Workshop
NEON Brownbag: Intro to Working with HDF5
NEON
This workshop will provide hands on experience with working hierarchical data formats (HDF5) in R.
Objectives
After completing this workshop, you will be able to:
- Describe what the Hierarchical Data Format (HDF5) is.
- Create and read from HDF5 files in R.
- Read and visualization time series data stored in an HDF5 format.
Things to Do Before the Workshop
To participant in this workshop, you will need a laptop with the most current version of R and, preferably, RStudio loaded on your computer. For details on setting up R & RStudio in Mac, PC, or Linux operating systems please see Additional Set up Resources below.
Install R Libraries
Please install or update each package prior to the start of the workshop.
-
rhdf5:
source("http://bioconductor.org/biocLite.R") biocLite("rhdf5")
-
ggplot2:
install.packages("ggplot2")
-
dpylr:
install.packages("dplyr")
: data manipulation at its finest! -
scales:
install.packages("scales")
: this library makes it easier to plot time series data
Data to Download
[[nid:6329]] [[nid:6330]] [[nid:6332]]
Background Reading
- If you are unfamiliar with the HDF5 format, please read * Hierarchical Data Formats - What is HDF5?* tutorial
Schedule
Additional Set Up Instructions
R & RStudio
Prior to the workshop you should have R and, preferably, RStudio installed on your computer.
[[nid:6408]] [[nid:6512]]
Install HDFView
The free HDFView application allows you to explore the contents of an HDF5 file.
To install HDFView:
-
Click to go to the download page.
-
From the section titled HDF-Java 2.1x Pre-Built Binary Distributions select the HDFView download option that matches the operating system and computer setup (32 bit vs 64 bit) that you have. The download will start automatically.
-
Open the downloaded file.
- Mac - You may want to add the HDFView application to your Applications directory.
- Windows - Unzip the file, open the folder, run the .exe file, and follow directions to complete installation.
- Open HDFView to ensure that the program installed correctly.
QGIS (Optional)
QGIS is a cross-platform Open Source Geographic Information system.
Online LiDAR Data/las Viewer (Optional)
Plas.io is a open source LiDAR data viewer developed by Martin Isenberg of Las Tools and several of his colleagues.
Schedule
Filter, Piping, and GREPL Using R DPLYR - An Intro
Learning Objectives
After completing this tutorial, you will be able to:
- Filter data, alone and combined with simple pattern matching grepl().
- Use the group_by function in dplyr.
- Use the summarise function in dplyr.
- "Pipe" functions using dplyr syntax.
Things You’ll Need To Complete This Tutorial
You will need the most current version of R and, preferably, RStudio
loaded
on your computer to complete this tutorial.
Install R Packages
-
neonUtilities:
install.packages("neonUtilities")
tools for working with NEON data -
dplyr:
install.packages("dplyr")
used for data manipulation
Intro to dplyr
When working with data frames in R, it is often useful to manipulate and
summarize data. The dplyr
package in R offers one of the most comprehensive
group of functions to perform common manipulation tasks. In addition, the
dplyr
functions are often of a simpler syntax than most other data
manipulation functions in R.
Elements of dplyr
There are several elements of dplyr
that are unique to the library, and that
do very cool things!
Functions for manipulating data
The text below was exerpted from the R CRAN dpylr vignettes.
Dplyr aims to provide a function for each basic verb of data manipulating, like:
-
filter()
(andslice()
)- filter rows based on values in specified columns
-
arrange()
- sort data by values in specified columns
-
select()
(andrename()
)- view and work with data from only specified columns
-
distinct()
- view and work with only unique values from specified columns
-
mutate()
(andtransmute()
)- add new data to the data frame
-
summarise()
- calculate specified summary statistics on data
-
sample_n()
andsample_frac()
- return a random sample of rows
Format of function calls
The single table verb functions share these features:
- The first argument is a
data.frame
(or a dplyr special class tbl_df, known as a 'tibble').-
dplyr
can work with data.frames as is, but if you're dealing with large data it's worthwhile to convert them to a tibble, to avoid printing a lot of data to the screen. You can do this with the function
as_tibble()
. - Calling the class function on a tibble will return the vector
c("tbl_df", "tbl", "data.frame")
.
-
- The subsequent arguments describe how to manipulate the data (e.g., based on which columns, using which summary statistics), and you can refer to columns directly (without using $).
- The result is always a new tibble.
- Function calls do not generate 'side-effects'; you always have to assign the results to an object
Grouped operations
Certain functions (e.g., group_by, summarise, and other 'aggregate functions') allow you to get information for groups of data, in one fell swoop. This is like performing database functions with knowing SQL or any other db specific code. Powerful stuff!
Piping
We often need to get a subset of data using one function, and then use another function to do something with that subset (and we may do this multiple times). This leads to nesting functions, which can get messy and hard to keep track of. Enter 'piping', dplyr's way of feeding the output of one function into another, and so on, without the hassleof parentheses and brackets.
Let's say we want to start with the data frame my_data
, apply function1()
,
then function2()
, and then function3()
. This is what that looks like without
piping:
function3(function2(function1(my_data)))
This is messy, difficult to read, and the reverse of the order our functions actually occur. If any of these functions needed additional arguments, the readability would be even worse!
The piping operator %>%
takes everything in front of it and "pipes" it into
the first argument of the function after. So now our example looks like this:
my_data %>%
function1() %>%
function2() %>%
function3()
This runs identically to the original nested version!
For example, if we want to find the mean body weight of male mice, we'd do this:
myMammalData %>% # start with a data frame
filter(sex=='M') %>% # first filter for rows where sex is male
summarise (mean_weight = mean(weight)) # find the mean of the weight
# column, store as mean_weight
This is read as "from data frame myMammalData
, select only males and return
the mean weight as a new list mean_weight
".
Download Small Mammal Data
Before we get started, we will need to download our data to investigate. To
do so, we will use the loadByProduct()
function from the neonUtilities
package to download data straight from the NEON servers. To learn more about
this function, please see the Download and Explore NEON data tutorial here.
Let's look at the NEON small mammal capture data from Harvard Forest (within Domain 01) for all of 2014. This site is located in the heart of the Lyme disease epidemic.
Read more about NEON terrestrial measurements here.
# load packages
library(dplyr)
library(neonUtilities)
# load the NEON small mammal capture data
# NOTE: the check.size = TRUE argument means the function
# will require confirmation from you that you want to load
# the quantity of data requested
loadData <- loadByProduct(dpID="DP1.10072.001", site = "HARV",
startdate = "2014-01", enddate = "2014-12",
check.size = TRUE) # Console requires your response!
# if you'd like, check out the data
str(loadData)
The loadByProduct()
function calls the NEON server, downloads the monthly
data files, and 'stacks' them together to form two tables of data called
'mam_pertrapnight' and 'mam_perplotnight'. It also saves the readme file,
explanations of variables, and validation metadata, and combines these all into
a single 'list' that we called 'loadData'. The only part of this list that we
really need for this tutorial is the 'mam_pertrapnight' table, so let's extract
just that one and call it 'myData'.
myData <- loadData$mam_pertrapnight
class(myData) # Confirm that 'myData' is a data.frame
## [1] "data.frame"
Use dplyr
For the rest of this tutorial, we are only going to be working with three variables within 'myData':
-
scientificName
a string of "Genus species" -
sex
a string with "F", "M", or "U" -
identificationQualifier
a string noting uncertainty in the species identification
filter()
This function:
- extracts only a subset of rows from a data frame according to specified conditions
- is similar to the base function
subset()
, but with simpler syntax - inputs: data object, any number of conditional statements on the named columns of the data object
- output: a data object of the same class as the input object (e.g., data.frame in, data.frame out) with only those rows that meet the conditions
For example, let's create a new dataframe that contains only female Peromyscus mainculatus, one of the key small mammal players in the life cycle of Lyme disease-causing bacterium.
# filter on `scientificName` is Peromyscus maniculatus and `sex` is female.
# two equals signs (==) signifies "is"
data_PeroManicFemales <- filter(myData,
scientificName == 'Peromyscus maniculatus',
sex == 'F')
# Note how we were able to put multiple conditions into the filter statement,
# pretty cool!
So we have a dataframe with our female P. mainculatus but how many are there?
# how many female P. maniculatus are in the dataset
# would could simply count the number of rows in the new dataset
nrow(data_PeroManicFemales)
## [1] 98
# or we could write is as a sentence
print(paste('In 2014, NEON technicians captured',
nrow(data_PeroManicFemales),
'female Peromyscus maniculatus at Harvard Forest.',
sep = ' '))
## [1] "In 2014, NEON technicians captured 98 female Peromyscus maniculatus at Harvard Forest."
That's awesome that we can quickly and easily count the number of female
Peromyscus maniculatus that were captured at Harvard Forest in 2014. However,
there is a slight problem. There are two Peromyscus species that are common
in Harvard Forest: Peromyscus maniculatus (deer mouse) and Peromyscus leucopus
(white-footed mouse). These species are difficult to distinguish in the field,
leading to some uncertainty in the identification, which is noted in the
'identificationQualifier' data field by the term "cf. species" or "aff. species".
(For more information on these terms and 'open nomenclature' please see this great Wiki article here).
When the field technician is certain of their identification (or if they forget
to note their uncertainty), identificationQualifier will be NA
. Let's run the
same code as above, but this time specifying that we want only those observations
that are unambiguous identifications.
# filter on `scientificName` is Peromyscus maniculatus and `sex` is female.
# two equals signs (==) signifies "is"
data_PeroManicFemalesCertain <- filter(myData,
scientificName == 'Peromyscus maniculatus',
sex == 'F',
identificationQualifier =="NA")
# Count the number of un-ambiguous identifications
nrow(data_PeroManicFemalesCertain)
## [1] 0
Woah! So every single observation of a Peromyscus maniculatus had some level
of uncertainty that the individual may actually be Peromyscus leucopus. This
is understandable given the difficulty of field identification for these species.
Given this challenge, it will be best for us to consider these mice at the genus
level. We can accomplish this by searching for only the genus name in the
'scientificName' field using the grepl()
function.
grepl()
This is a function in the base package (e.g., it isn't part of dplyr
) that is
part of the suite of Regular Expressions functions. grepl
uses regular
expressions to match patterns in character strings. Regular expressions offer
very powerful and useful tricks for data manipulation. They can be complicated
and therefore are a challenge to learn, but well worth it!
Here, we present a very simple example.
- inputs: pattern to match, character vector to search for a match
- output: a logical vector indicating whether the pattern was found within each element of the input character vector
Let's use grepl
to learn more about our possible disease vectors. In reality,
all species of Peromyscus are viable players in Lyme disease transmission, and
they are difficult to distinguise in a field setting, so we really should be
looking at all species of Peromyscus. Since we don't have genera split out as
a separate field, we have to search within the scientificName
string for the
genus -- this is a simple example of pattern matching.
We can use the dplyr
function filter()
in combination with the base function
grepl()
to accomplish this.
# combine filter & grepl to get all Peromyscus (a part of the
# scientificName string)
data_PeroFemales <- filter(myData,
grepl('Peromyscus', scientificName),
sex == 'F')
# how many female Peromyscus are in the dataset
print(paste('In 2014, NEON technicians captured',
nrow(data_PeroFemales),
'female Peromyscus spp. at Harvard Forest.',
sep = ' '))
## [1] "In 2014, NEON technicians captured 558 female Peromyscus spp. at Harvard Forest."
group_by() + summarise()
An alternative to using the filter function to subset the data (and make a new
data object in the process), is to calculate summary statistics based on some
grouping factor. We'll use group_by()
, which does basically the same thing as
SQL or other tools for interacting with relational databases. For those
unfamiliar with SQL, no worries - dplyr
provides lots of additional
functionality for working with databases (local and remote) that does not
require knowledge of SQL. How handy!
The group_by()
function in dplyr
allows you to perform functions on a subset
of a dataset without having to create multiple new objects or construct for()
loops. The combination of group_by()
and summarise()
are great for
generating simple summaries (counts, sums) of grouped data.
NOTE: Be continentious about using summarise
with other summary functions! You
need to think about weighting for means and variances, and summarize
doesn't
work precisely for medians if there is any missing data (e.g., if there was no
value recorded, maybe it was for a good reason!).
Continuing with our small mammal data, since the diversity of the entire small
mammal community has been shown to impact disease dynamics among the key
reservoir species, we really want to know more about the demographics of the
whole community. We can quickly generate counts by species and sex in 2 lines of
code, using group_by
and summarise
.
# how many of each species & sex were there?
# step 1: group by species & sex
dataBySpSex <- group_by(myData, scientificName, sex)
# step 2: summarize the number of individuals of each using the new df
countsBySpSex <- summarise(dataBySpSex, n_individuals = n())
## `summarise()` regrouping output by 'scientificName' (override with `.groups` argument)
# view the data (just top 10 rows)
head(countsBySpSex, 10)
## # A tibble: 10 x 3
## # Groups: scientificName [5]
## scientificName sex n_individuals
## <chr> <chr> <int>
## 1 Blarina brevicauda F 50
## 2 Blarina brevicauda M 8
## 3 Blarina brevicauda U 22
## 4 Blarina brevicauda <NA> 19
## 5 Glaucomys volans M 1
## 6 Mammalia sp. U 1
## 7 Mammalia sp. <NA> 1
## 8 Microtus pennsylvanicus F 2
## 9 Myodes gapperi F 103
## 10 Myodes gapperi M 99
Note: the output of step 1 (dataBySpSex
) does not look any different than the
original dataframe (myData
), but the application of subsequent functions (e.g.,
summarise) to this new dataframe will produce distinctly different results than
if you tried the same operations on the original. Try it if you want to see the
difference! This is because the group_by()
function converted dataBySpSex
into a "grouped_df" rather than just a "data.frame". In order to confirm this,
try using the class()
function on both myData
and dataBySpSex
. You can
also read the help documentation for this function by running the code:
?group_by()
# View class of 'myData' object
class(myData)
## [1] "data.frame"
# View class of 'dataBySpSex' object
class(dataBySpSex)
## [1] "grouped_df" "tbl_df" "tbl" "data.frame"
# View help file for group_by() function
?group_by()
Pipe functions together
We created multiple new data objects during our explorations of dplyr
functions, above. While this works, we can produce the same results more
efficiently by chaining functions together and creating only one new data object
that encapsulates all of the previously sought information: filter
on only
females, grepl
to get only Peromyscus spp., group_by
individual species, and
summarise
the numbers of individuals.
# combine several functions to get a summary of the numbers of individuals of
# female Peromyscus species in our dataset.
# remember %>% are "pipes" that allow us to pass information from one function
# to the next.
dataBySpFem <- myData %>%
filter(grepl('Peromyscus', scientificName), sex == "F") %>%
group_by(scientificName) %>%
summarise(n_individuals = n())
## `summarise()` ungrouping output (override with `.groups` argument)
# view the data
dataBySpFem
## # A tibble: 3 x 2
## scientificName n_individuals
## <chr> <int>
## 1 Peromyscus leucopus 455
## 2 Peromyscus maniculatus 98
## 3 Peromyscus sp. 5
Cool!
Base R only
So that is nice, but we had to install a new package dplyr
. You might ask,
"Is it really worth it to learn new commands if I can do this is base R." While
we think "yes", why don't you see for yourself. Here is the base R code needed
to accomplish the same task.
# For reference, the same output but using R's base functions
# First, subset the data to only female Peromyscus
dataFemPero <- myData[myData$sex == 'F' &
grepl('Peromyscus', myData$scientificName), ]
# Option 1 --------------------------------
# Use aggregate and then rename columns
dataBySpFem_agg <-aggregate(dataFemPero$sex ~ dataFemPero$scientificName,
data = dataFemPero, FUN = length)
names(dataBySpFem_agg) <- c('scientificName', 'n_individuals')
# view output
dataBySpFem_agg
## scientificName n_individuals
## 1 Peromyscus leucopus 455
## 2 Peromyscus maniculatus 98
## 3 Peromyscus sp. 5
# Option 2 --------------------------------------------------------
# Do it by hand
# Get the unique scientificNames in the subset
sppInDF <- unique(dataFemPero$scientificName[!is.na(dataFemPero$scientificName)])
# Use a loop to calculate the numbers of individuals of each species
sciName <- vector(); numInd <- vector()
for (i in sppInDF) {
sciName <- c(sciName, i)
numInd <- c(numInd, length(which(dataFemPero$scientificName==i)))
}
#Create the desired output data frame
dataBySpFem_byHand <- data.frame('scientificName'=sciName,
'n_individuals'=numInd)
# view output
dataBySpFem_byHand
## scientificName n_individuals
## 1 Peromyscus leucopus 455
## 2 Peromyscus maniculatus 98
## 3 Peromyscus sp. 5
Hierarchical Data Formats - What is HDF5?
Learning Objectives
After completing this tutorial, you will be able to:
- Explain what the Hierarchical Data Format (HDF5) is.
- Describe the key benefits of the HDF5 format, particularly related to big data.
- Describe both the types of data that can be stored in HDF5 and how it can be stored/structured.
About Hierarchical Data Formats - HDF5
The Hierarchical Data Format version 5 (HDF5), is an open source file format that supports large, complex, heterogeneous data. HDF5 uses a "file directory" like structure that allows you to organize data within the file in many different structured ways, as you might do with files on your computer. The HDF5 format also allows for embedding of metadata making it self-describing.
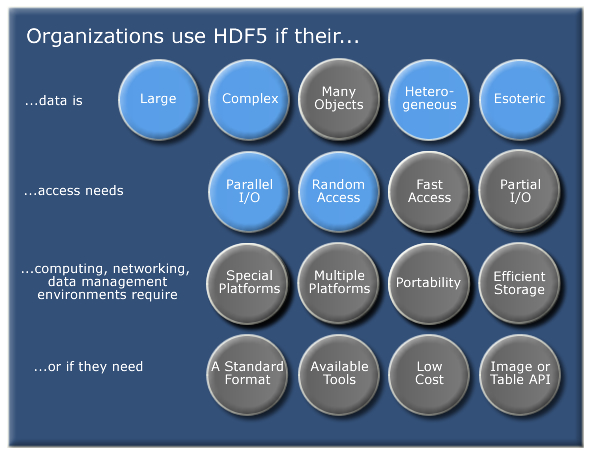
Hierarchical Structure - A file directory within a file
The HDF5 format can be thought of as a file system contained and described
within one single file. Think about the files and folders stored on your computer.
You might have a data directory with some temperature data for multiple field
sites. These temperature data are collected every minute and summarized on an
hourly, daily and weekly basis. Within one HDF5 file, you can store a similar
set of data organized in the same way that you might organize files and folders
on your computer. However in a HDF5 file, what we call "directories" or "folders"
on our computers, are called groups
and what we call files on our
computer are called datasets
.
2 Important HDF5 Terms
- Group: A folder like element within an HDF5 file that might contain other groups OR datasets within it.
- Dataset: The actual data contained within the HDF5 file. Datasets are often (but don't have to be) stored within groups in the file.
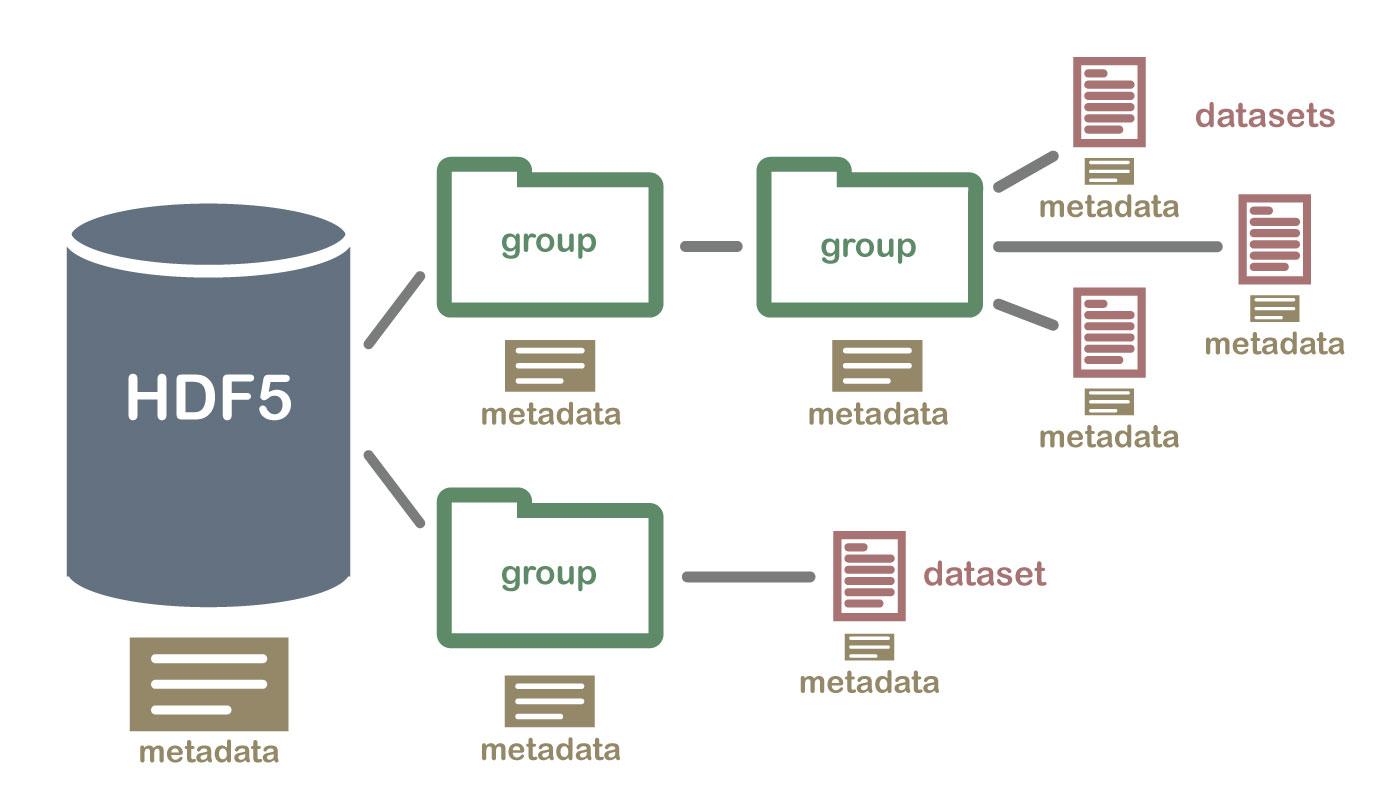
An HDF5 file containing datasets, might be structured like this:
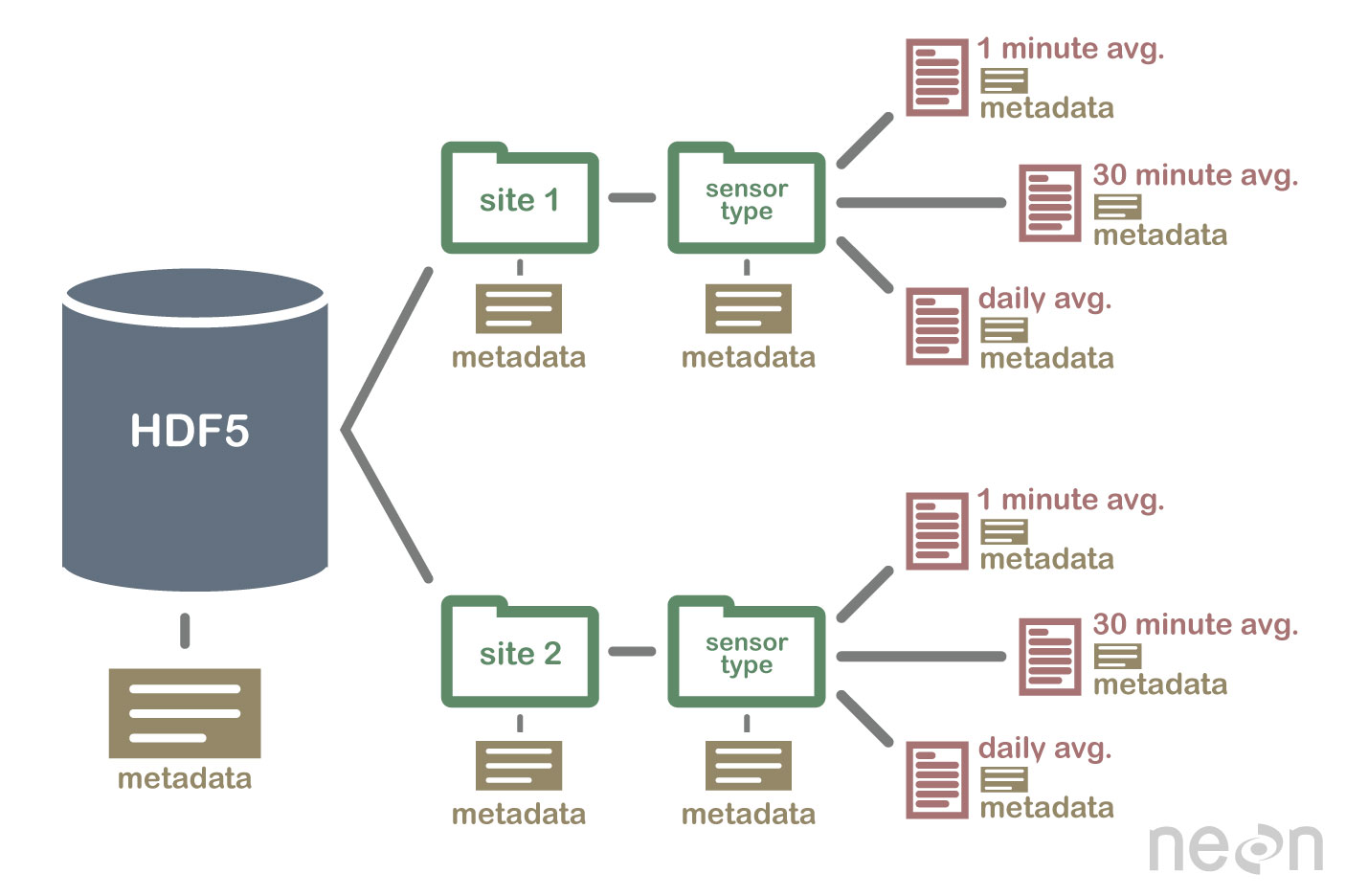
HDF5 is a Self Describing Format
HDF5 format is self describing. This means that each file, group and dataset can have associated metadata that describes exactly what the data are. Following the example above, we can embed information about each site to the file, such as:
- The full name and X,Y location of the site
- Description of the site.
- Any documentation of interest.
Similarly, we might add information about how the data in the dataset were collected, such as descriptions of the sensor used to collect the temperature data. We can also attach information, to each dataset within the site group, about how the averaging was performed and over what time period data are available.
One key benefit of having metadata that are attached to each file, group and
dataset, is that this facilitates automation without the need for a separate
(and additional) metadata document. Using a programming language, like R or
Python
, we can grab information from the metadata that are already associated
with the dataset, and which we might need to process the dataset.
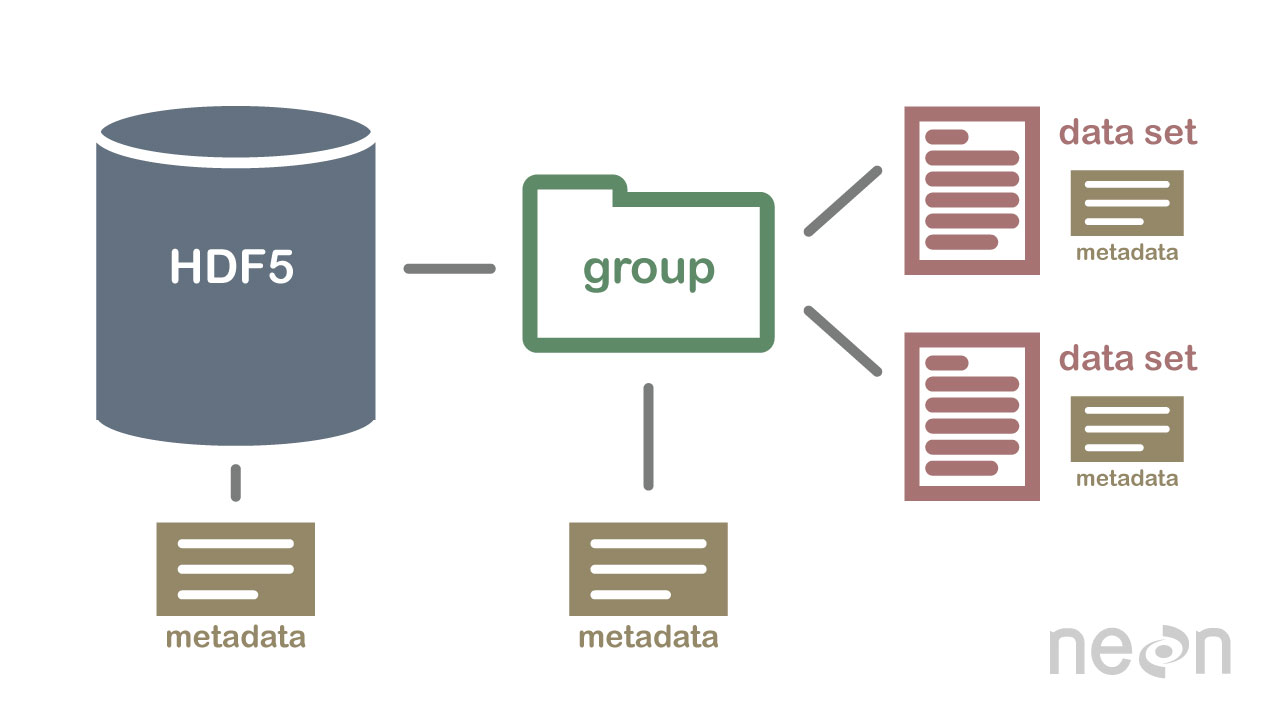
Compressed & Efficient subsetting
The HDF5 format is a compressed format. The size of all data contained within
HDF5 is optimized which makes the overall file size smaller. Even when
compressed, however, HDF5 files often contain big data and can thus still be
quite large. A powerful attribute of HDF5 is data slicing
, by which a
particular subsets of a dataset can be extracted for processing. This means that
the entire dataset doesn't have to be read into memory (RAM); very helpful in
allowing us to more efficiently work with very large (gigabytes or more) datasets!
Heterogeneous Data Storage
HDF5 files can store many different types of data within in the same file. For example, one group may contain a set of datasets to contain integer (numeric) and text (string) data. Or, one dataset can contain heterogeneous data types (e.g., both text and numeric data in one dataset). This means that HDF5 can store any of the following (and more) in one file:
- Temperature, precipitation and PAR (photosynthetic active radiation) data for a site or for many sites
- A set of images that cover one or more areas (each image can have specific spatial information associated with it - all in the same file)
- A multi or hyperspectral spatial dataset that contains hundreds of bands.
- Field data for several sites characterizing insects, mammals, vegetation and meteorology.
- A set of images that cover one or more areas (each image can have unique spatial information associated with it)
- And much more!
Open Format
The HDF5 format is open and free to use. The supporting libraries (and a free
viewer), can be downloaded from the
HDF Group
website. As such, HDF5 is widely supported in a host of programs, including
open source programming languages like R and Python
, and commercial
programming tools like Matlab
and IDL
. Spatial data that are stored in HDF5
format can be used in GIS and imaging programs including QGIS
, ArcGIS
, and
ENVI
.
Summary Points - Benefits of HDF5
- Self-Describing The datasets with an HDF5 file are self describing. This allows us to efficiently extract metadata without needing an additional metadata document.
- Supporta Heterogeneous Data: Different types of datasets can be contained within one HDF5 file.
- Supports Large, Complex Data: HDF5 is a compressed format that is designed to support large, heterogeneous, and complex datasets.
- Supports Data Slicing: "Data slicing", or extracting portions of the dataset as needed for analysis, means large files don't need to be completely read into the computers memory or RAM.
-
Open Format - wide support in the many tools: Because the HDF5 format is
open, it is supported by a host of programming languages and tools, including
open source languages like R and
Python
and open GIS tools likeQGIS
.
HDFView: Exploring HDF5 Files in the Free HDFview Tool
In this tutorial you will use the free HDFView tool to explore HDF5 files and the groups and datasets contained within. You will also see how HDF5 files can be structured and explore metadata using both spatial and temporal data stored in HDF5!
Learning Objectives
After completing this activity, you will be able to:
- Explain how data can be structured and stored in HDF5.
- Navigate to metadata in an HDF5 file, making it "self describing".
- Explore HDF5 files using the free HDFView application.
Tools You Will Need
Install the free HDFView application. This application allows you to explore the contents of an HDF5 file easily. Click here to go to the download page.
Data to Download
NOTE: The first file downloaded has an .HDF5 file extension, the second file downloaded below has an .h5 extension. Both extensions represent the HDF5 data type.
NEON Teaching Data Subset: Sample Tower Temperature - HDF5
These temperature data were collected by the National Ecological Observatory Network's flux towers at field sites across the US. The entire dataset can be accessed by request from the NEON Data Portal.
Download DatasetDownload NEON Teaching Data Subset: Imaging Spectrometer Data - HDF5
These hyperspectral remote sensing data provide information on the National Ecological Observatory Network's San Joaquin Exerimental Range field site. The data were collected over the San Joaquin field site located in California (Domain 17) and processed at NEON headquarters. The entire dataset can be accessed by request from the NEON Data Portal.
Download DatasetInstalling HDFView
Select the HDFView download option that matches the operating system (Mac OS X, Windows, or Linux) and computer setup (32 bit vs 64 bit) that you have.
This tutorial was written with graphics from the VS 2012 version, but it is applicable to other versions as well.
Hierarchical Data Format 5 - HDF5
Hierarchical Data Format version 5 (HDF5), is an open file format that supports large, complex, heterogeneous data. Some key points about HDF5:
- HDF5 uses a "file directory" like structure.
- The HDF5 data models organizes information using
Groups
. Each group may contain one or moredatasets
. - HDF5 is a self describing file format. This means that the metadata for the data contained within the HDF5 file, are built into the file itself.
- One HDF5 file may contain several heterogeneous data types (e.g. images, numeric data, data stored as strings).
For more introduction to the HDF5 format, see our About Hierarchical Data Formats - What is HDF5? tutorial.
In this tutorial, we will explore two different types of data saved in HDF5. This will allow us to better understand how one file can store multiple different types of data, in different ways.
Part 1: Exploring Temperature Data in HDF5 Format in HDFView
The first thing that we will do is open an HDF5 file in the viewer to get a better idea of how HDF5 files can be structured.
Open a HDF5/H5 file in HDFView
To begin, open the HDFView application.
Within the HDFView application, select File --> Open and navigate to the folder
where you saved the NEONDSTowerTemperatureData.hdf5
file on your computer. Open this file in HDFView.
If you click on the name of the HDF5 file in the left hand window of HDFView, you can view metadata for the file. This will be located in the bottom window of the application.
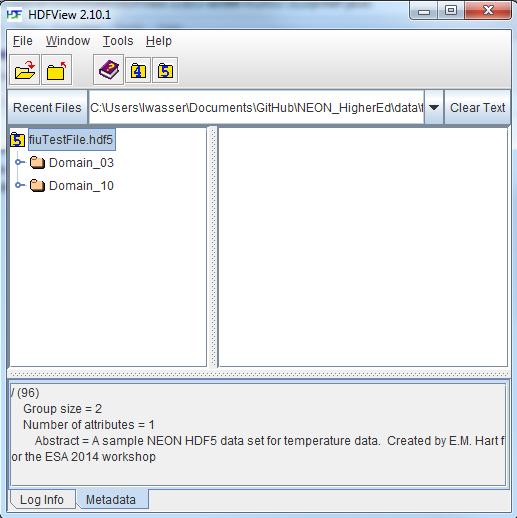
Explore File Structure in HDFView
Next, explore the structure of this file. Notice that there are two Groups (represented as folder icons in the viewer) called "Domain_03" and "Domain_10". Within each domain group, there are site groups (NEON sites that are located within those domains). Expand these folders by double clicking on the folder icons. Double clicking expands the groups content just as you might expand a folder in Windows explorer.
Notice that there is metadata associated with each group.
Double click on the OSBS
group located within the Domain_03 group. Notice in
the metadata window that OSBS
contains data collected from the
NEON Ordway-Swisher Biological Station field site.
Within the OSBS
group there are two more groups - Min_1 and Min_30. What data
are contained within these groups?
Expand the "min_1" group within the OSBS site in Domain_03. Notice that there are five more nested groups named "Boom_1, 2, etc". A boom refers to an arm on a tower, which sits at a particular height and to which are attached sensors for collecting data on such variables as temperature, wind speed, precipitation, etc. In this case, we are working with data collected using temperature sensors, mounted on the tower booms.
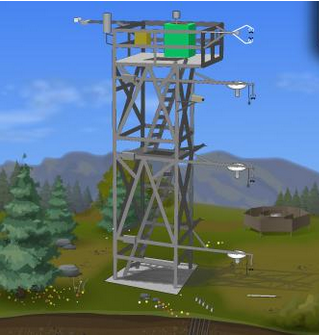
Speaking of temperature - what type of sensor is collected the data within the boom_1 folder at the Ordway Swisher site? HINT: check the metadata for that dataset.
Expand the "Boom_1" folder by double clicking it. Finally, we have arrived at a dataset! Have a look at the metadata associated with the temperature dataset within the boom_1 group. Notice that there is metadata describing each attribute in the temperature dataset. Double click on the group name to open up the table in a tabular format. Notice that these data are temporal.
So this is one example of how an HDF5 file could be structured. This particular file contains data from multiple sites, collected from different sensors (mounted on different booms on the tower) and collected over time. Take some time to explore this HDF5 dataset within the HDFViewer.
Part 2: Exploring Hyperspectral Imagery stored in HDF5
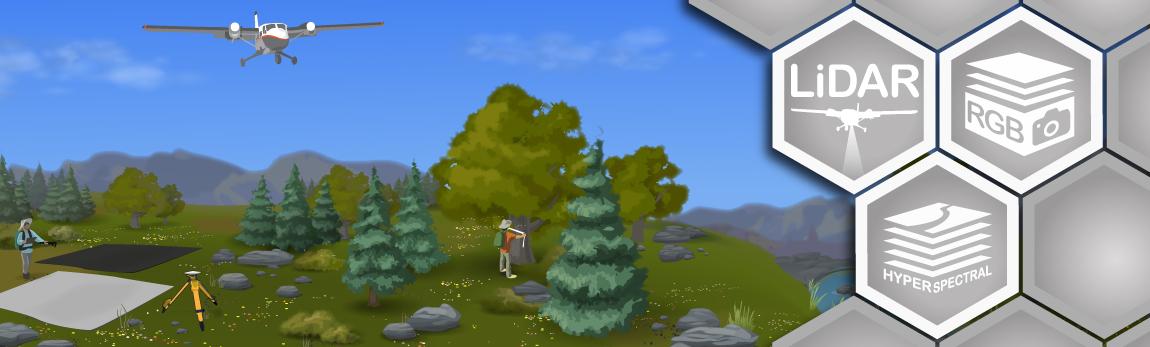
Next, we will explore a hyperspectral dataset, collected by the NEON Airborne Observation Platform (AOP) and saved in HDF5 format. Hyperspectral data are naturally hierarchical, as each pixel in the dataset contains reflectance values for hundreds of bands collected by the sensor. The NEON sensor (imaging spectrometer) collected data within 428 bands.
A few notes about hyperspectral imagery:
- An imaging spectrometer, which collects hyperspectral imagery, records light energy reflected off objects on the earth's surface.
- The data are inherently spatial. Each "pixel" in the image is located spatially and represents an area of ground on the earth.
- Similar to an Red, Green, Blue (RGB) camera, an imaging spectrometer records reflected light energy. Each pixel will contain several hundred bands worth of reflectance data.
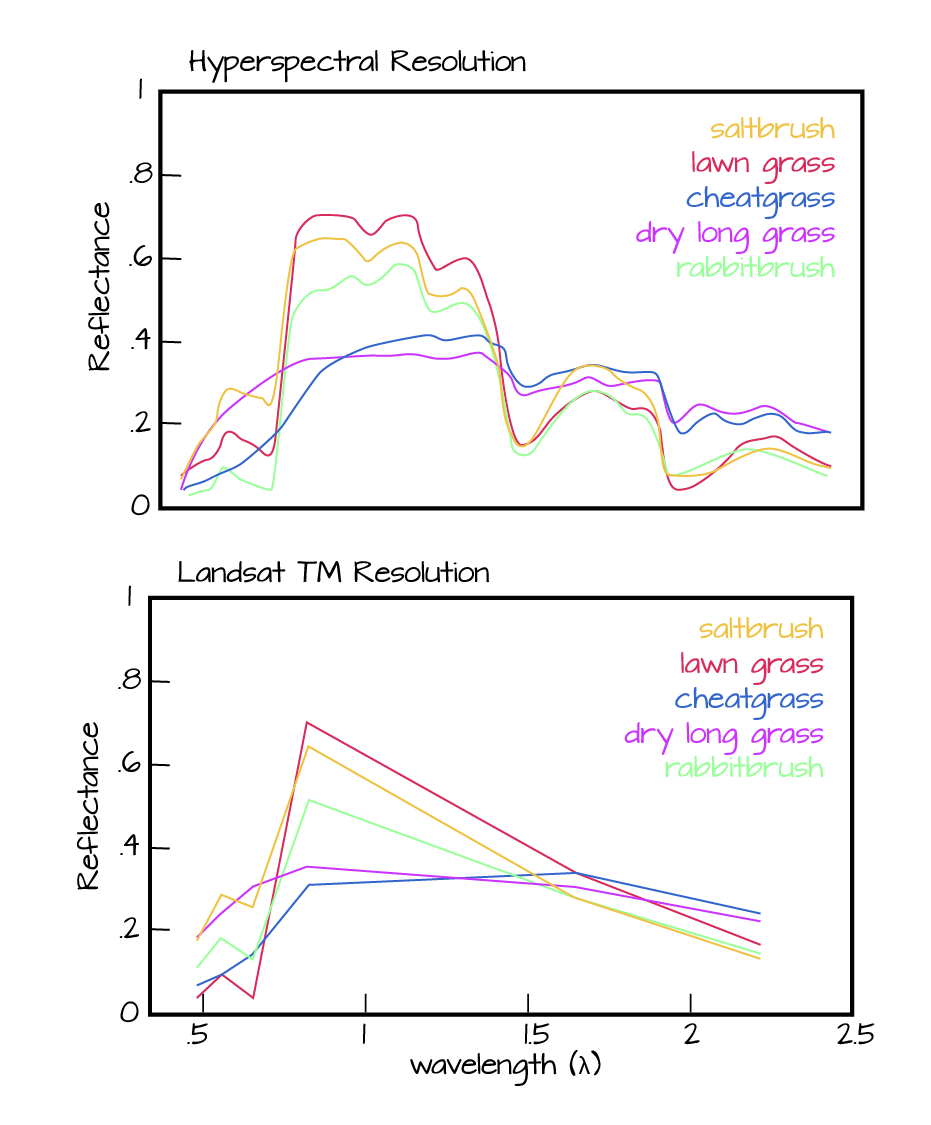
Read more about hyperspectral remote sensing data:
Let's open some hyperspectral imagery stored in HDF5 format to see what the file structure can like for a different type of data.
Open the file. Notice that it is structured differently. This file is composed of 3 datasets:
- Reflectance,
- fwhm, and
- wavelength.
It also contains some text information called "map info". Finally it contains a group called spatial info.
Let's first look at the metadata stored in the spatialinfo group. This group contains all of the spatial information that a GIS program would need to project the data spatially.
Next, double click on the wavelength dataset. Note that this dataset contains the central wavelength value for each band in the dataset.
Finally, click on the reflectance dataset. Note that in the metadata for the dataset that the structure of the dataset is 426 x 501 x 477 (wavelength, line, sample), as indicated in the metadata. Right click on the reflectance dataset and select Open As. Click Image in the "display as" settings on the left hand side of the popup.
In this case, the image data are in the second and third dimensions of this dataset. However, HDFView will default to selecting the first and second dimensions
Let’s tell the HDFViewer to use the second and third dimensions to view the image:
- Under
height
, make suredim 1
is selected. - Under
width
, make suredim 2
is selected.
Notice an image preview appears on the left of the pop-up window. Click OK to open the image. You may have to play with the brightness and contrast settings in the viewer to see the data properly.
Explore the spectral dataset in the HDFViewer taking note of the metadata and data stored within the file.
Introduction to HDF5 Files in R
Learning Objectives
After completing this tutorial, you will be able to:
- Understand how HDF5 files can be created and structured in R using the rhdf5 libraries.
- Understand the three key HDF5 elements: the HDF5 file itself, groups, and datasets.
- Understand how to add and read attributes from an HDF5 file.
Things You’ll Need To Complete This Tutorial
To complete this tutorial you will need the most current version of R and, preferably, RStudio loaded on your computer.
R Libraries to Install:
- rhdf5: The rhdf5 package is hosted on Bioconductor not CRAN. Directions for installation are in the first code chunk.
More on Packages in R – Adapted from Software Carpentry.
Data to Download
We will use the file below in the optional challenge activity at the end of this tutorial.
NEON Teaching Data Subset: Field Site Spatial Data
These remote sensing data files provide information on the vegetation at the National Ecological Observatory Network's San Joaquin Experimental Range and Soaproot Saddle field sites. The entire dataset can be accessed by request from the NEON Data Portal.
Download DatasetSet Working Directory: This lesson assumes that you have set your working directory to the location of the downloaded and unzipped data subsets.
An overview of setting the working directory in R can be found here.
R Script & Challenge Code: NEON data lessons often contain challenges that reinforce learned skills. If available, the code for challenge solutions is found in the downloadable R script of the entire lesson, available in the footer of each lesson page.
Additional Resources
Consider reviewing the documentation for the RHDF5 package.
About HDF5
The HDF5 file can store large, heterogeneous datasets that include metadata. It
also supports efficient data slicing
, or extraction of particular subsets of a
dataset which means that you don't have to read large files read into the
computers memory / RAM in their entirety in order work with them.
HDF5 in R
To access HDF5 files in R, we will use the rhdf5
library which is part of
the Bioconductor
suite of R libraries. It might also be useful to install
the
free HDF5 viewer
which will allow you to explore the contents of an HDF5 file using a graphic interface.
More about working with HDFview and a hands-on activity here.
First, let's get R setup. We will use the rhdf5 library. To access HDF5 files in R, we will use the rhdf5 library which is part of the Bioconductor suite of R packages. As of May 2020 this package was not yet on CRAN.
# Install rhdf5 package (only need to run if not already installed)
#install.packages("BiocManager")
#BiocManager::install("rhdf5")
# Call the R HDF5 Library
library("rhdf5")
# set working directory to ensure R can find the file we wish to import and where
# we want to save our files
wd <- "~/Git/data/" #This will depend on your local environment
setwd(wd)
Read more about the
rhdf5
package here.
Create an HDF5 File in R
Now, let's create a basic H5 file with one group and one dataset in it.
# Create hdf5 file
h5createFile("vegData.h5")
## [1] TRUE
# create a group called aNEONSite within the H5 file
h5createGroup("vegData.h5", "aNEONSite")
## [1] TRUE
# view the structure of the h5 we've created
h5ls("vegData.h5")
## group name otype dclass dim
## 0 / aNEONSite H5I_GROUP
Next, let's create some dummy data to add to our H5 file.
# create some sample, numeric data
a <- rnorm(n=40, m=1, sd=1)
someData <- matrix(a,nrow=20,ncol=2)
Write the sample data to the H5 file.
# add some sample data to the H5 file located in the aNEONSite group
# we'll call the dataset "temperature"
h5write(someData, file = "vegData.h5", name="aNEONSite/temperature")
# let's check out the H5 structure again
h5ls("vegData.h5")
## group name otype dclass dim
## 0 / aNEONSite H5I_GROUP
## 1 /aNEONSite temperature H5I_DATASET FLOAT 20 x 2
View a "dump" of the entire HDF5 file. NOTE: use this command with CAUTION if you are working with larger datasets!
# we can look at everything too
# but be cautious using this command!
h5dump("vegData.h5")
## $aNEONSite
## $aNEONSite$temperature
## [,1] [,2]
## [1,] 0.33155432 2.4054446
## [2,] 1.14305151 1.3329978
## [3,] -0.57253964 0.5915846
## [4,] 2.82950139 0.4669748
## [5,] 0.47549005 1.5871517
## [6,] -0.04144519 1.9470377
## [7,] 0.63300177 1.9532294
## [8,] -0.08666231 0.6942748
## [9,] -0.90739256 3.7809783
## [10,] 1.84223101 1.3364965
## [11,] 2.04727590 1.8736805
## [12,] 0.33825921 3.4941913
## [13,] 1.80738042 0.5766373
## [14,] 1.26130759 2.2307994
## [15,] 0.52882731 1.6021497
## [16,] 1.59861449 0.8514808
## [17,] 1.42037674 1.0989390
## [18,] -0.65366487 2.5783750
## [19,] 1.74865593 1.6069304
## [20,] -0.38986048 -1.9471878
# Close the file. This is good practice.
H5close()
Add Metadata (attributes)
Let's add some metadata (called attributes in HDF5 land) to our dummy temperature data. First, open up the file.
# open the file, create a class
fid <- H5Fopen("vegData.h5")
# open up the dataset to add attributes to, as a class
did <- H5Dopen(fid, "aNEONSite/temperature")
# Provide the NAME and the ATTR (what the attribute says) for the attribute.
h5writeAttribute(did, attr="Here is a description of the data",
name="Description")
h5writeAttribute(did, attr="Meters",
name="Units")
Now we can add some attributes to the file.
# let's add some attributes to the group
did2 <- H5Gopen(fid, "aNEONSite/")
h5writeAttribute(did2, attr="San Joaquin Experimental Range",
name="SiteName")
h5writeAttribute(did2, attr="Southern California",
name="Location")
# close the files, groups and the dataset when you're done writing to them!
H5Dclose(did)
H5Gclose(did2)
H5Fclose(fid)
Working with an HDF5 File in R
Now that we've created our H5 file, let's use it! First, let's have a look at the attributes of the dataset and group in the file.
# look at the attributes of the precip_data dataset
h5readAttributes(file = "vegData.h5",
name = "aNEONSite/temperature")
## $Description
## [1] "Here is a description of the data"
##
## $Units
## [1] "Meters"
# look at the attributes of the aNEONsite group
h5readAttributes(file = "vegData.h5",
name = "aNEONSite")
## $Location
## [1] "Southern California"
##
## $SiteName
## [1] "San Joaquin Experimental Range"
# let's grab some data from the H5 file
testSubset <- h5read(file = "vegData.h5",
name = "aNEONSite/temperature")
testSubset2 <- h5read(file = "vegData.h5",
name = "aNEONSite/temperature",
index=list(NULL,1))
H5close()
Once we've extracted data from our H5 file, we can work with it in R.
# create a quick plot of the data
hist(testSubset2)
Time to practice the skills you've learned. Open up the D17_2013_SJER_vegStr.csv in R.
- Create a new HDF5 file called
vegStructure
. - Add a group in your HDF5 file called
SJER
. - Add the veg structure data to that folder.
- Add some attributes the SJER group and to the data.
- Now, repeat the above with the D17_2013_SOAP_vegStr csv.
- Name your second group SOAP
Hint: read.csv()
is a good way to read in .csv files.