HDF5 file can store large, heterogeneous datasets that include metadata. It
also supports efficient data slicing
, or extraction of particular subsets of a
dataset which means that you don't have to read large files read into the
computers memory / RAM in their entirety in order work with them.
HDF5 in R
To access HDF5 files in R, we will use the rhdf5
library which is part of
the Bioconductor
suite of R libraries. It might also be useful to install
the
free HDF5 viewer
which will allow you to explore the contents of an HDF5 file using a graphic interface.
More about working with HDFview and a hands-on activity here.
First, let's get R setup. We will use the rhdf5 library. To access HDF5 files in R, we will use the rhdf5 library which is part of the Bioconductor suite of R packages. As of May 2020 this package was not yet on CRAN.
# Install rhdf5 package (only need to run if not already installed)
#install.packages("BiocManager")
#BiocManager::install("rhdf5")
# Call the R HDF5 Library
library("rhdf5")
# set working directory to ensure R can find the file we wish to import and where
# we want to save our files
wd <- "~/Git/data/" #This will depend on your local environment
setwd(wd)
Read more about the
rhdf5
package here.
Create an HDF5 File in R
Now, let's create a basic H5 file with one group and one dataset in it.
# Create hdf5 file
h5createFile("vegData.h5")
## [1] TRUE
# create a group called aNEONSite within the H5 file
h5createGroup("vegData.h5", "aNEONSite")
## [1] TRUE
# view the structure of the h5 we've created
h5ls("vegData.h5")
## group name otype dclass dim
## 0 / aNEONSite H5I_GROUP
Next, let's create some dummy data to add to our H5 file.
# create some sample, numeric data
a <- rnorm(n=40, m=1, sd=1)
someData <- matrix(a,nrow=20,ncol=2)
Write the sample data to the H5 file.
# add some sample data to the H5 file located in the aNEONSite group
# we'll call the dataset "temperature"
h5write(someData, file = "vegData.h5", name="aNEONSite/temperature")
# let's check out the H5 structure again
h5ls("vegData.h5")
## group name otype dclass dim
## 0 / aNEONSite H5I_GROUP
## 1 /aNEONSite temperature H5I_DATASET FLOAT 20 x 2
View a "dump" of the entire HDF5 file. NOTE: use this command with CAUTION if you are working with larger datasets!
# we can look at everything too
# but be cautious using this command!
h5dump("vegData.h5")
## $aNEONSite
## $aNEONSite$temperature
## [,1] [,2]
## [1,] 0.33155432 2.4054446
## [2,] 1.14305151 1.3329978
## [3,] -0.57253964 0.5915846
## [4,] 2.82950139 0.4669748
## [5,] 0.47549005 1.5871517
## [6,] -0.04144519 1.9470377
## [7,] 0.63300177 1.9532294
## [8,] -0.08666231 0.6942748
## [9,] -0.90739256 3.7809783
## [10,] 1.84223101 1.3364965
## [11,] 2.04727590 1.8736805
## [12,] 0.33825921 3.4941913
## [13,] 1.80738042 0.5766373
## [14,] 1.26130759 2.2307994
## [15,] 0.52882731 1.6021497
## [16,] 1.59861449 0.8514808
## [17,] 1.42037674 1.0989390
## [18,] -0.65366487 2.5783750
## [19,] 1.74865593 1.6069304
## [20,] -0.38986048 -1.9471878
# Close the file. This is good practice.
H5close()
Add Metadata (attributes)
Let's add some metadata (called attributes in HDF5 land) to our dummy temperature data. First, open up the file.
# open the file, create a class
fid <- H5Fopen("vegData.h5")
# open up the dataset to add attributes to, as a class
did <- H5Dopen(fid, "aNEONSite/temperature")
# Provide the NAME and the ATTR (what the attribute says) for the attribute.
h5writeAttribute(did, attr="Here is a description of the data",
name="Description")
h5writeAttribute(did, attr="Meters",
name="Units")
Now we can add some attributes to the file.
# let's add some attributes to the group
did2 <- H5Gopen(fid, "aNEONSite/")
h5writeAttribute(did2, attr="San Joaquin Experimental Range",
name="SiteName")
h5writeAttribute(did2, attr="Southern California",
name="Location")
# close the files, groups and the dataset when you're done writing to them!
H5Dclose(did)
H5Gclose(did2)
H5Fclose(fid)
Working with an HDF5 File in R
Now that we've created our H5 file, let's use it! First, let's have a look at the attributes of the dataset and group in the file.
# look at the attributes of the precip_data dataset
h5readAttributes(file = "vegData.h5",
name = "aNEONSite/temperature")
## $Description
## [1] "Here is a description of the data"
##
## $Units
## [1] "Meters"
# look at the attributes of the aNEONsite group
h5readAttributes(file = "vegData.h5",
name = "aNEONSite")
## $Location
## [1] "Southern California"
##
## $SiteName
## [1] "San Joaquin Experimental Range"
# let's grab some data from the H5 file
testSubset <- h5read(file = "vegData.h5",
name = "aNEONSite/temperature")
testSubset2 <- h5read(file = "vegData.h5",
name = "aNEONSite/temperature",
index=list(NULL,1))
H5close()
Once we've extracted data from our H5 file, we can work with it in R.
# create a quick plot of the data
hist(testSubset2)
Time to practice the skills you've learned. Open up the D17_2013_SJER_vegStr.csv in R.
- Create a new HDF5 file called
vegStructure
. - Add a group in your HDF5 file called
SJER
. - Add the veg structure data to that folder.
- Add some attributes the SJER group and to the data.
- Now, repeat the above with the D17_2013_SOAP_vegStr csv.
- Name your second group SOAP
Hint: read.csv()
is a good way to read in .csv files.
Intro to Working with Hyperspectral Remote Sensing Data in HDF5 Format in R
In this tutorial, we will show how to read and extract NEON reflectance data stored within an HDF5 file using R.
Learning Objectives
After completing this tutorial, you will be able to:
- Explain how HDF5 data can be used to store spatial data and the associated benefits of this format when working with large spatial data cubes.
- Extract metadata from HDF5 files.
- Slice or subset HDF5 data. You will extract one band of pixels.
- Plot a matrix as an image and a raster.
- Export a final GeoTIFF (spatially projected) that can be used both in further analysis and in common GIS tools like QGIS.
Things You’ll Need To Complete This Tutorial
To complete this tutorial you will need the most current version of R and, preferably, RStudio installed on your computer.
R Libraries to Install:
-
rhdf5:
install.packages("BiocManager")
,BiocManager::install("rhdf5")
-
terra:
install.packages("terra")
-
neonUtilities:
install.packages("neonUtilities")
More on Packages in R - Adapted from Software Carpentry.
Data to Download
Data will be downloaded in the tutorial using the neonUtilities::byTileAOP
function.
These hyperspectral remote sensing data provide information on the National Ecological Observatory Network's San Joaquin Experimental Range field site in March of 2021. The data were collected over the San Joaquin field site located in California (Domain 17).The entire dataset can be also be downloaded from the NEON Data Portal.
Set Working Directory: This lesson assumes that you have set your working directory to the location of the downloaded data.
An overview of setting the working directory in R can be found here.
R Script & Challenge Code: NEON data lessons often contain challenges to reinforce skills. If available, the code for challenge solutions is found in the downloadable R script of the entire lesson, available in the footer of each lesson page.
About Hyperspectral Remote Sensing Data
The electromagnetic spectrum is composed of thousands of bands representing different types of light energy. Imaging spectrometers (instruments that collect hyperspectral data) break the electromagnetic spectrum into groups of bands that support classification of objects by their spectral properties on the Earth's surface. Hyperspectral data consists of many bands - up to hundreds of bands - that span a portion of the electromagnetic spectrum, from the visible to the Short Wave Infrared (SWIR) regions.
The NEON imaging spectrometer (NIS) collects data within the 380 nm to 2510 nm portions of the electromagnetic spectrum within bands that are approximately 5 nm in width. This results in a hyperspectral data cube that contains approximately 426 bands - which means BIG DATA.
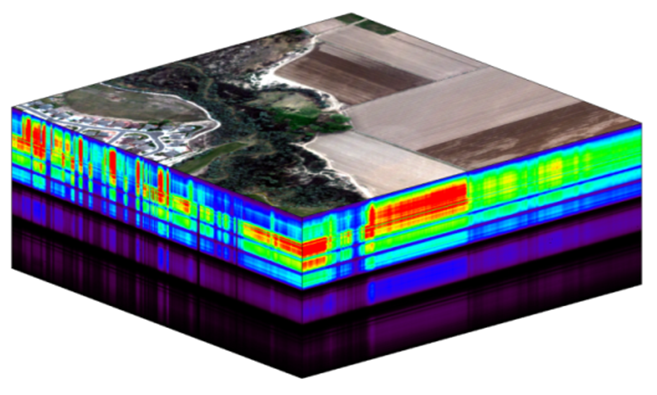
The HDF5 data model natively compresses data stored within it (makes it smaller) and supports data slicing (extracting only the portions of the data that you need to work with rather than reading the entire dataset into memory). These features make it ideal for working with large data cubes such as those generated by imaging spectrometers, in addition to supporting spatial data and associated metadata.
In this tutorial we will demonstrate how to read and extract spatial raster data stored within an HDF5 file using R.
Read HDF5 data into R
We will use the terra
and rhdf5
packages to read in the HDF5 file that contains hyperspectral data for the
NEON San Joaquin (SJER) field site.
Let's start by calling the needed packages and reading in our NEON HDF5 file.
Please be sure that you have at least version 2.10 of rhdf5
installed. Use:
packageVersion("rhdf5")
to check the package version.
Data Tip: To update all packages installed in R, use update.packages()
.
# Load `terra` and `rhdf5` packages to read NIS data into R
library(terra)
library(rhdf5)
library(neonUtilities)
Set the working directory to ensure R can find the file we are importing, and we know where the file is being saved. You can move the file that is downloaded afterward, but be sure to re-set the path to the file.
wd <- "~/data/" #This will depend on your local environment
setwd(wd)
We can use the neonUtilities
function byTileAOP
to download a single reflectance tile. You can run help(byTileAOP)
to see more details on what the various inputs are. For this exercise, we'll specify the UTM Easting and Northing to be (257500, 4112500), which will download the tile with the lower left corner (257000,4112000). By default, the function will check the size total size of the download and ask you whether you wish to proceed (y/n). This file is ~672.7 MB, so make sure you have enough space on your local drive. You can set check.size=FALSE
if you want to download without a prompt.
byTileAOP(dpID='DP3.30006.001',
site='SJER',
year='2021',
easting=257500,
northing=4112500,
check.size=TRUE, # set to FALSE if you don't want to enter y/n
savepath = wd)
This file will be downloaded into a nested subdirectory under the ~/data
folder, inside a folder named DP3.30006.001
(the Data Product ID). The file should show up in this location: ~/data/DP3.30006.001/neon-aop-products/2021/FullSite/D17/2021_SJER_5/L3/Spectrometer/Reflectance/NEON_D17_SJER_DP3_257000_4112000_reflectance.h5
.
Data Tip: To make sure you are pointing to the correct path, look in the ~/data
folder and navigate to where the .h5 file is saved, or use the R command list.files(path=wd,pattern="\\.h5$",recursive=TRUE,full.names=TRUE)
to display the full path of the .h5 file. Note, if you have any other .h5 files downloaded in this folder, it will display all of the hdf5 files.
# Define the h5 file name to be opened
h5_file <- paste0(wd,"DP3.30006.001/neon-aop-products/2021/FullSite/D17/2021_SJER_5/L3/Spectrometer/Reflectance/NEON_D17_SJER_DP3_257000_4112000_reflectance.h5")
You can use h5ls
and/or View(h5ls(...))
to look at the contents of the hdf5 file, as follows:
# look at the HDF5 file structure
View(h5ls(h5_file,all=T))
When you look at the structure of the data, take note of the "map info" dataset, the Coordinate_System
group, and the wavelength
and Reflectance
datasets. The Coordinate_System
folder contains the spatial attributes of the data including its EPSG Code, which is easily converted to a Coordinate Reference System (CRS). The CRS documents how the data are physically located on the Earth. The wavelength
dataset contains the wavelength values for each band in the data. The Reflectance
dataset contains the image data that we will use for both data processing and visualization.
More Information on raster metadata:
-
Raster Data in R - The Basics - this tutorial explains more about how rasters work in R and their associated metadata.
-
About Hyperspectral Remote Sensing Data -this tutorial explains more about metadata and important concepts associated with multi-band (multi and hyperspectral) rasters.
Data Tip - HDF5 Structure: Note that the structure of individual HDF5 files may vary depending on who produced the data. In this case, the Wavelength and reflectance data within the file are both h5 datasets. However, the spatial information is contained within a group. Data downloaded from another organization (like NASA) may look different. This is why it's important to explore the data as a first step!
We can use the h5readAttributes()
function to read and extract metadata from the HDF5 file. Let's start by learning about the wavelengths described within this file.
# get information about the wavelengths of this dataset
wavelengthInfo <- h5readAttributes(h5_file,"/SJER/Reflectance/Metadata/Spectral_Data/Wavelength")
wavelengthInfo
## $Description
## [1] "Central wavelength of the reflectance bands."
##
## $Units
## [1] "nanometers"
Next, we can use the h5read
function to read the data contained within the HDF5 file. Let's read in the wavelengths of the band centers:
# read in the wavelength information from the HDF5 file
wavelengths <- h5read(h5_file,"/SJER/Reflectance/Metadata/Spectral_Data/Wavelength")
head(wavelengths)
## [1] 381.6035 386.6132 391.6229 396.6327 401.6424 406.6522
tail(wavelengths)
## [1] 2485.693 2490.703 2495.713 2500.722 2505.732 2510.742
Which wavelength is band 21 associated with?
(Hint: look at the wavelengths vector that we just imported and check out the data located at index 21 - wavelengths[21]
).
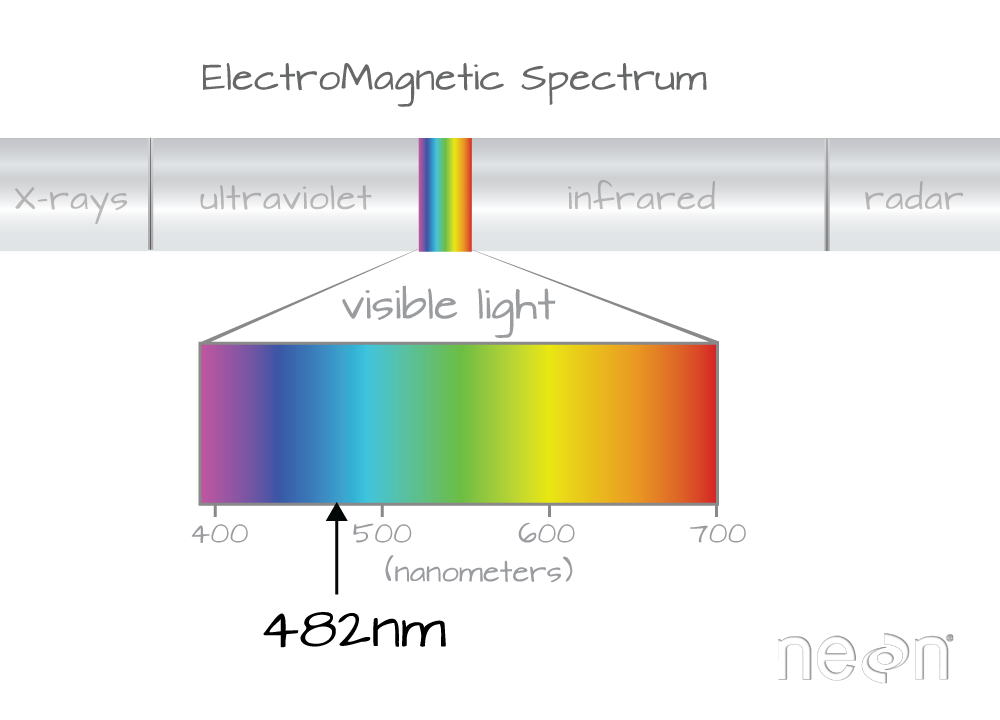
Band 21 has a associated wavelength center of 481.7982 nanometers (nm) which is in the blue portion (~380-500 nm) of the visible electromagnetic spectrum (~380-700 nm).
Bands and Wavelengths
A band represents a group of wavelengths. For example, the wavelength values between 695 nm and 700 nm might be one band captured by an imaging spectrometer. The imaging spectrometer collects reflected light energy in a pixel for light in that band. Often when you work with a multi- or hyperspectral dataset, the band information is reported as the center wavelength value. This value represents the mean value of the wavelengths represented in that band. Thus in a band spanning 695-700 nm, the center would be 697.5 nm). The full width half max (FWHM) will also be reported. This value can be thought of as the spread of the band around that center point. So, a band that covers 800-805 nm might have a FWHM of 5 nm and a wavelength value of 802.5 nm.
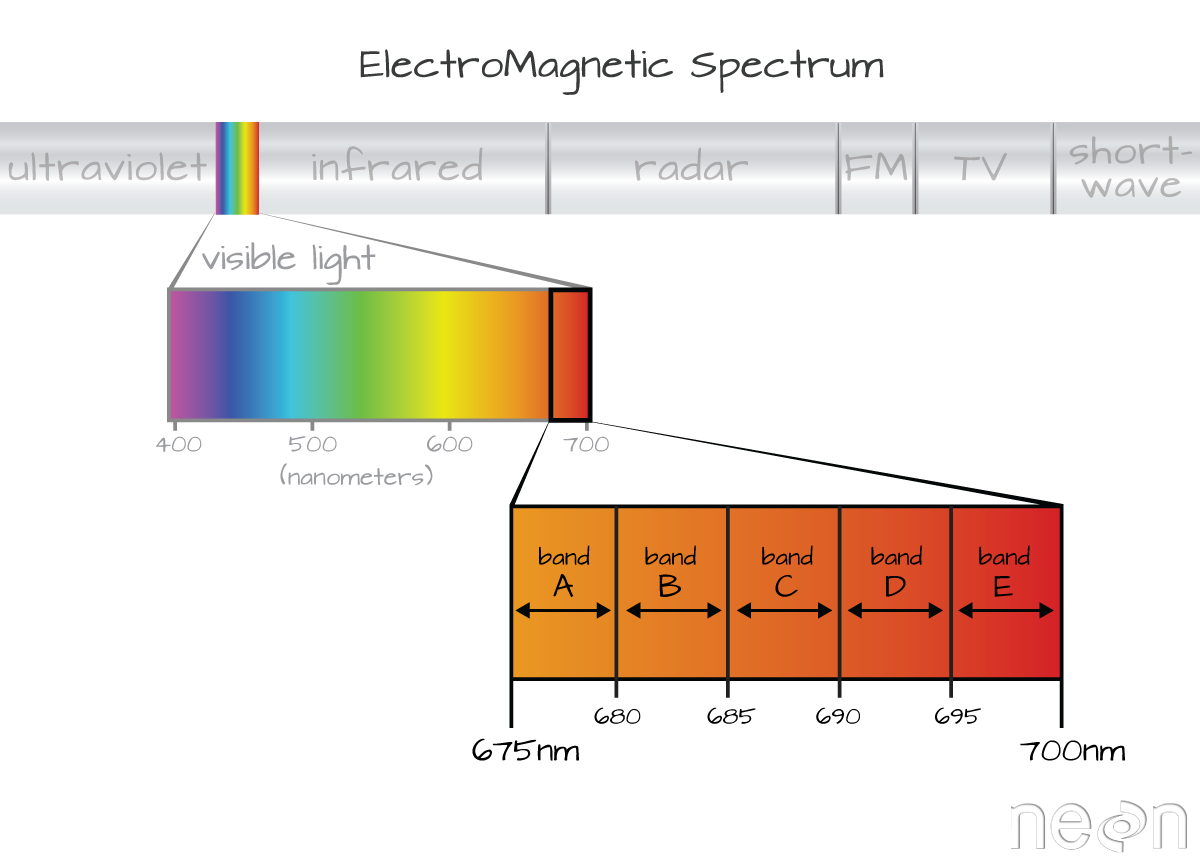
The HDF5 dataset that we are working with in this activity may contain more information than we need to work with. For example, we don't necessarily need to process all 426 bands available in a full NEON hyperspectral reflectance file - if we are interested in creating a product like NDVI which only uses bands in the Near InfraRed (NIR) and Red portions of the spectrum. Or we might only be interested in a spatial subset of the data - perhaps an area where we have collected corresponding ground data in the field.
The HDF5 format allows us to slice (or subset) the data - quickly extracting the subset that we need to process. Let's extract one of the green bands - band 34.
By the way - what is the center wavelength value associated with band 34?
Hint: wavelengths[34]
.
How do we know this band is a green band in the visible portion of the spectrum?
In order to effectively subset our data, let's first read the reflectance metadata stored as attributes in the "Reflectance_Data" dataset.
# First, we need to extract the reflectance metadata:
reflInfo <- h5readAttributes(h5_file, "/SJER/Reflectance/Reflectance_Data")
reflInfo
## $Cloud_conditions
## [1] "For cloud conditions information see Weather Quality Index dataset."
##
## $Cloud_type
## [1] "Cloud type may have been selected from multiple flight trajectories."
##
## $Data_Ignore_Value
## [1] -9999
##
## $Description
## [1] "Atmospherically corrected reflectance."
##
## $Dimension_Labels
## [1] "Line, Sample, Wavelength"
##
## $Dimensions
## [1] 1000 1000 426
##
## $Interleave
## [1] "BSQ"
##
## $Scale_Factor
## [1] 10000
##
## $Spatial_Extent_meters
## [1] 257000 258000 4112000 4113000
##
## $Spatial_Resolution_X_Y
## [1] 1 1
##
## $Units
## [1] "Unitless."
##
## $Units_Valid_range
## [1] 0 10000
# Next, we read the different dimensions
nRows <- reflInfo$Dimensions[1]
nCols <- reflInfo$Dimensions[2]
nBands <- reflInfo$Dimensions[3]
nRows
## [1] 1000
nCols
## [1] 1000
nBands
## [1] 426
The HDF5 read function reads data in the order: Bands, Cols, Rows. This is different from how R reads data. We'll adjust for this later.
# Extract or "slice" data for band 34 from the HDF5 file
b34 <- h5read(h5_file,"/SJER/Reflectance/Reflectance_Data",index=list(34,1:nCols,1:nRows))
# what type of object is b34?
class(b34)
## [1] "array"
A Note About Data Slicing in HDF5
Data slicing allows us to extract and work with subsets of the data rather than reading in the entire dataset into memory. In this example, we will extract and plot the green band without reading in all 426 bands. The ability to slice large datasets makes HDF5 ideal for working with big data.
Next, let's convert our data from an array (more than 2 dimensions) to a matrix (just 2 dimensions). We need to have our data in a matrix format to plot it.
# convert from array to matrix by selecting only the first band
b34 <- b34[1,,]
# display the class of this re-defined variable
class(b34)
## [1] "matrix" "array"
Arrays vs. Matrices
Arrays are matrices with more than 2 dimensions. When we say dimension, we are talking about the "z" associated with the data (imagine a series of tabs in a spreadsheet). Put the other way: matrices are arrays with only 2 dimensions. Arrays can have any number of dimensions one, two, ten or more.
Here is a matrix that is 4 x 3 in size (4 rows and 3 columns):
Metric | species 1 | species 2 |
---|---|---|
total number | 23 | 45 |
average weight | 14 | 5 |
average length | 2.4 | 3.5 |
average height | 32 | 12 |
Dimensions in Arrays
An array contains 1 or more dimensions in the "z" direction. For example, let's say that we collected the same set of species data for every day in a 30 day month. We might then have a matrix like the one above for each day for a total of 30 days making a 4 x 3 x 30 array (this dataset has more than 2 dimensions). More on R object types here (links to external site, DataCamp).
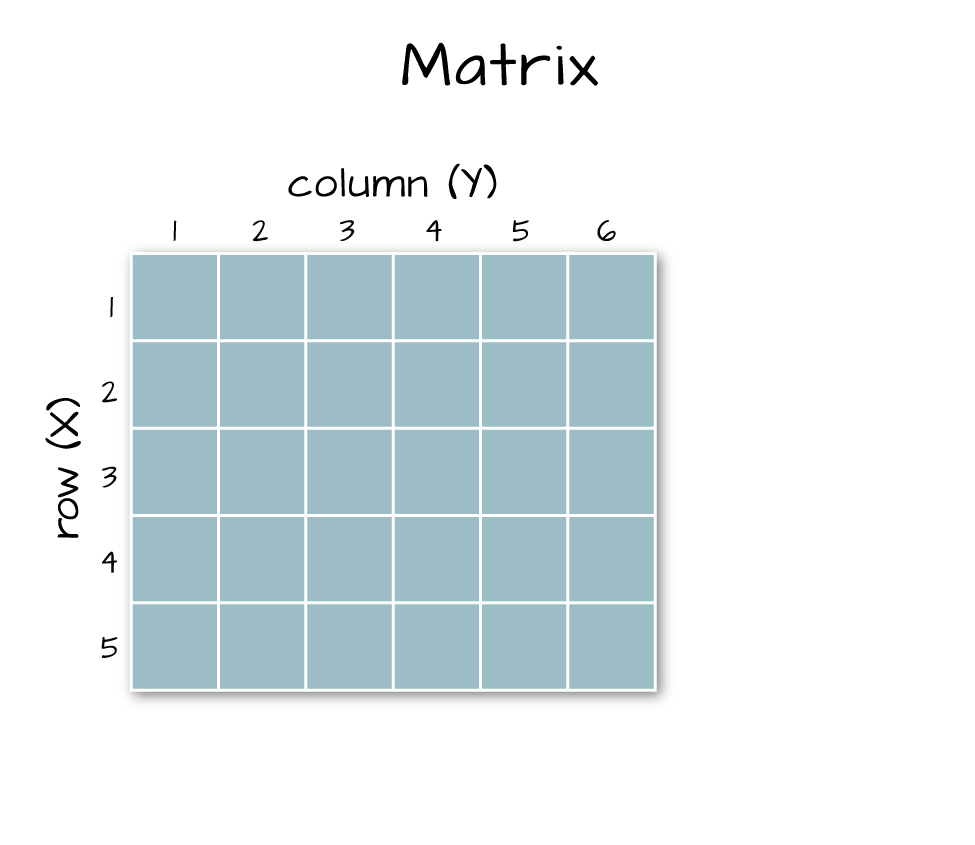
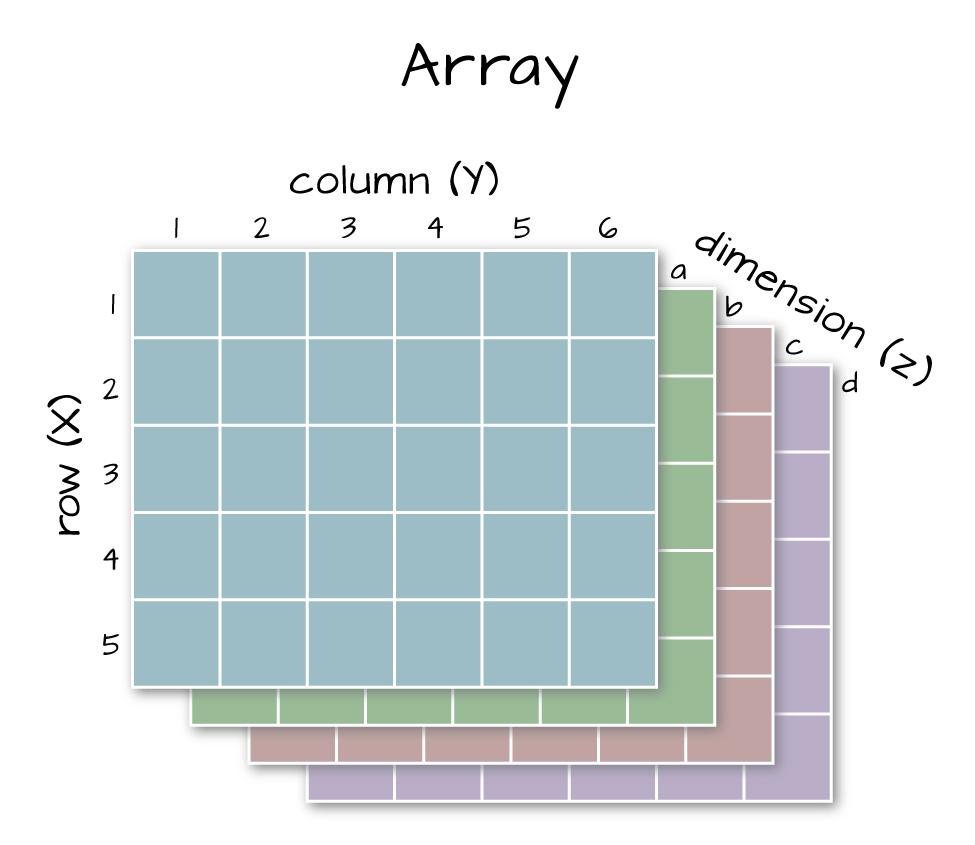
Next, let's look at the metadata for the reflectance data. When we do this, take note of 1) the scale factor and 2) the data ignore value. Then we can plot the band 34 data. Plotting spatial data as a visual "data check" is a good idea to make sure processing is being performed correctly and all is well with the image.
# look at the metadata for the reflectance dataset
h5readAttributes(h5_file,"/SJER/Reflectance/Reflectance_Data")
## $Cloud_conditions
## [1] "For cloud conditions information see Weather Quality Index dataset."
##
## $Cloud_type
## [1] "Cloud type may have been selected from multiple flight trajectories."
##
## $Data_Ignore_Value
## [1] -9999
##
## $Description
## [1] "Atmospherically corrected reflectance."
##
## $Dimension_Labels
## [1] "Line, Sample, Wavelength"
##
## $Dimensions
## [1] 1000 1000 426
##
## $Interleave
## [1] "BSQ"
##
## $Scale_Factor
## [1] 10000
##
## $Spatial_Extent_meters
## [1] 257000 258000 4112000 4113000
##
## $Spatial_Resolution_X_Y
## [1] 1 1
##
## $Units
## [1] "Unitless."
##
## $Units_Valid_range
## [1] 0 10000
# plot the image
image(b34)
What do you notice about the first image? It's washed out and lacking any detail. What could be causing this? It got better when plotting the log of the values, but still not great.
# this is a little hard to visually interpret - what happens if we plot a log of the data?
image(log(b34))
Let's look at the distribution of reflectance values in our data to figure out what is going on.
# Plot range of reflectance values as a histogram to view range
# and distribution of values.
hist(b34,breaks=50,col="darkmagenta")
# View values between 0 and 5000
hist(b34,breaks=100,col="darkmagenta",xlim = c(0, 5000))
# View higher values
hist(b34, breaks=100,col="darkmagenta",xlim = c(5000, 15000),ylim = c(0, 750))
As you're examining the histograms above, keep in mind that reflectance values range between 0-1. The data scale factor in the metadata tells us to divide all reflectance values by 10,000. Thus, a value of 5,000 equates to a reflectance value of 0.50. Storing data as integers (without decimal places) compared to floating points (with decimal places) creates a smaller file. This type of scaling is commin in remote sensing datasets.
Notice in the data that there are some larger reflectance values (>5,000) that represent a smaller number of pixels. These pixels are skewing how the image renders.
Data Ignore Value
Image data in raster format will often contain a data ignore value and a scale factor. The data ignore value represents pixels where there are no data. Among other causes, no data values may be attributed to the sensor not collecting data in that area of the image or to processing results which yield null values.
Remember that the metadata for the Reflectance
dataset designated -9999 as data ignore value
. Thus, let's set all pixels with a value == -9999 to NA
(no value). If we do this, R won't render these pixels.
# there is a no data value in our raster - let's define it
noDataValue <- as.numeric(reflInfo$Data_Ignore_Value)
noDataValue
## [1] -9999
# set all values equal to the no data value (-9999) to NA
b34[b34 == noDataValue] <- NA
# plot the image now
image(b34)
Reflectance Values and Image Stretch
Our image still looks dark because R is trying to render all reflectance values between 0 and 14999 as if they were distributed equally in the histogram. However we know they are not distributed equally. There are many more values between 0-5000 than there are values >5000.
Images contain a distribution of reflectance values. A typical image viewing program will render the values by distributing the entire range of reflectance values across a range of "shades" that the monitor can render - between 0 and 255. However, often the distribution of reflectance values is not linear. For example, in the case of our data, most of the reflectance values fall between 0 and 0.5. Yet there are a few values >0.8 that are heavily impacting the way the image is drawn on our monitor. Imaging processing programs like ENVI, QGIS and ArcGIS (and even Adobe Photoshop) allow you to adjust the stretch of the image. This is similar to adjusting the contrast and brightness in Photoshop.
The proper way to adjust our data would be to apply what's called an image stretch
. We will learn how to stretch our image data later. For now, let's plot the values as the log function on the pixel reflectance values to factor out those larger values.
image(log(b34))
The log applied to our image increases the contrast making it look more like an image. However, look at the images below. The top one is an RGB image as the image should look. The bottom one is our log-adjusted image. Notice a difference?
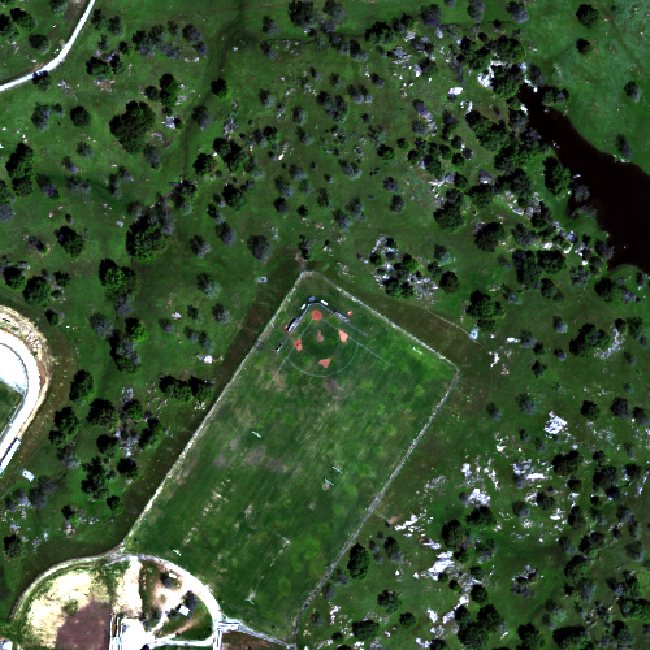
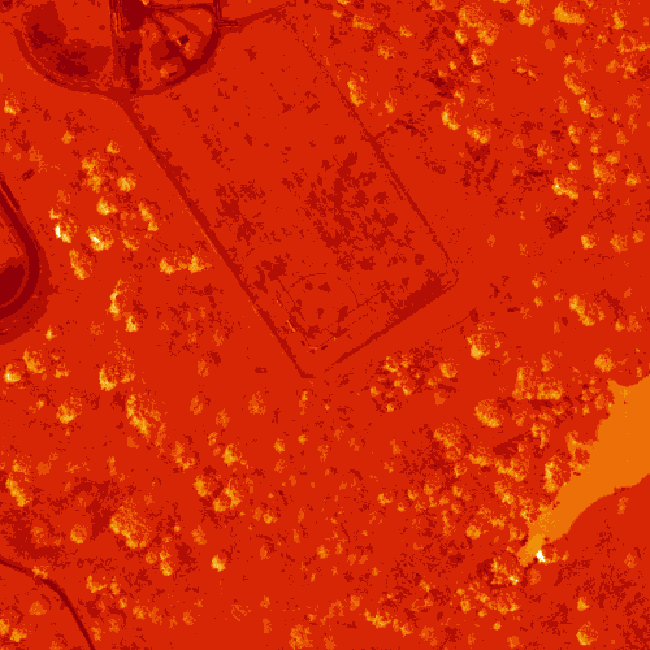
Transpose Image
Notice that there are three data dimensions for this file: Bands x Rows x Columns. However, when R reads in the dataset, it reads them as: Columns x Bands x Rows. The data are flipped. We can quickly transpose the data to correct for this using the t
or transpose
command in R.
The orientation is rotated in our log adjusted image. This is because R reads in matrices starting from the upper left hand corner. While most rasters read pixels starting from the lower left hand corner. In the next section, we will deal with this issue by creating a proper georeferenced (spatially located) raster in R. The raster format will read in pixels following the same methods as other GIS and imaging processing software like QGIS and ENVI do.
# We need to transpose x and y values in order for our
# final image to plot properly
b34 <- t(b34)
image(log(b34), main="Transposed Image")
Create a Georeferenced Raster
Next, we will create a proper raster using the b34
matrix. The raster format will allow us to define and manage:
- Image stretch
- Coordinate reference system & spatial reference
- Resolution
- and other raster attributes...
It will also account for the orientation issue discussed above.
To create a raster in R, we need a few pieces of information, including:
- The coordinate reference system (CRS)
- The spatial extent of the image
Define Raster CRS
First, we need to define the Coordinate reference system (CRS) of the raster. To do that, we can first grab the EPSG code from the HDF5 attributes, and covert the EPSG to a CRS string. Then we can assign that CRS to the raster object.
# Extract the EPSG from the h5 dataset
h5EPSG <- h5read(h5_file, "/SJER/Reflectance/Metadata/Coordinate_System/EPSG Code")
# convert the EPSG code to a CRS string
h5CRS <- crs(paste0("+init=epsg:",h5EPSG))
# define final raster with projection info
# note that capitalization will throw errors on a MAC.
# if UTM is all caps it might cause an error!
b34r <- rast(b34,
crs=h5CRS)
# view the raster attributes
b34r
## class : SpatRaster
## dimensions : 1000, 1000, 1 (nrow, ncol, nlyr)
## resolution : 1, 1 (x, y)
## extent : 0, 1000, 0, 1000 (xmin, xmax, ymin, ymax)
## coord. ref. : WGS 84 / UTM zone 11N
## source(s) : memory
## name : lyr.1
## min value : 32
## max value : 13129
# let's have a look at our properly oriented raster. Take note of the
# coordinates on the x and y axis.
image(log(b34r),
xlab = "UTM Easting",
ylab = "UTM Northing",
main = "Properly Oriented Raster")
Next we define the extents of our raster. The extents will be used to calculate the raster's resolution. Fortunately, the spatial extent is provided in the HDF5 file "Reflectance_Data" group attributes that we saved before as reflInfo
.
# Grab the UTM coordinates of the spatial extent
xMin <- reflInfo$Spatial_Extent_meters[1]
xMax <- reflInfo$Spatial_Extent_meters[2]
yMin <- reflInfo$Spatial_Extent_meters[3]
yMax <- reflInfo$Spatial_Extent_meters[4]
# define the extent (left, right, top, bottom)
rasExt <- ext(xMin,xMax,yMin,yMax)
rasExt
## SpatExtent : 257000, 258000, 4112000, 4113000 (xmin, xmax, ymin, ymax)
# assign the spatial extent to the raster
ext(b34r) <- rasExt
# look at raster attributes
b34r
## class : SpatRaster
## dimensions : 1000, 1000, 1 (nrow, ncol, nlyr)
## resolution : 1, 1 (x, y)
## extent : 257000, 258000, 4112000, 4113000 (xmin, xmax, ymin, ymax)
## coord. ref. : WGS 84 / UTM zone 11N
## source(s) : memory
## name : lyr.1
## min value : 32
## max value : 13129
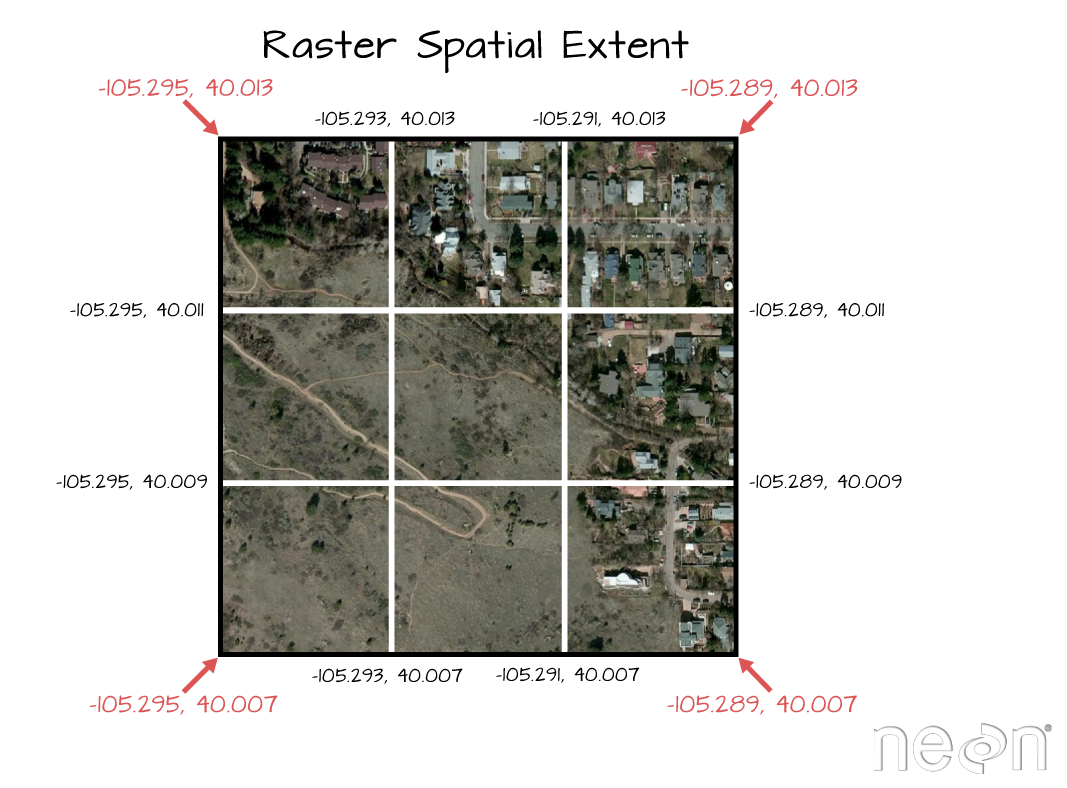
Learn more about working with Raster data in R in the Data Carpentry workshop: Introduction to Geospatial Raster and Vector Data with R.
We can adjust the colors of our raster as well, if desired.
# let's change the colors of our raster and adjust the zlim
col <- terrain.colors(25)
image(b34r,
xlab = "UTM Easting",
ylab = "UTM Northing",
main= "Spatially Referenced Raster",
col=col,
zlim=c(0,3000))
We've now created a raster from band 34 reflectance data. We can export the data as a raster, using the writeRaster
command. Note that it's good practice to close the H5 connection before moving on!
# write out the raster as a geotiff
writeRaster(b34r,
file=paste0(wd,"band34.tif"),
overwrite=TRUE)
# close the H5 file
H5close()
Challenge: Work with Rasters
Try these three extensions on your own:
-
Create rasters using other bands in the dataset.
-
Vary the distribution of values in the image to mimic an image stretch. e.g.
b34[b34 > 6000 ] <- 6000
-
Use what you know to extract ALL of the reflectance values for ONE pixel rather than for an entire band. HINT: this will require you to pick an x and y value and then all values in the z dimension:
aPixel<- h5read(h5_file,"Reflectance",index=list(NULL,100,35))
. Plot the spectra output.
Creating a Raster Stack from Hyperspectral Imagery in HDF5 Format in R
In this tutorial, we will learn how to create multi (3) band images from hyperspectral data. We will also learn how to perform some basic raster calculations (known as raster math in the GIS world).
Learning Objectives
After completing this activity, you will be able to:
- Extract a "slice" of data from a hyperspectral data cube.
- Create a raster "stack" in R which can be used to create RGB images from band combinations in a hyperspectral data cube.
- Plot data spatially on a map.
- Create basic vegetation indices like NDVI using raster-based calculations in R.
Things You’ll Need To Complete This Tutorial
To complete this tutorial you will need the most current version of R and, preferably, RStudio loaded on your computer.
R Libraries to Install:
-
rhdf5:
install.packages("BiocManager")
,BiocManager::install("rhdf5")
-
terra:
install.packages("terra")
-
neonUtilities:
install.packages("neonUtilities")
More on Packages in R - Adapted from Software Carpentry.
Data
These hyperspectral remote sensing data provide information on the National Ecological Observatory Network's San Joaquin Experimental Range (SJER) field site in March of 2021. The data used in this lesson is the 1km by 1km mosaic tile named NEON_D17_SJER_DP3_257000_4112000_reflectance.h5. If you already completed the previous lesson in this tutorial series, you do not need to download this data again. The entire SJER reflectance dataset can be accessed from the NEON Data Portal.
Set Working Directory: This lesson assumes that you have set your working directory to the location of the downloaded data, as explained in the tutorial.
An overview of setting the working directory in R can be found here.
R Script & Challenge Code: NEON data lessons often contain challenges to reinforce skills. If available, the code for challenge solutions is found in the downloadable R script of the entire lesson, available in the footer of each lesson page.
Recommended Skills
For this tutorial you should be comfortable working with HDF5 files that contain hyperspectral data, including reading in reflectance values and associated metadata and attributes.
If you aren't familiar with these steps already, we highly recommend you work through the Introduction to Working with Hyperspectral Data in HDF5 Format in R tutorial before moving on to this tutorial.
About Hyperspectral Data
We often want to generate a 3 band image from multi or hyperspectral data. The most commonly recognized band combination is RGB which stands for Red, Green and Blue. RGB images are just like an image that your camera takes. But other band combinations can be useful too. For example, near infrared images highlight healthy vegetation, which makes it easy to classify or identify where vegetation is located on the ground.
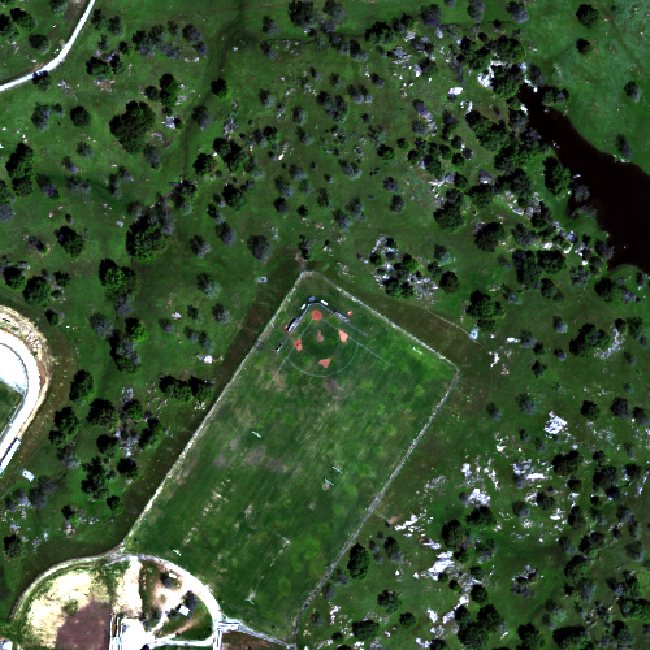
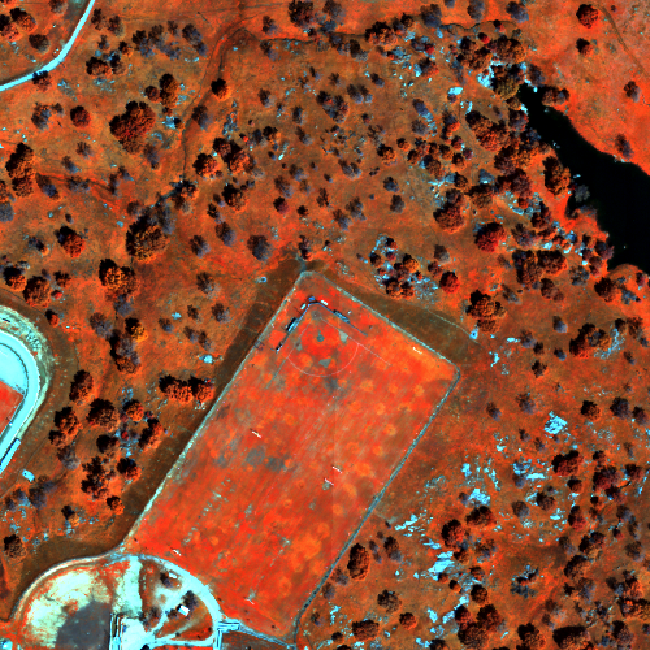
Data Tip - Band Combinations: The Biodiversity Informatics group created a great interactive tool that lets you explore band combinations. Check it out. Learn more about band combinations using a great online tool from the American Museum of Natural History! (The tool requires Flash player.)
Create a Raster Stack in R
In the previous lesson, we exported a single band of the NEON Reflectance data from a HDF5 file. In this activity, we will create a full color image using 3 (red, green and blue - RGB) bands. We will follow many of the steps we followed in the Intro to Working with Hyperspectral Remote Sensing Data in HDF5 Format in R tutorial. These steps included loading required packages, downloading the data (optionally, you don't need to do this if you downloaded the data from the previous lesson), and reading in our file and viewing the hdf5 file structure.
First, let's load the required R packages, terra
and rhdf5
.
library(terra)
library(rhdf5)
library(neonUtilities)
Next set the working directory to ensure R can find the file we wish to import. Be sure to move the download into your working directory!
# set working directory (this will depend on your local environment)
wd <- "~/data/"
setwd(wd)
We can use the neonUtilities
function byTileAOP
to download a single reflectance tile. You can run help(byTileAOP)
to see more details on what the various inputs are. For this exercise, we'll specify the UTM Easting and Northing to be (257500, 4112500), which will download the tile with the lower left corner (257000, 4112000).
byTileAOP(dpID = 'DP3.30006.001',
site = 'SJER',
year = '2021',
easting = 257500,
northing = 4112500,
savepath = wd)
This file will be downloaded into a nested subdirectory under the ~/data
folder, inside a folder named DP3.30006.001
(the Data Product ID). The file should show up in this location: ~/data/DP3.30006.001/neon-aop-products/2021/FullSite/D17/2021_SJER_5/L3/Spectrometer/Reflectance/NEON_D17_SJER_DP3_257000_4112000_reflectance.h5
.
Now we can read in the file. You can move this file to a different location, but make sure to change the path accordingly.
# Define the h5 file name to be opened
h5_file <- paste0(wd,"DP3.30006.001/neon-aop-products/2021/FullSite/D17/2021_SJER_5/L3/Spectrometer/Reflectance/NEON_D17_SJER_DP3_257000_4112000_reflectance.h5")
As in the last lesson, let's use View(h5ls)
to take a look inside this hdf5 dataset:
View(h5ls(h5_file,all=T))
To spatially locate our raster data, we need a few key attributes:
- The coordinate reference system
- The spatial extent of the raster
We'll begin by grabbing these key attributes from the H5 file.
# define coordinate reference system from the EPSG code provided in the HDF5 file
h5EPSG <- h5read(h5_file,"/SJER/Reflectance/Metadata/Coordinate_System/EPSG Code" )
h5CRS <- crs(paste0("+init=epsg:",h5EPSG))
# get the Reflectance_Data attributes
reflInfo <- h5readAttributes(h5_file,"/SJER/Reflectance/Reflectance_Data" )
# Grab the UTM coordinates of the spatial extent
xMin <- reflInfo$Spatial_Extent_meters[1]
xMax <- reflInfo$Spatial_Extent_meters[2]
yMin <- reflInfo$Spatial_Extent_meters[3]
yMax <- reflInfo$Spatial_Extent_meters[4]
# define the extent (left, right, top, bottom)
rastExt <- ext(xMin,xMax,yMin,yMax)
# view the extent to make sure that it looks right
rastExt
## SpatExtent : 257000, 258000, 4112000, 4113000 (xmin, xmax, ymin, ymax)
# Finally, define the no data value for later
h5NoDataValue <- as.integer(reflInfo$Data_Ignore_Value)
cat('No Data Value:',h5NoDataValue)
## No Data Value: -9999
Next, we'll write a function that will perform the processing that we did step by step in the Intro to Working with Hyperspectral Remote Sensing Data in HDF5 Format in R. This will allow us to process multiple bands in bulk.
The function band2Rast
slices a band of data from the HDF5 file, and extracts the reflectance array for that band. It then converts the data into a matrix, converts it to a raster, and finally returns a spatially corrected raster for the specified band.
The function requires the following variables:
- file: the hdf5 reflectance file
- band: the band number we wish to extract
- noDataValue: the noDataValue for the raster
-
extent: a terra spatial extent (
SpatExtent
) object . - crs: the Coordinate Reference System for the raster
The function output is a spatially referenced, R terra object.
# file: the hdf5 file
# band: the band you want to process
# returns: a matrix containing the reflectance data for the specific band
band2Raster <- function(file, band, noDataValue, extent, CRS){
# first, read in the raster
out <- h5read(file,"/SJER/Reflectance/Reflectance_Data",index=list(band,NULL,NULL))
# Convert from array to matrix
out <- (out[1,,])
# transpose data to fix flipped row and column order
# depending upon how your data are formatted you might not have to perform this
# step.
out <- t(out)
# assign data ignore values to NA
# note, you might chose to assign values of 15000 to NA
out[out == noDataValue] <- NA
# turn the out object into a raster
outr <- rast(out,crs=CRS)
# assign the extents to the raster
ext(outr) <- extent
# return the terra raster object
return(outr)
}
Now that the function is created, we can create our list of rasters. The list specifies which bands (or dimensions in our hyperspectral dataset) we want to include in our raster stack. Let's start with a typical RGB (red, green, blue) combination. We will use bands 14, 9, and 4 (bands 58, 34, and 19 in a full NEON hyperspectral dataset).
Data Tip - wavelengths and bands: Remember that you can look at the wavelengths dataset in the HDF5 file to determine the center wavelength value for each band. Keep in mind that this data subset only includes every fourth band that is available in a full NEON hyperspectral dataset!
# create a list of the bands (R,G,B) we want to include in our stack
rgb <- list(58,34,19)
# lapply tells R to apply the function to each element in the list
rgb_rast <- lapply(rgb,FUN=band2Raster, file = h5_file,
noDataValue=h5NoDataValue,
ext=rastExt,
CRS=h5CRS)
Check out the properties or rgb_rast:
rgb_rast
## [[1]]
## class : SpatRaster
## dimensions : 1000, 1000, 1 (nrow, ncol, nlyr)
## resolution : 1, 1 (x, y)
## extent : 257000, 258000, 4112000, 4113000 (xmin, xmax, ymin, ymax)
## coord. ref. : WGS 84 / UTM zone 11N
## source(s) : memory
## name : lyr.1
## min value : 0
## max value : 14950
##
## [[2]]
## class : SpatRaster
## dimensions : 1000, 1000, 1 (nrow, ncol, nlyr)
## resolution : 1, 1 (x, y)
## extent : 257000, 258000, 4112000, 4113000 (xmin, xmax, ymin, ymax)
## coord. ref. : WGS 84 / UTM zone 11N
## source(s) : memory
## name : lyr.1
## min value : 32
## max value : 13129
##
## [[3]]
## class : SpatRaster
## dimensions : 1000, 1000, 1 (nrow, ncol, nlyr)
## resolution : 1, 1 (x, y)
## extent : 257000, 258000, 4112000, 4113000 (xmin, xmax, ymin, ymax)
## coord. ref. : WGS 84 / UTM zone 11N
## source(s) : memory
## name : lyr.1
## min value : 9
## max value : 11802
Note that it displays properties of 3 rasters. Finally, we can create a raster stack from our list of rasters as follows:
rgbStack <- rast(rgb_rast)
In the code chunk above, we used the lapply()
function, which is a powerful, flexible way to apply a function (in this case, our band2Raster()
function) multiple times. You can learn more about lapply() here.
NOTE: We are using the raster stack
object in R to store several rasters that are of the same CRS and extent. This is a popular and convenient way to organize co-incident rasters.
Next, add the names of the bands to the raster so we can easily keep track of the bands in the list.
# Create a list of band names
bandNames <- paste("Band_",unlist(rgb),sep="")
# set the rasterStack's names equal to the list of bandNames created above
names(rgbStack) <- bandNames
# check properties of the raster list - note the band names
rgbStack
## class : SpatRaster
## dimensions : 1000, 1000, 3 (nrow, ncol, nlyr)
## resolution : 1, 1 (x, y)
## extent : 257000, 258000, 4112000, 4113000 (xmin, xmax, ymin, ymax)
## coord. ref. : WGS 84 / UTM zone 11N
## source(s) : memory
## names : Band_58, Band_34, Band_19
## min values : 0, 32, 9
## max values : 14950, 13129, 11802
# scale the data as specified in the reflInfo$Scale Factor
rgbStack <- rgbStack/as.integer(reflInfo$Scale_Factor)
# plot one raster in the stack to make sure things look OK.
plot(rgbStack$Band_58, main="Band 58")
We can play with the color ramps too if we want:
# change the colors of our raster
colors1 <- terrain.colors(25)
image(rgbStack$Band_58, main="Band 58", col=colors1)
# adjust the zlims or the stretch of the image
image(rgbStack$Band_58, main="Band 58", col=colors1, zlim = c(0,.5))
# try a different color palette
colors2 <- topo.colors(15, alpha = 1)
image(rgbStack$Band_58, main="Band 58", col=colors2, zlim=c(0,.5))
The plotRGB
function allows you to combine three bands to create an true-color image.
# create a 3 band RGB image
plotRGB(rgbStack,
r=1,g=2,b=3,
stretch = "lin")
A note about image stretching: Notice that we use the argument stretch="lin"
in this plotting function, which automatically stretches the brightness values for us to produce a natural-looking image.
Once you've created your raster, you can export it as a GeoTIFF using writeRaster
. You can bring this GeoTIFF into any GIS software, such as QGIS or ArcGIS.
# Write out final raster
# Note: if you set overwrite to TRUE, then you will overwrite (and lose) any older version of the tif file!
writeRaster(rgbStack, file=paste0(wd,"NEON_hyperspectral_tutorial_example_RGB_image.tif"), overwrite=TRUE)
Data Tip - False color and near infrared images: Use the band combinations listed at the top of this page to modify the raster list. What type of image do you get when you change the band values?
Challenge: Other band combinations
Use different band combinations to create other "RGB" images. Suggested band combinations are below for use with the full NEON hyperspectral reflectance datasets (for this example dataset, divide the band number by 4 and round to the nearest whole number):
- Color Infrared/False Color: rgb (90,34,19)
- SWIR, NIR, Red Band: rgb (152,90,58)
- False Color: rgb (363,246,55)
Raster Math - Creating NDVI and other Vegetation Indices in R
In this last part, we will calculate some vegetation indices using raster math in R! We will start by creating NDVI or Normalized Difference Vegetation Index.
About NDVI
NDVI is a ratio between the near infrared (NIR) portion of the electromagnetic spectrum and the red portion of the spectrum.
$$ NDVI = \frac{NIR-RED}{NIR+RED} $$
Please keep in mind that there are different ways to aggregate bands when using hyperspectral data. This example is using individual bands to perform the NDVI calculation. Using individual bands is not necessarily the best way to calculate NDVI from hyperspectral data.
# Calculate NDVI
# select bands to use in calculation (red, NIR)
ndviBands <- c(58,90)
# create raster list and then a stack using those two bands
ndviRast <- lapply(ndviBands,FUN=band2Raster, file = h5_file,
noDataValue=h5NoDataValue,
ext=rastExt, CRS=h5CRS)
ndviStack <- rast(ndviRast)
# make the names pretty
bandNDVINames <- paste("Band_",unlist(ndviBands),sep="")
names(ndviStack) <- bandNDVINames
# view the properties of the new raster stack
ndviStack
## class : SpatRaster
## dimensions : 1000, 1000, 2 (nrow, ncol, nlyr)
## resolution : 1, 1 (x, y)
## extent : 257000, 258000, 4112000, 4113000 (xmin, xmax, ymin, ymax)
## coord. ref. : WGS 84 / UTM zone 11N
## source(s) : memory
## names : Band_58, Band_90
## min values : 0, 11
## max values : 14950, 14887
#calculate NDVI
NDVI <- function(x) {
(x[,2]-x[,1])/(x[,2]+x[,1])
}
ndviCalc <- app(ndviStack,NDVI)
plot(ndviCalc, main="NDVI for the NEON SJER Field Site")
# Now, play with breaks and colors to create a meaningful map
# add a color map with 4 colors
myCol <- rev(terrain.colors(4)) # use the 'rev()' function to put green as the highest NDVI value
# add breaks to the colormap, including lowest and highest values (4 breaks = 3 segments)
brk <- c(0, .25, .5, .75, 1)
# plot the image using breaks
plot(ndviCalc, main="NDVI for the NEON SJER Field Site", col=myCol, breaks=brk)
Challenge: Work with Indices
Try the following on your own:
- Calculate the Normalized Difference Nitrogen Index (NDNI) using the following equation:
$$ NDNI = \frac{log(\frac{1}{p_{1510}}) - log(\frac{1}{p_{1680}})}{log(\frac{1}{p_{1510}}) + log(\frac{1}{p_{1680}})} $$
-
Calculate the Enhanced Vegetation Index (EVI). Hint: Look up the formula, and apply the appropriate NEON bands. Hint: You can look at satellite datasets, such as USGS Landsat EVI.
-
Explore the bands in the hyperspectral data. What happens if you average reflectance values across multiple Red and NIR bands and then calculate NDVI?